A Neuroevolutionary Approach for System Identification
Journal of Control, Automation and Electrical Systems(2023)
摘要
Through System Identification techniques, it is possible to obtain a mathematical model for a dynamic system from its input/output data. Due to their intrinsic dynamic behavior and simple and fast training procedure, the use of echo state networks (ESNs), a kind of neural network, for System Identification is advantageous. However, ESNs have global parameters that should be tuned in order to improve their performance in a determined task. Besides, a random reservoir may not be ideal in terms of performance. Due to their theoretical ability to obtain good solutions with few evaluations, the Real Coded Quantum-Inspired Evolutionary Algorithm (QIEA-R) and the Covariance Matrix Adaptation Evolution Strategy (CMA-ES) represent efficient alternatives of evolutionary algorithms for optimizing ESN global parameters and weights. Thus, this work proposes a neuro-evolutionary method that automatically defines an ESN for System Identification problems. The method initially focuses on finding the best ESN global parameters by using the QIEA-R or the CMA-ES then, in sequence, selecting its best reservoir, which can be done by a second optimization focused on some reservoir weights or by doing a simple choice based on networks with random reservoirs. The method was applied to seven benchmark problems in System Identification produced good results when compared to traditional methods.
更多查看译文
关键词
Echo state networks,Evolutionary algorithms,System identification,Quantum-inspired evolutionary algorithms
AI 理解论文
溯源树
样例
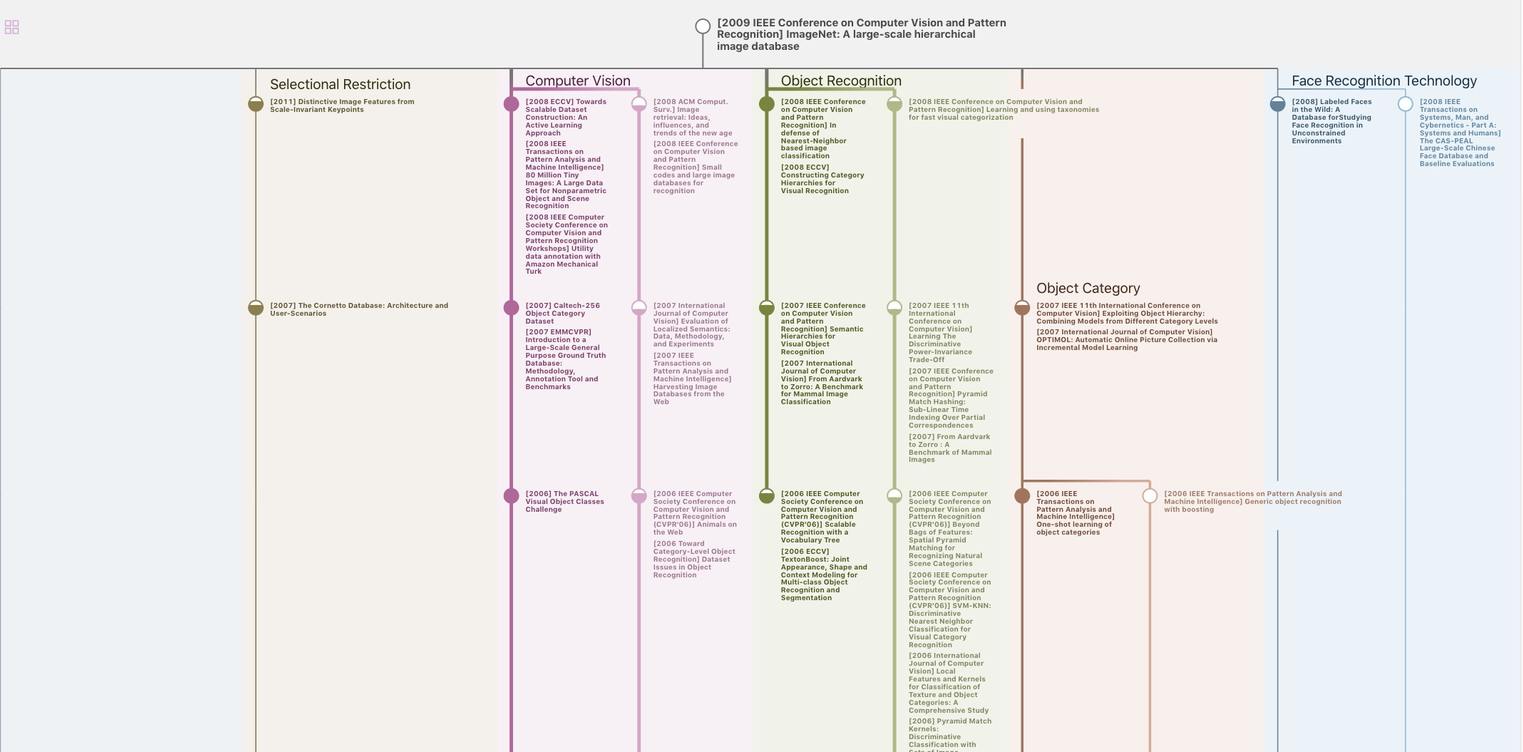
生成溯源树,研究论文发展脉络
Chat Paper
正在生成论文摘要