Accelerated design of low-activation high entropy alloys with desired phase and property by machine learning
APPLIED MATERIALS TODAY(2024)
摘要
Low-activation high-entropy alloys (HEAs) have been regarded as novel candidate structural materials for fusion reactors due to their excellent mechanical and radiation resistant properties. Nevertheless, the potential vast composition space brings a prominent challenge in the design of low-activation HEAs. Herein, a new strategy based on machine learning (ML) was proposed to accelerate the exploitation of low-activation HEAs with desired phase and property. Two optimized classification models with accuracy of > 85% were developed to identify single-phase body-centered-cubic (BCC) solid-solution (SS) HEAs. One regression model with correlation coefficient (R) of > 0.9 was constructed to predict the hardness of HEAs. The phase and hardness prediction models were combined in accordance with an integrated design strategy to identify the low-activation HEAs with desired phase and property from 284,634 candidates. With this design strategy, a new desired single-phase BCC Fe35Cr30V20Mn10Ti5 low-activation HEA with 555.9 +/- 15.3 HV was designed and fabricated via only two experimental iterations. Besides, two new phase selection rules with accuracy of > 95% were given to efficiently screen SS and BCC HEAs, respectively. Our research work not only provides new ideas to search for low-activation HEAs, but also can be extended to the integrated design of structure and performance of other advanced materials.
更多查看译文
关键词
Machine learning,Feature screening,High entropy alloys,Phase classification,Hardness prediction
AI 理解论文
溯源树
样例
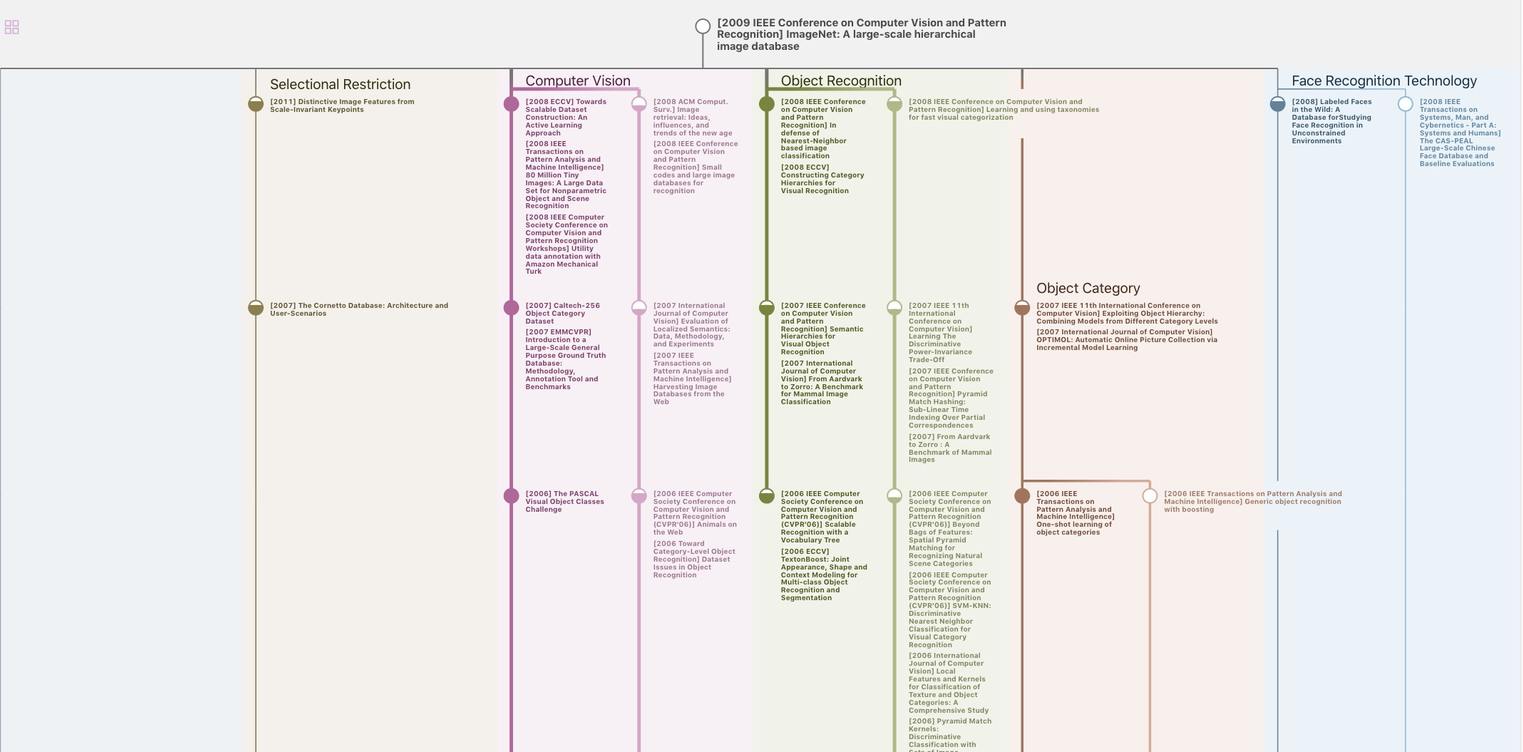
生成溯源树,研究论文发展脉络
Chat Paper
正在生成论文摘要