Dense depth distillation with out-of-distribution simulated images
KNOWLEDGE-BASED SYSTEMS(2024)
摘要
We study data-free knowledge distillation (KD) for monocular depth estimation (MDE), which learns a lightweight model for real-world depth perception tasks by compressing it from a trained teacher model while lacking training data in the target domain. Owing to the essential difference between image classification and dense regression, previous methods of data-free KD are not applicable to MDE. To strengthen its applicability in real-world tasks, in this paper, we propose to apply KD with out-of-distribution simulated images. The major challenges to be resolved are (i) lacking prior information about scene configurations of real-world training data and (ii) domain shift between simulated and real-world images. To cope with these difficulties, we propose a tailored framework for depth distillation. The framework generates new training samples for embracing a multitude of possible object arrangements in the target domain and utilizes a transformation network to efficiently adapt them to the feature statistics preserved in the teacher model. Through extensive experiments on various depth estimation models and two different datasets, we show that our method outperforms the baseline KD by a good margin and even achieves slightly better performance with as few as 1/6 of training images, demonstrating a clear superiority.
更多查看译文
关键词
Monocular depth estimation,Knowledge distillation,Data-free KD,Dense distillation
AI 理解论文
溯源树
样例
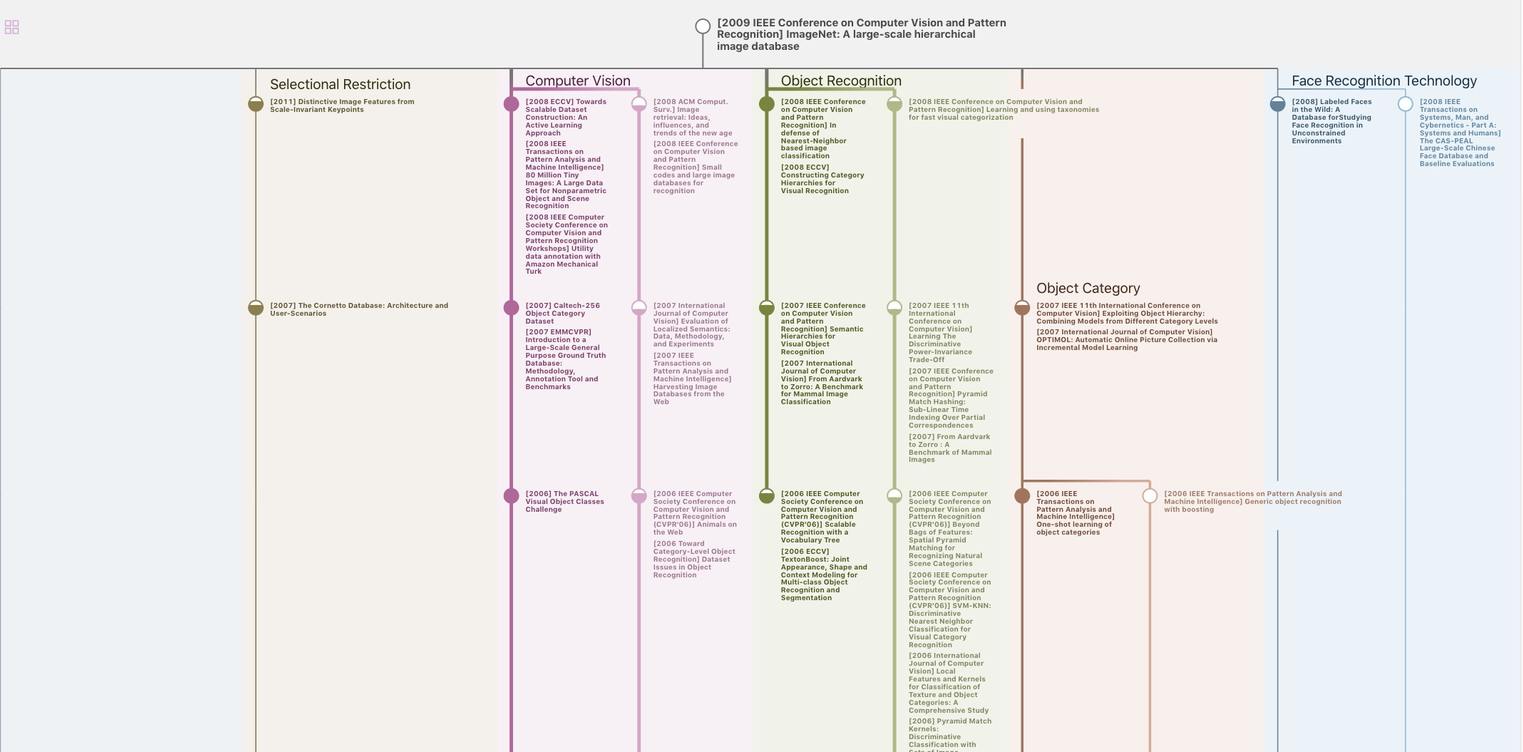
生成溯源树,研究论文发展脉络
Chat Paper
正在生成论文摘要