Huber Principal Component Analysis for Large-dimensional Factor Models
arXiv (Cornell University)(2023)
摘要
Factor models have found widespread applications in economics and finance, but the heavy-tailed character of macroeconomic and financial data is often overlooked in the existing literature. To address this issue and achieve robustness, we propose an approach to estimate factor loadings and scores by minimizing the Huber loss function, motivated by the equivalence of conventional Principal Component Analysis (PCA) and the constrained least squares method in the factor model. We provide two algorithms based on different penalty forms. The first minimizes the $\ell_2$-norm-type Huber loss, performing PCA on the weighted sample covariance matrix and is named Huber PCA. The second version minimizes the element-wise type Huber loss and can be solved by an iterative Huber regression algorithm. We investigate the theoretical minimizer of the element-wise Huber loss function and show that it has the same convergence rate as conventional PCA under finite second-moment conditions on idiosyncratic errors. Additionally, we propose a consistent model selection criterion based on rank minimization to determine the number of factors robustly. We demonstrate the advantages of Huber PCA using a real financial portfolio selection example, and an R package called ``HDRFA" is available on CRAN to conduct robust factor analysis.
更多查看译文
关键词
principal component analysis,factor,models,large-dimensional
AI 理解论文
溯源树
样例
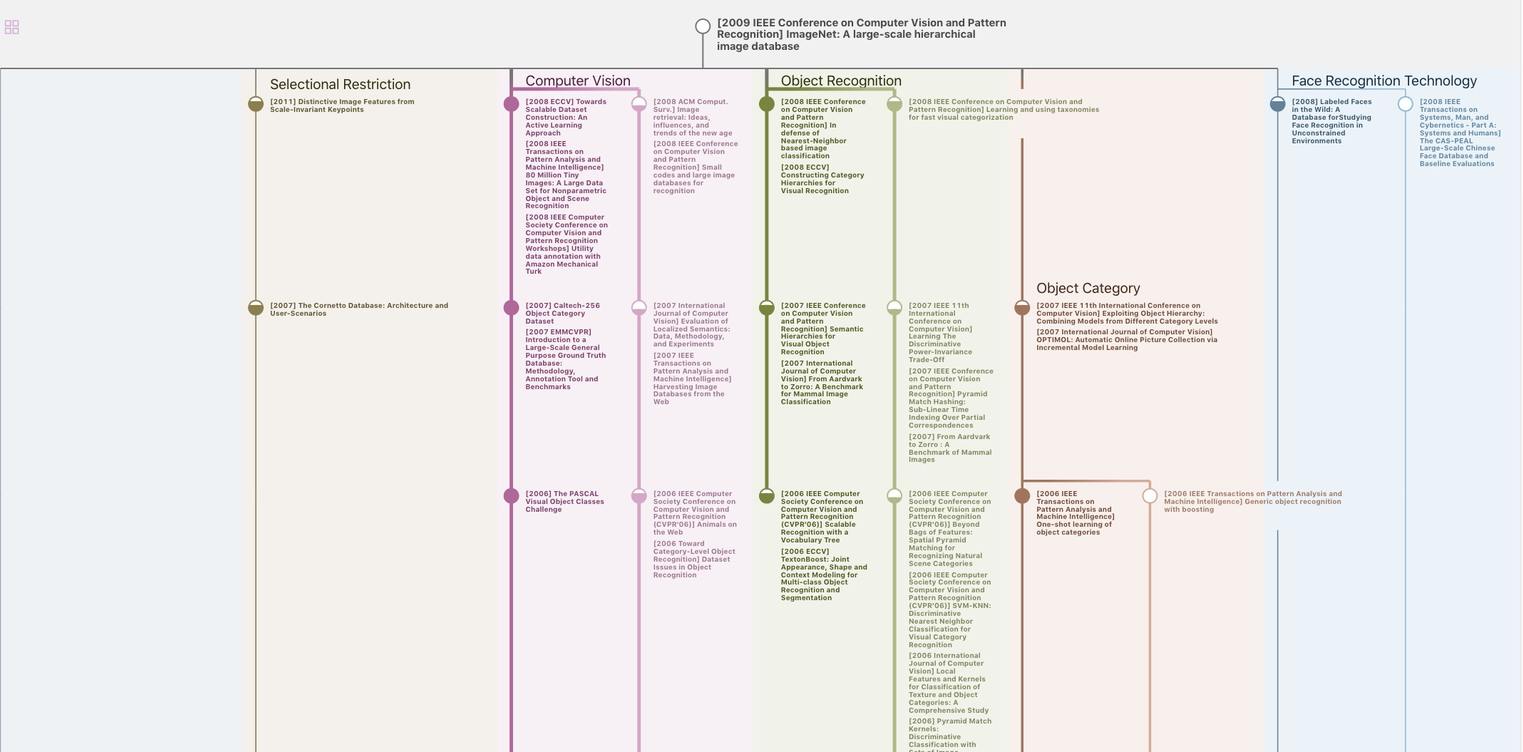
生成溯源树,研究论文发展脉络
Chat Paper
正在生成论文摘要