Influence of Structure and Texture Feature on Retrieval of Ramie Leaf Area Index
AGRONOMY-BASEL(2023)
摘要
Leaf area index (LAI), as one of the important crop field phenotypes, plays a crucial role in evaluating crop growth and yield. The remote sensing method for crop parameters monitoring makes it possible to obtain crop LAI in a large area, efficiently and non-destructively. Fusion of spectral features, structure features, and texture features obtained by remote sensing images can effectively improve LAI estimation performance, but the impact of crop structure and texture feature differences on LAI remote sensing monitoring remains unclear. In this study, ramie was classified according to the differences of canopy structure, and then the LAI inversion performance of ramie was evaluated under different datasets to determine the contribution of structure features (canopy coverage, plant height) and texture features derived from remote sensing images to LAI estimation. Finally, multi-feature remote sensing indexes were integrated to construct the optimal ramie LAI estimation model. The results showed that structure features and texture features had an effect on ramie LAI inversion performance, and there were significant differences in LAI estimation accuracy among different data sets. A single structure feature or texture feature could not significantly optimize LAI inversion performance, while multi-feature fusion could effectively improve LAI estimation accuracy (R-2 = 0.776, RMSE = 0.740). This study provides a robust, practical, and low-cost method for ramie LAI estimation, and provides a reference for crop LAI inversion research based on structural features and texture features.
更多查看译文
关键词
leaf area index,ramie,structural feature,texture feature,unmanned aerial vehicle
AI 理解论文
溯源树
样例
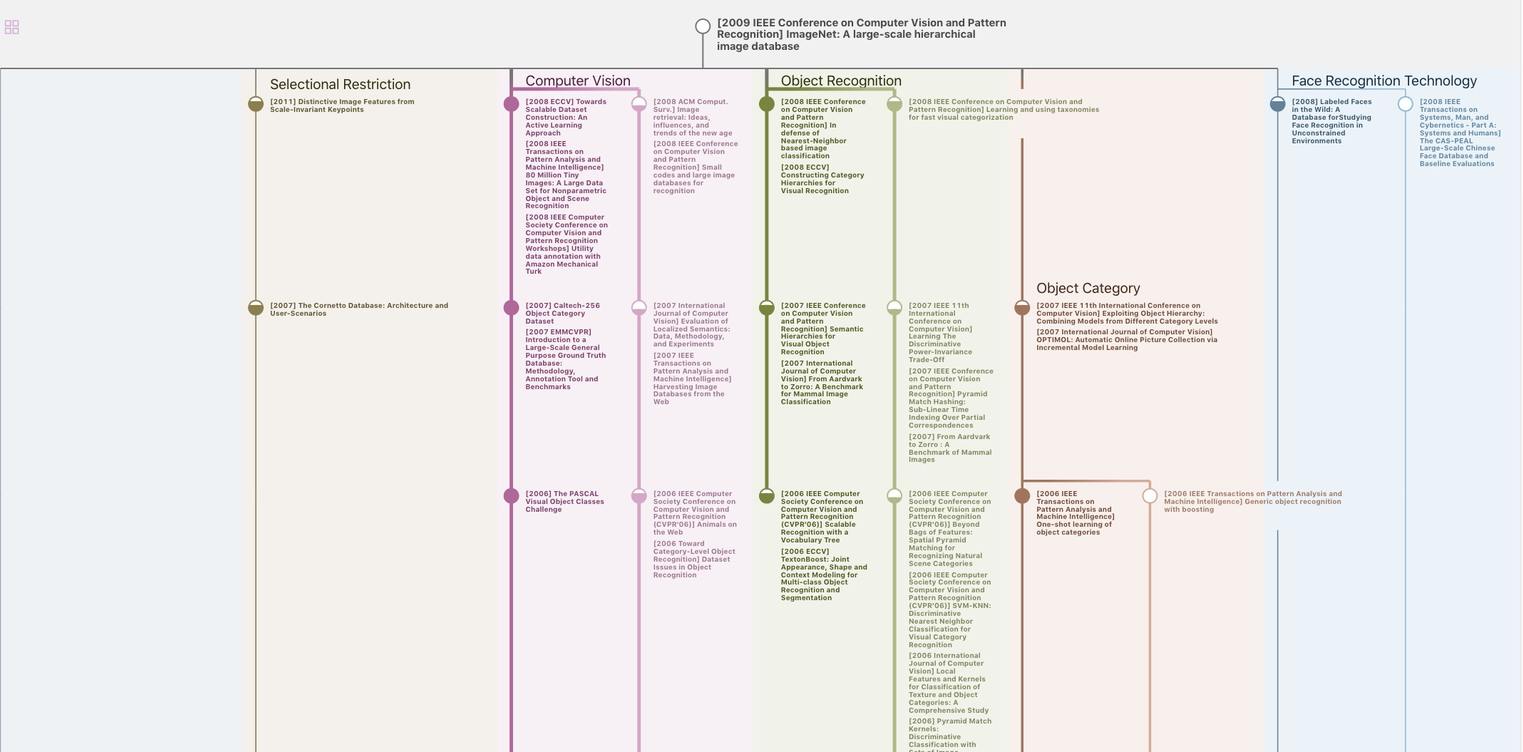
生成溯源树,研究论文发展脉络
Chat Paper
正在生成论文摘要