Sparse Bayesian Learning Approach for Compound Bearing Fault Diagnosis.
IEEE transactions on industrial informatics(2024)
摘要
Compound bearing fault diagnosis is an essentially challenging task due to the mutual interference among multiple fault components. The state-of-the-art methods usually take the potential fault characteristic frequencies as the prior knowledge and then try to recover every fault component by exploiting the impulse signal sparsity. However, they inevitably suffer from algorithmic degradation caused by energy leakage, l(1)-norm approximation, and/or improper parameter selection. To handle these shortcomings, in this paper, we propose a novel sparse Bayesian learning (SBL)-based method for the compound bearing fault diagnosis. We first present a new categorical probabilistic model to efficiently capture the truly-occurred fault components with a truncated feasible domain, which can greatly reduce the energy leakage effect. Then, we devise a more general SBL framework to recover the compound sparse impulse signal under the new categorical probabilistic model. The newly proposed method successfully avoids the l1 -norm approximation and manual parameter selection, thus it can yield much higher accuracy and robustness. Both simulations and experiments demonstrate the superiority of the developed method.
更多查看译文
关键词
Compound bearing fault diagnosis,sparse representation,group-sparsity,sparse Bayesian learning
AI 理解论文
溯源树
样例
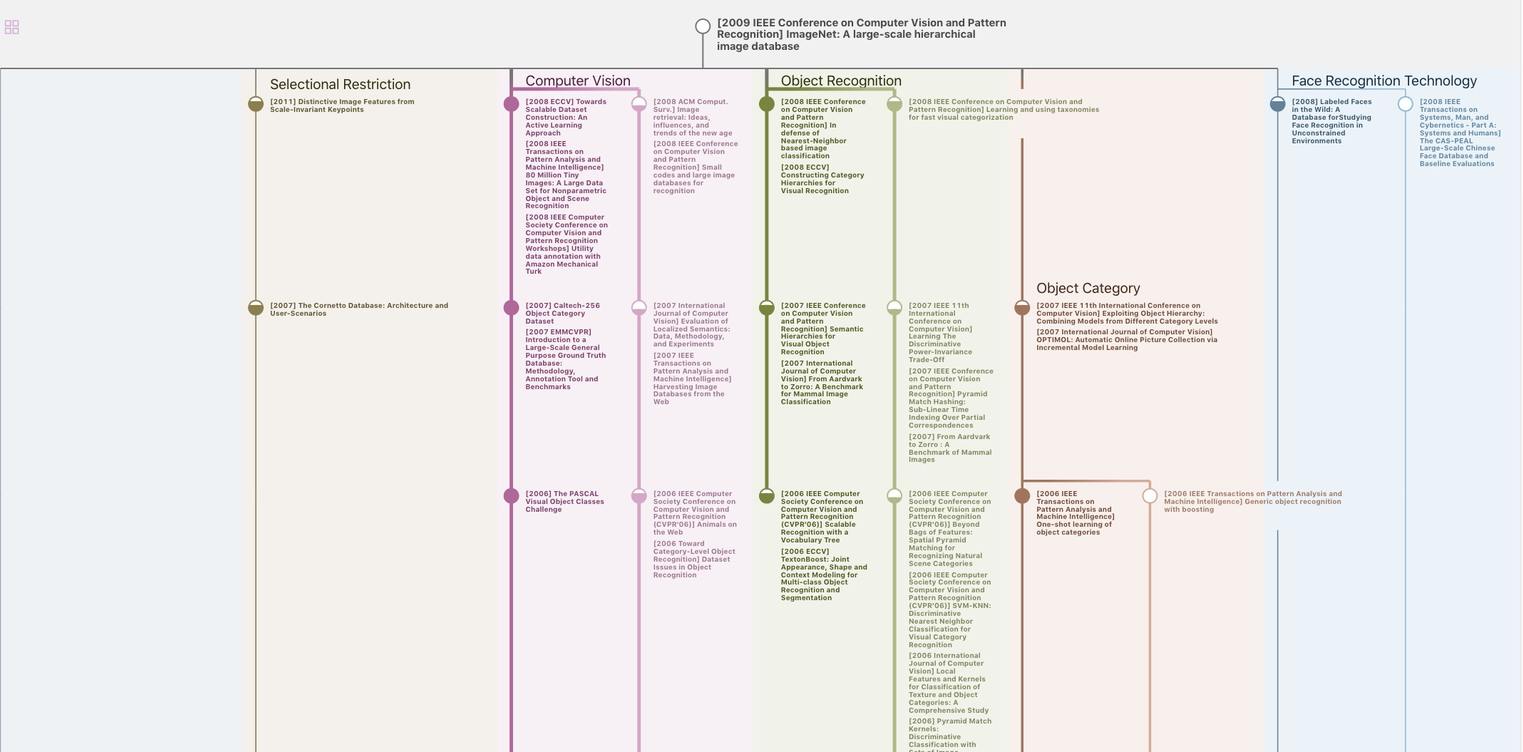
生成溯源树,研究论文发展脉络
Chat Paper
正在生成论文摘要