Multi-Label Image Classification Model Based on Multiscale Fusion and Adaptive Label Correlation
Journal of Shanghai Jiaotong University (Science)(2024)
摘要
At present, research on multi-label image classification mainly focuses on exploring the correlation between labels to improve the classification accuracy of multi-label images. However, in existing methods, label correlation is calculated based on the statistical information of the data. This label correlation is global and depends on the dataset, not suitable for all samples. In the process of extracting image features, the characteristic information of small objects in the image is easily lost, resulting in a low classification accuracy of small objects. To this end, this paper proposes a multi-label image classification model based on multiscale fusion and adaptive label correlation. The main idea is: first, the feature maps of multiple scales are fused to enhance the feature information of small objects. Semantic guidance decomposes the fusion feature map into feature vectors of each category, then adaptively mines the correlation between categories in the image through the self-attention mechanism of graph attention network, and obtains feature vectors containing category-related information for the final classification. The mean average precision of the model on the two public datasets of VOC 2007 and MS COCO 2014 reached 95.6% and 83.6%, respectively, and most of the indicators are better than those of the existing latest methods.
更多查看译文
关键词
image classification,label correlation,graph attention network,small object,multi-scale fusion,图像分类,标签相关性,图注意力网络,小目标,多尺度融合,TP391.41,A
AI 理解论文
溯源树
样例
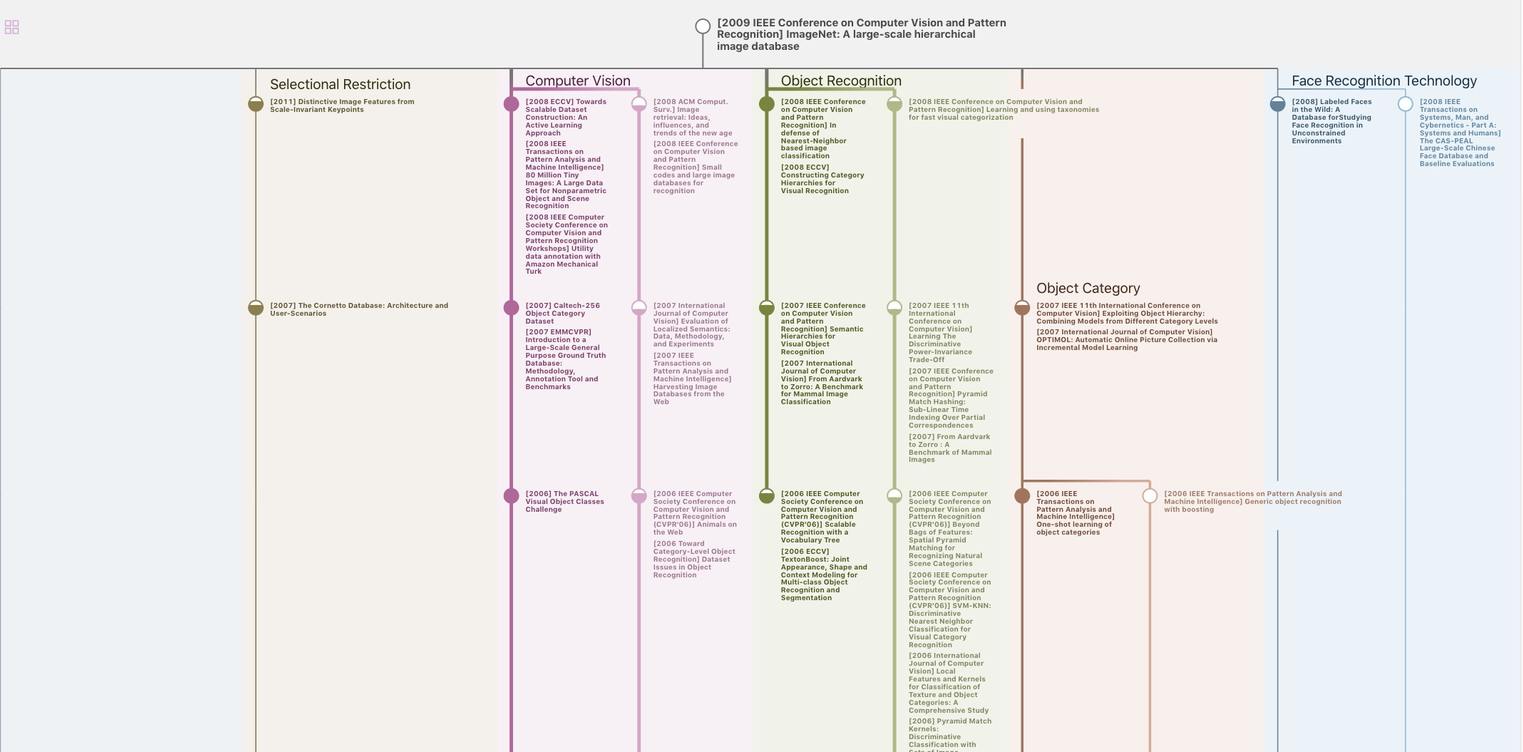
生成溯源树,研究论文发展脉络
Chat Paper
正在生成论文摘要