CRNet: Channel-Enhanced Remodeling-Based Network for Salient Object Detection in Optical Remote Sensing Images.
IEEE transactions on geoscience and remote sensing(2023)
摘要
Despite the remarkable progress made by the salient object detection of natural sensing images (NSI-SOD), the complex background and scale diversity issues of remote sensing images (RSIs) still pose a substantial obstacle. In this study, we build an end-to-end channel-enhanced remodeling-based network (CRNet) for optical RSIs (ORSIs) to highlight salient objects through feature augmentation. First, the backbone convolutional block is used to suggest the fundamental characteristics. Then, we use the channel enhance module (CEM) to enhance the shallow features. CEM primarily relies on the channel attention (CA) mechanism and uses a no-downscaling strategy to produce local cross-channel interaction, which lowers model complexity while enhancing extraction performance. Meanwhile, we use the redefined feature module (RFM) to reconstruct the deep features and generate global attention features by dimensional transformation and feature relationship aggregation to achieve the role of locating salient targets. Finally, the cascade combines the multiscale features to provide the final saliency map. To further enhance the representational power of the network, we use a hybrid loss function to improve performance. The proposed approach outperforms current state-of-the-art (SOTA) methods, as shown by several experiments on three available datasets. The source code of the proposed CRNet is available publicly at https://github.com/hilitteq/CRNet.git .
更多查看译文
关键词
Channel enhance module (CEM),optical remote sensing images (RSIS),redefined feature module (RFM),salient object detection (SOD)
AI 理解论文
溯源树
样例
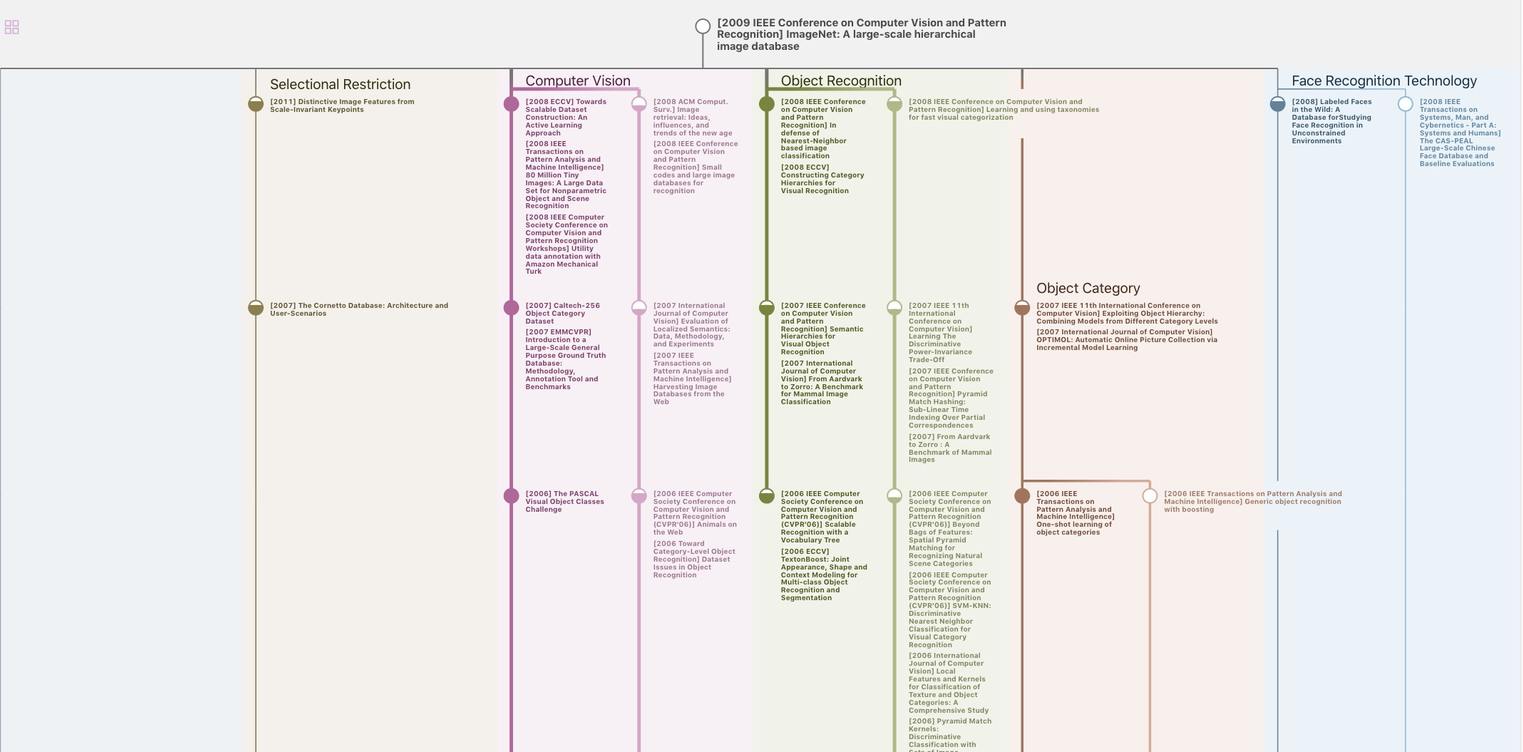
生成溯源树,研究论文发展脉络
Chat Paper
正在生成论文摘要