Investigation on Feature Attribution for Remaining Useful Life Prediction Model of Cryogenic Ball Bearing
Mechanisms and machine science(2023)
摘要
This paper investigates the feature attribution in remaining useful life (RUL) prediction model of cryogenic ball bearing. The RUL prediction model is constructed based on artificial neural network (ANN) by using the TensorFlow platform for training the degradation curve of bearing. To train the models, 5 run-to-failure (RTF) data of cryogenic ball bearings were used. The experiment was driven to 3,600rpm with 20kN axial load and 2.5kN radial load for accelerated life test (ALT) of bearing. 6 sensor data (motor input current, bearing outer race torque, test bearing temperature, and support bearing top and bottom temperature) were used in each case. Before training, min-max scaler was used to avoid biased toward a specific range of values. The model has 3 hidden layers with 0.25 dropout for each. Mean absolute percentage error (MAPE) and Root mean square error (RMSE) were used for evaluating the model. By applying SHapley Additive exPlanations (SHAP), it was confirmed that the current is the most attributing feature for the RUL prediction model, then the torque. Temperature also attributes to the model in order of distance away from the test bearing.
更多查看译文
关键词
useful life prediction model,bearing
AI 理解论文
溯源树
样例
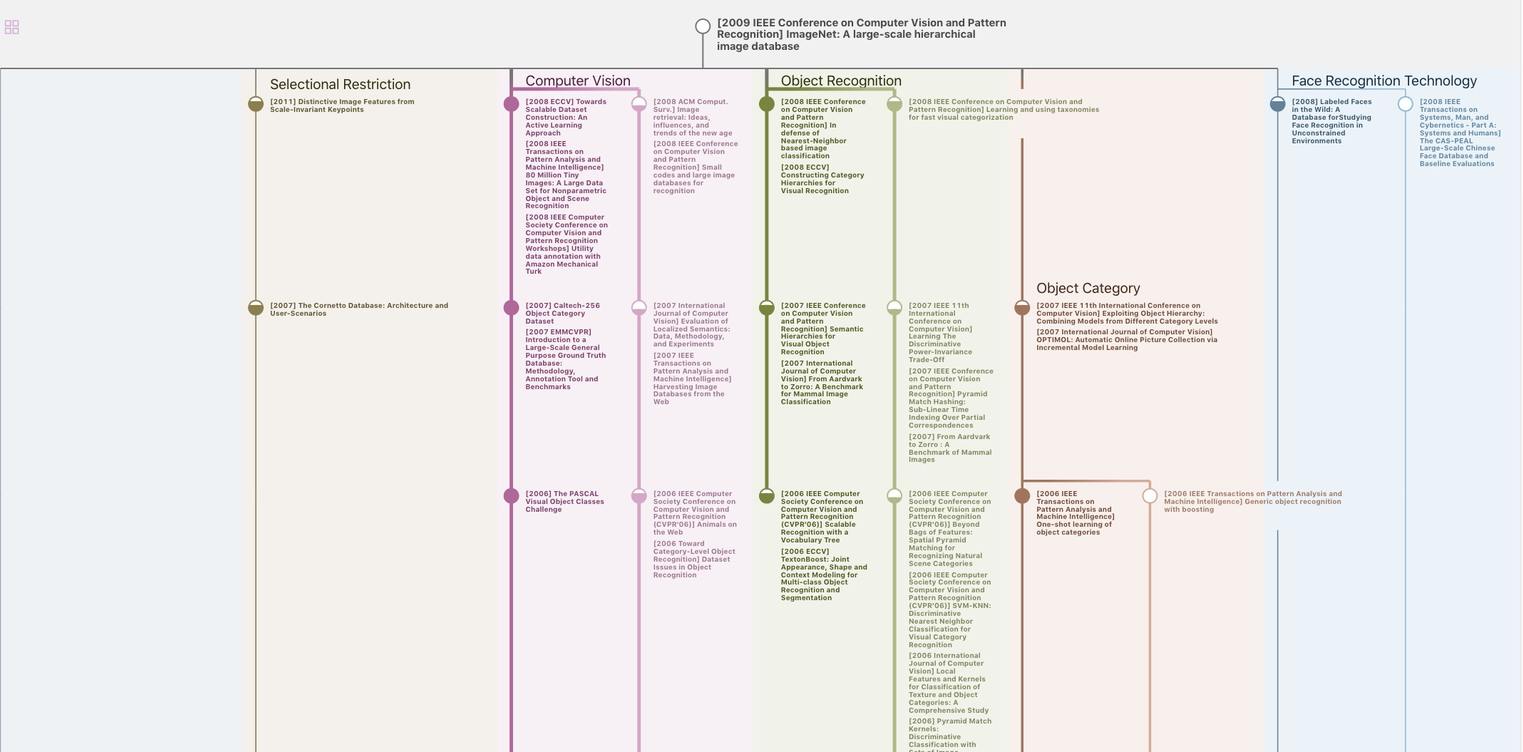
生成溯源树,研究论文发展脉络
Chat Paper
正在生成论文摘要