End-to-end 3D CNN for plot-scale soybean yield prediction using multitemporal UAV-based RGB images
Precision Agriculture(2023)
Abstract
Crop yield prediction from UAV images has significant potential in accelerating and revolutionizing crop breeding pipelines. Although convolutional neural networks (CNN) provide easy, accurate and efficient solutions over traditional machine learning models in computer vision applications, a CNN training requires large number of ground truth data, which is often difficult to collect in the agricultural context. The major objective of this study was to develope an end-to-end 3D CNN model for plot-scale soybean yield prediction using multitemporal UAV-based RGB images with approximately 30,000 sample plots. A low-cost UAV-RGB system was utilized and multitemporal images from 13 different experimental fields were collected at Argentina in 2021. Three commonly used 2D CNN architectures (i.e., VGG, ResNet and DenseNet) were transformed into 3D variants to incorporate the temporal data as the third dimension. Additionally, multiple spatiotemporal resolutions were considered as data input and the CNN architectures were trained with different combinations of input shapes. The results reveal that: (a) DenseNet provided the most efficient result (R2 0.69) in terms of accuracy and model complexity, followed by VGG (R2 0.70) and ResNet (R2 0.65); (b) Finer spatiotemporal resolution did not necessarily improve the model performance but increased the model complexity, while the coarser resolution achieved comparable results; and (c) DenseNet showed lower clustering patterns in its prediction maps compared to the other models. This study clearly identifies that multitemporal observation with UAV-based RGB images provides enough information for the 3D CNN architectures to accurately estimate soybean yield non-destructively and efficiently.
MoreTranslated text
Key words
Deep learning,Artificial intelligence,Plant phenotyping,Smart agriculture,AWS,TensorFlow
AI Read Science
Must-Reading Tree
Example
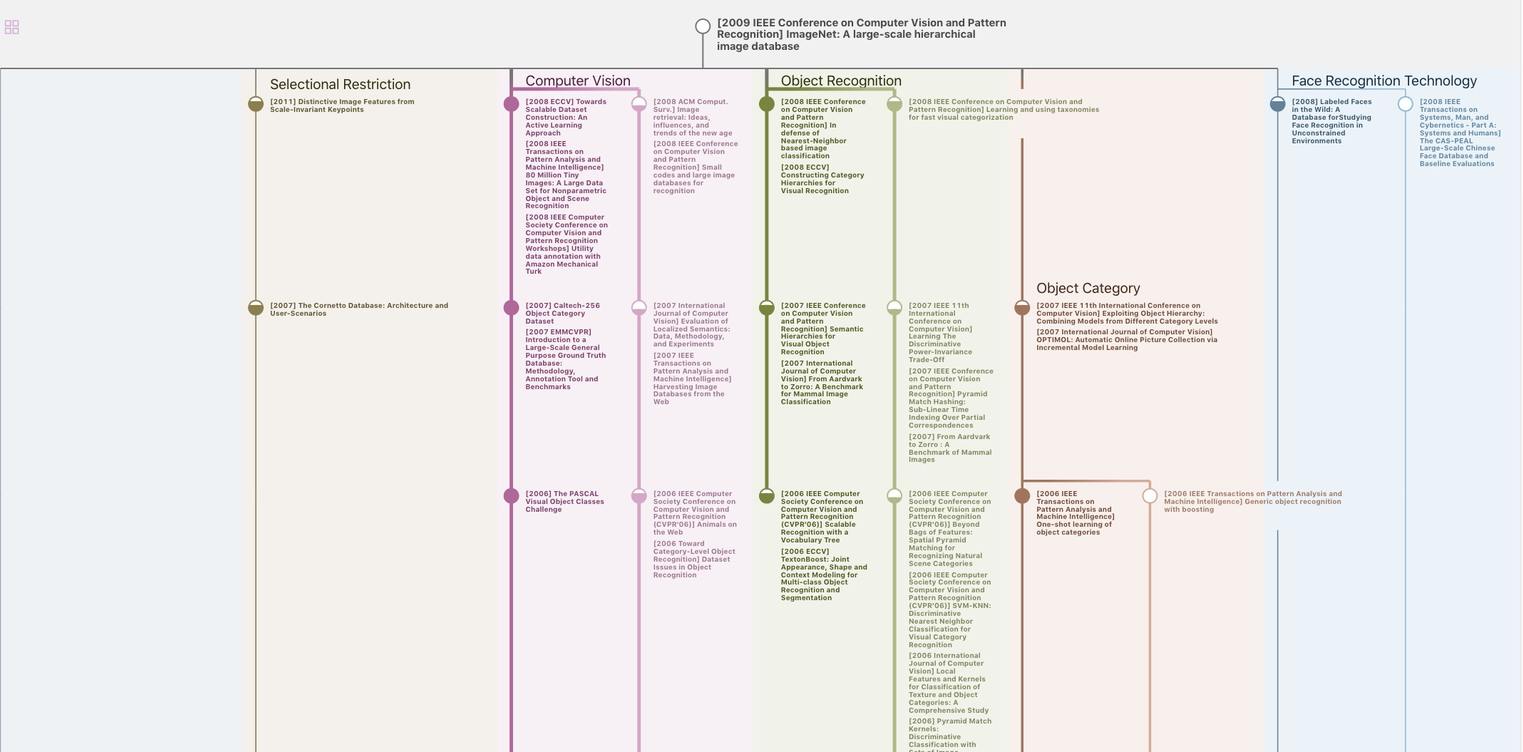
Generate MRT to find the research sequence of this paper
Chat Paper
Summary is being generated by the instructions you defined