Instance-level Loss Based Multiple-Instance Learning Framework for Acoustic Scene Classification
APPLIED ACOUSTICS(2024)
Abstract
An acoustic scene is inferred by detecting properties combining diverse sounds and acoustic environments. This study is intended to discover these properties effectively using multiple-instance learning (MIL). MIL, also known as a weakly supervised learning approach, is a strategy for extracting an instance vector from an audio chunk that composes an audio clip and utilizing these unlabeled instances to infer a scene corresponding to the input data. However, many studies pointed out an underestimation problem of MIL. In this study, we propose an enhanced MIL framework more suitable for ASC systems by defining instance-level labels and loss to extract and cluster instances effectively. Furthermore, we design a lightweight convolutional neural network named FUSE comprising frequency-, temporal-sided depthwise, and pointwise convolutional filters. Experimental results show that the confidence and proportion of positive instances significantly increase compared to vanilla MIL, overcoming the underestimation problem and improving the classification accuracy even higher than the supervised learning. The proposed system achieved a performance of 81.1%, 72.3%, and 58.3% on the TAU urban acoustic scenes 2019, 2020 mobile, and 2022 mobile datasets with 139 K parameters, respectively. In particular, it achieves the highest performance among the systems having under the 1 M parameters for the TAU urban acoustic scenes 2019 dataset.
MoreTranslated text
Key words
Acoustic scene classification,Multiple-instance learning,Weakly supervised learning
AI Read Science
Must-Reading Tree
Example
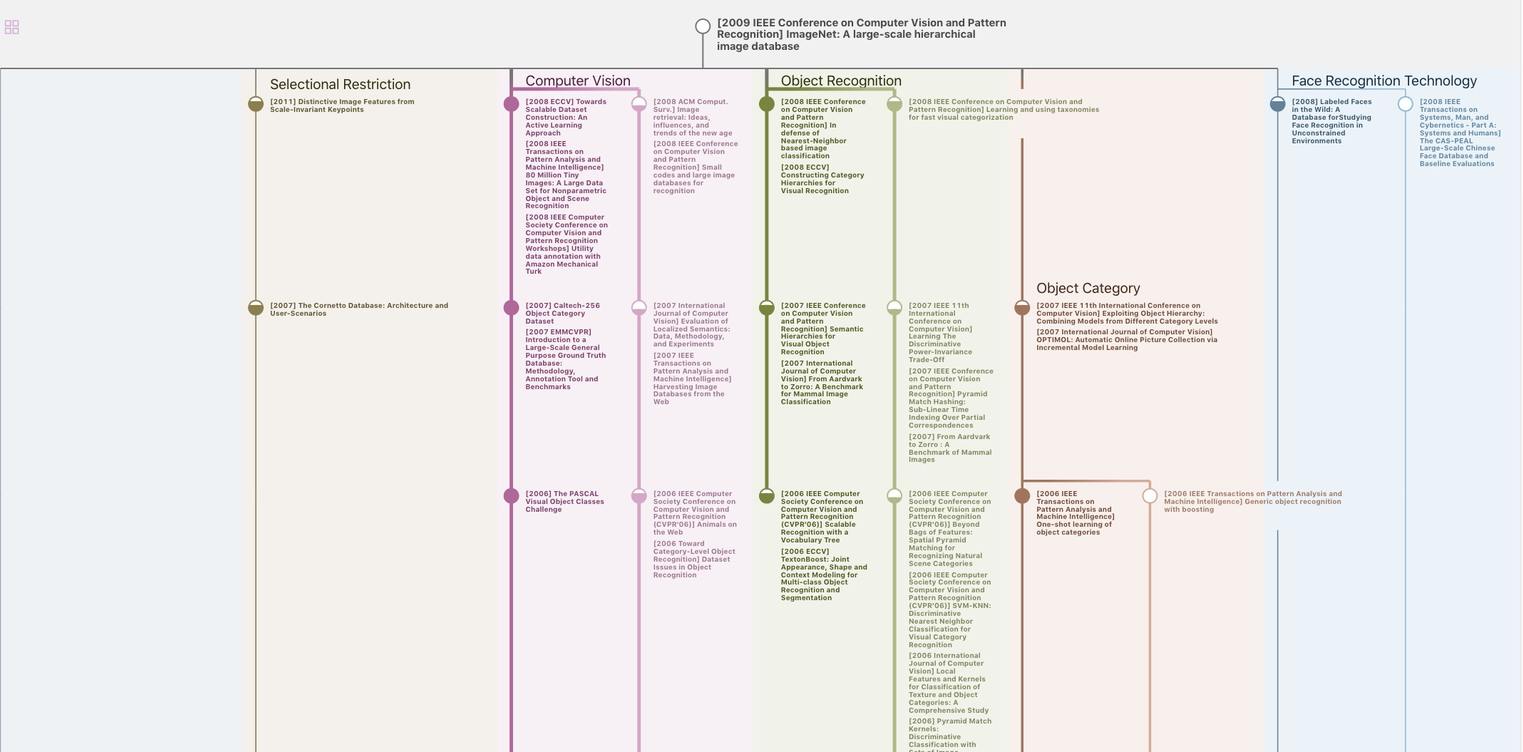
Generate MRT to find the research sequence of this paper
Chat Paper
Summary is being generated by the instructions you defined