Dual-Metric Neural Network With Attention Guidance for Surface Defect Few-Shot Detection in Smart Manufacturing
JOURNAL OF MANUFACTURING SCIENCE AND ENGINEERING-TRANSACTIONS OF THE ASME(2023)
摘要
As an important application of human-robot collaboration, intelligent detection of surface defects is crucial for production quality control, which also helps in relieving the workload of technical staff in human-centric smart manufacturing. To accurately detect defects with limited samples in industrial practice, a dual-metric neural network with attention-guided is proposed. First, an attention-guided recognition network with channel attention and position attention module is designed to efficiently learn representative defect features with limited samples. Second, aiming to detect defects with confusing surface images, a dual-metric function is presented to learn the classification boundary by controlling the distance of samples in feature space from intraclass and interclass. The experiment results on the fabric defect dataset demonstrate that the proposed approach outperforms state-of-the-art methods in accuracy, recall, precision, F1-score, and few-shot accuracy. Further comparative experiments reveal that the dual-metric function is superior in improving the few-shot detection accuracy.
更多查看译文
关键词
few-shot learning,metric learning,surface defect detection,fabric defect
AI 理解论文
溯源树
样例
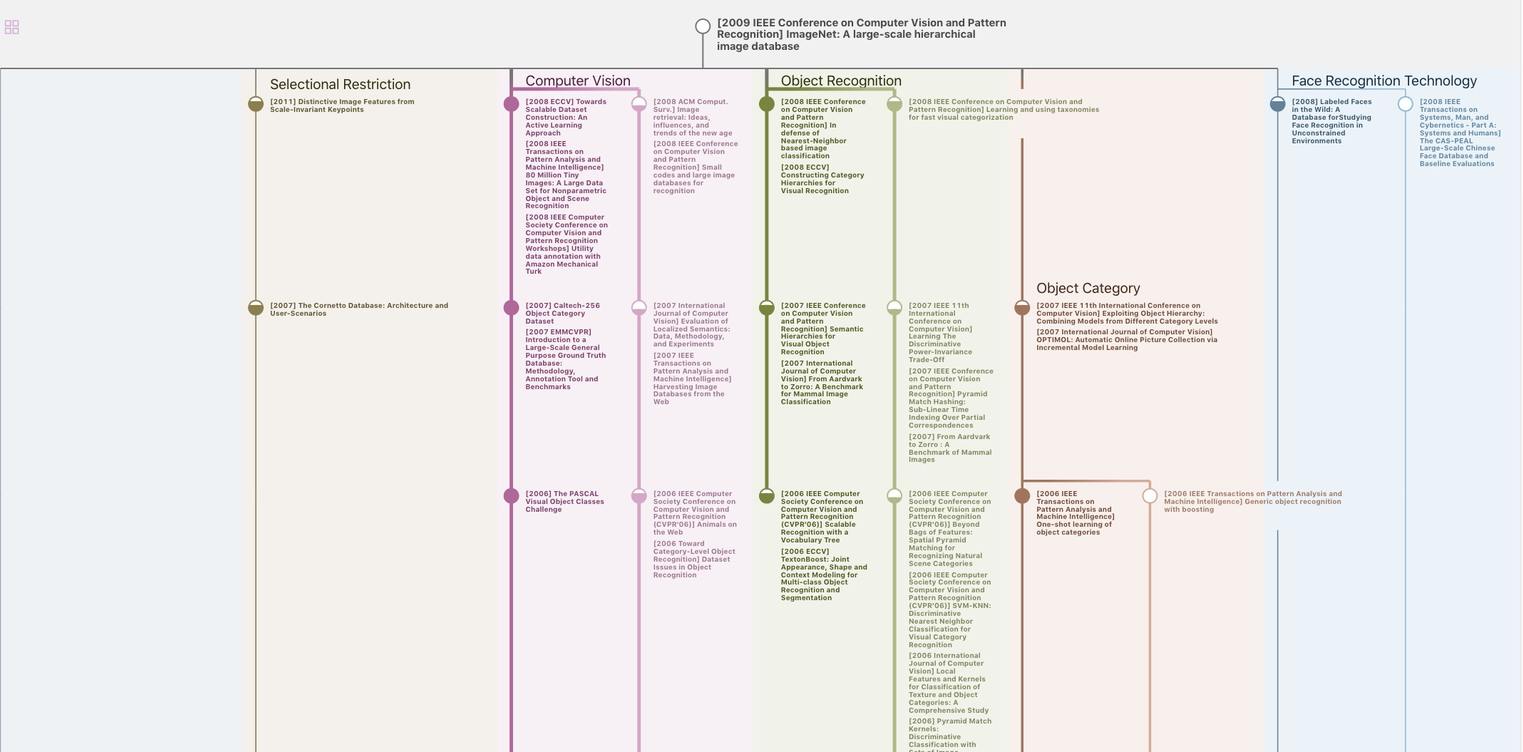
生成溯源树,研究论文发展脉络
Chat Paper
正在生成论文摘要