SPTR: Structure-Preserving Transformer for Unsupervised Indoor Depth Completion
IEEE TRANSACTIONS ON CIRCUITS AND SYSTEMS FOR VIDEO TECHNOLOGY(2024)
摘要
Recovering a dense depth map from a pair of indoor RGB and sparse depth images in an unsupervised manner is paramount in applications such as autonomous driving and 3D reconstruction. Most existing methods leverage sparse depth maps to directly estimate the dense depth map with the pixel-wise regression constraints over the input known depth. However, such regression constraints independently compare per-pixel depth values, which ignore the important 3D structures hidden behind depth maps and result in severe structural distortion and poor robustness. In this paper, we propose a Structure-Preserving Encoding (SPE) module by reformulating depth completion as the process of 3D structure generation. The generated structure should recover the complete scene and also consist with the known partial structure, so that the learned depth features from this task are able to encode rich structural information. In addition, SPE hierarchically interpolates and propagates the 3D structures into dense structure-aware positional encodings, which further boosts the information interactions between RGB and depth features via our transformer. Extensive experiments on VOID and NYUv2 demonstrate that SPTR outperforms the state-of-the-art methods by a large margin across various densities of input depths and a strong generalization ability to other datasets.
更多查看译文
关键词
Depth completion,RGB-D fusion,structure learning
AI 理解论文
溯源树
样例
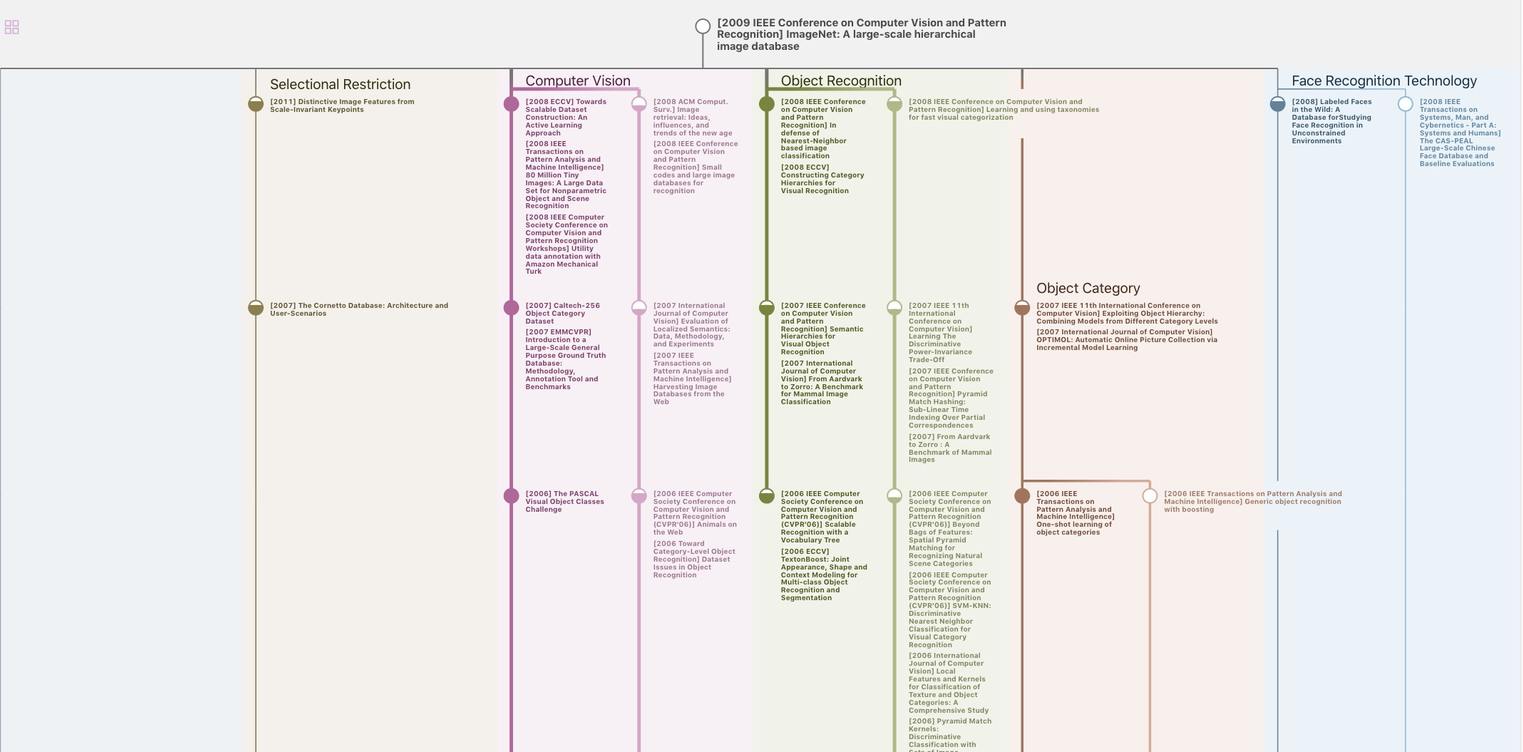
生成溯源树,研究论文发展脉络
Chat Paper
正在生成论文摘要