Digital twin modeling method of the temperature field of thermo-compression bonding blade based on generative adversarial networks
ADVANCES IN ENGINEERING SOFTWARE(2024)
Abstract
In this paper, a novel method of using deep learning to construct a digital twin of the thermos-compression bonding blade`s temperature field is presented. Usually, a heat transfer model based on numerical analysis can calculate the heat distribution in the welding process, but its complex modeling process and heavy computational effort are not appropriate for the deployment of the digital twin concept. The approach proposed in this paper is distinctive because it directly converts the collected process sensor data into the bonding blade's temperature field images and the bonding surface's temperature distribution curves for monitoring the welding process. This is achieved by training the generator and discriminator of the conditional depth convolutional generative adversarial network separately. The network is then capable of predicting the bonding blade's heat distribution within a certain range of welding parameters. Compared to methods based on numerical simulation, this approach is far more effective. Training and testing datasets were created to assess the suggested method's efficacy. Multi-scale structural similarity index, structural similarity index, peak signal-to-noise ratio, difference hash and mean absolute error were used to evaluate the simulations' quality. The results show that the simulation's results and the actual data agree quite well. The temperature changes that occur during the welding process may be quickly and accurately simulated using the proposed technology.
MoreTranslated text
Key words
Digital twin,GAN,SSIM,Thermo-compression bonding,Temperature field prediction
AI Read Science
Must-Reading Tree
Example
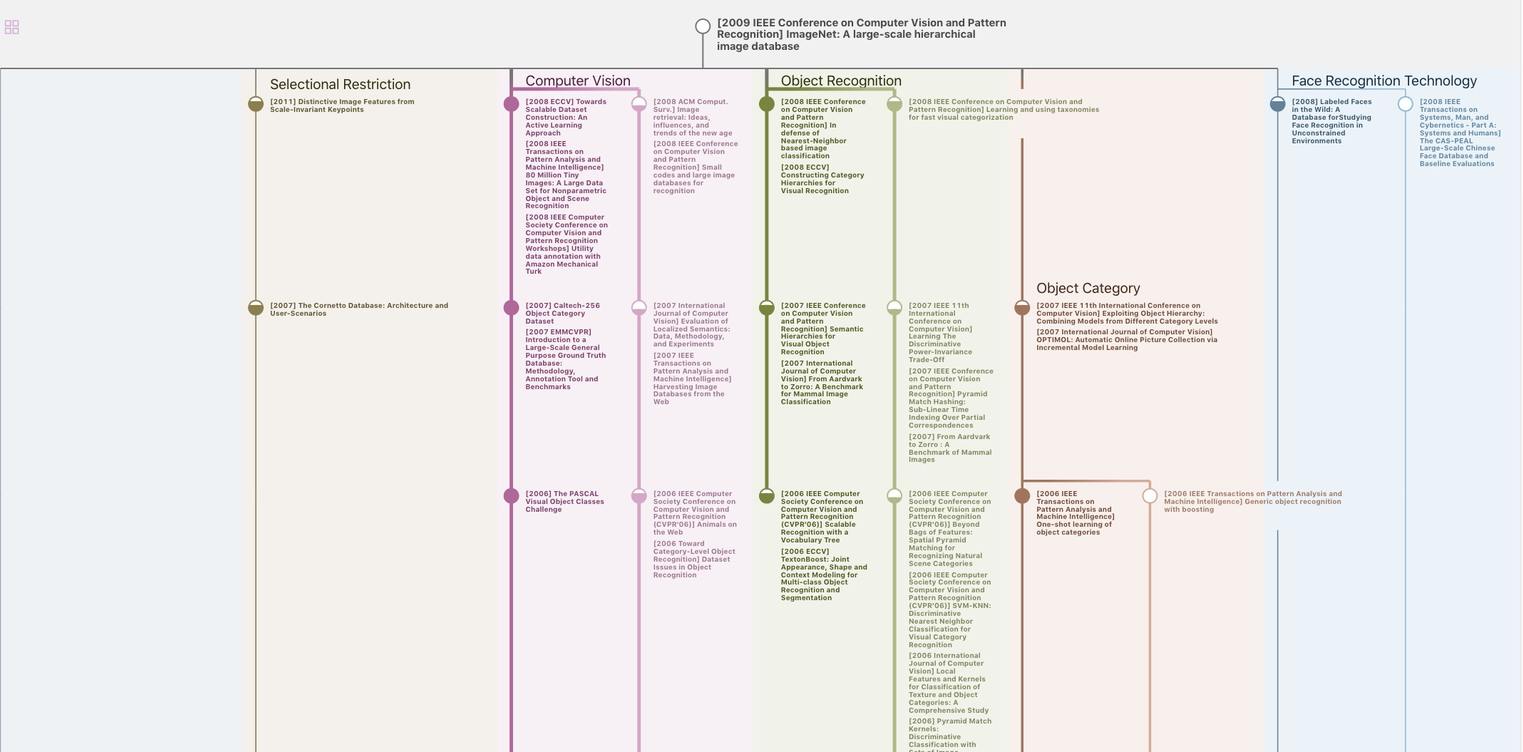
Generate MRT to find the research sequence of this paper
Chat Paper
Summary is being generated by the instructions you defined