Machine Learning Prediction of Electric Flux in Concrete and Mix Proportion Optimization Design
Materials today communications(2024)
摘要
This study explored nine machine learning (ML) methods, including linear, nonlinear and integrated learning models, to predict concrete electrical flux metrics reflecting the chloride ion permeability of concrete. Seven concrete mix parameters, including water (W), cement (C), fly ash (FA), ground granulated blast furnace slag (GGBS), fine aggregate (S), coarse aggregate (G) and water reducing admixture (WRA), were used as input parameters. A dataset consisting of 4029 sets of data was used to train and test the prediction model. K-fold cross-validation confirms the model’s accuracy and is performed by R2, MAE, MSE, and RMSE. The results showed that the nonlinear model outperformed the linear model; in particular, the model integrated with learning CatBoost exhibited higher prediction accuracy (R2 = 0.72, RMSE = 0.01, MSE = 0.01, MAE = 0.04). Based on this, a concrete chloride impermeability mix proportion optimization method targeting cost per square is proposed. The proposed model aims to achieve an ideal concrete mix proportion optimization based on ML algorithms and national specifications, which is of great significance to practical engineering.
更多查看译文
关键词
Machine learning,CatBoost algorithm,Electrical flux,Impermeability,Mix proportion optimization
AI 理解论文
溯源树
样例
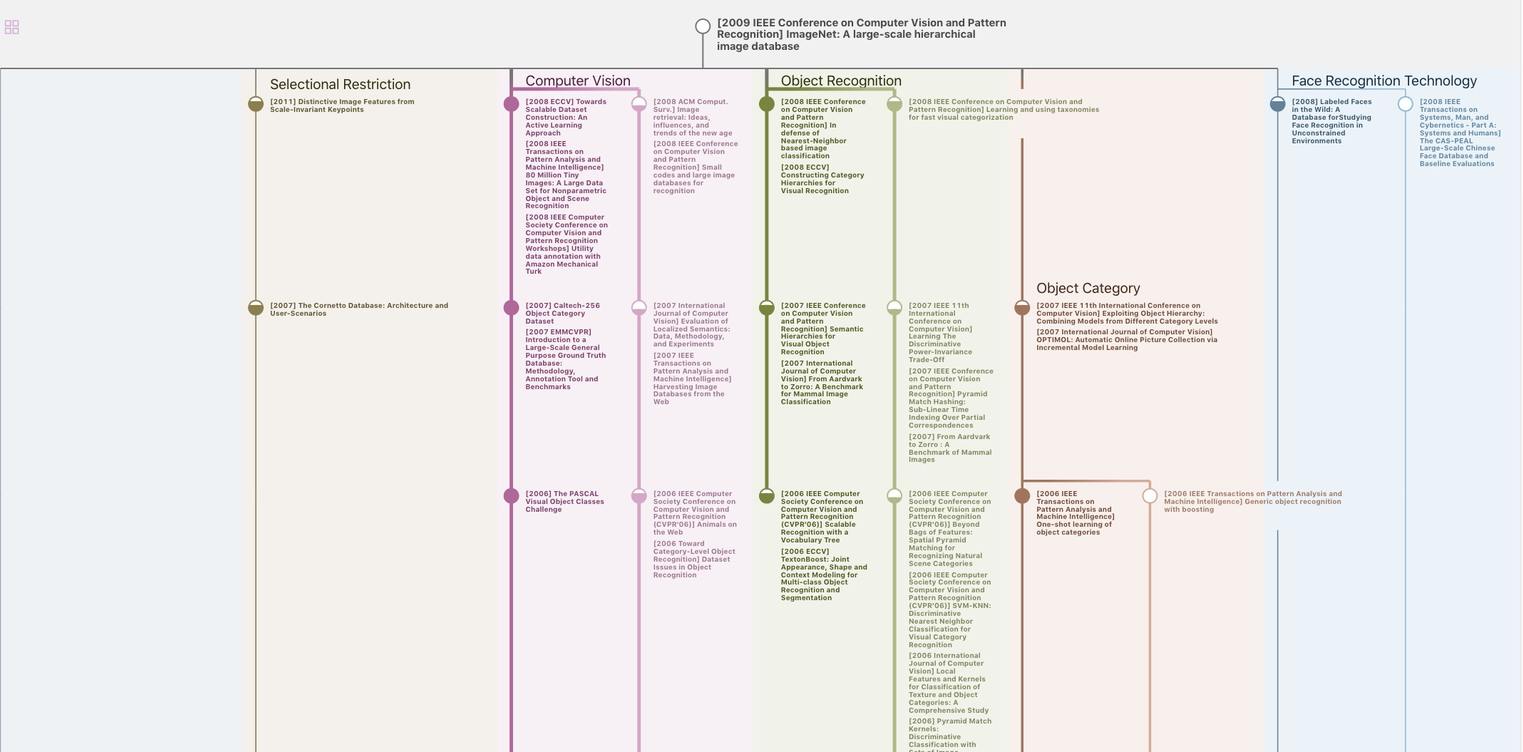
生成溯源树,研究论文发展脉络
Chat Paper
正在生成论文摘要