Accuracy and lower limits for iodine quantification in dual-energy CT with deep learning reconstruction
Research Square (Research Square)(2023)
摘要
Abstract Iodine quantification is a diagnostic indicator of vascularization in lesions; however, accurate and repeatable measurements, especially at low iodine concentrations, are challenging due to the limits of instruments used for iodine quantification. Measurements of iodine concentrations below the instrument limits are unrepeatable and can lead to misdiagnoses. Although the accuracies of iodine quantification have been reported, no prior studies have comprehensively examined the limits of the instrument. Therefore, this study aimed to evaluate the accuracy, limit of detection (LOD), and limit of quantification (LOQ) of iodine quantification using fast kilovoltage-switching dual-energy computed tomography (DECT). We used iodine inserts with seven nominal iodine concentration and performed DECT scans in small and large phantom at different CT dose indexes. We assessed accuracy using relative measurement errors, calculated the LOD using calibration curves between the nominal and measured iodine densities, and examined the LOQ using the relative standard deviation of repeated measurements. We found that LOD and LOQ decreased with increasing CT dose indices and were lower for the small phantom than for the large phantom. The measured iodine densities plateaued for 0.3 and 0.5 mgI/mL in small and large phantoms, respectively, and these results indicate the limits of instrument used for iodine quantification. The relative measurement errors decreased with increasing nominal iodine concentrations above the LOD or LOQ levels. However, no clear trend was observed below these limits. Therefore, these findings revealed the necessity of a comprehensive evaluation of accuracy and LOD/LOQ for iodine quantification to diagnose lesions reliably with iodine density.
更多查看译文
关键词
dual-energy dual-energy ct,deep learning reconstruction,iodine quantification
AI 理解论文
溯源树
样例
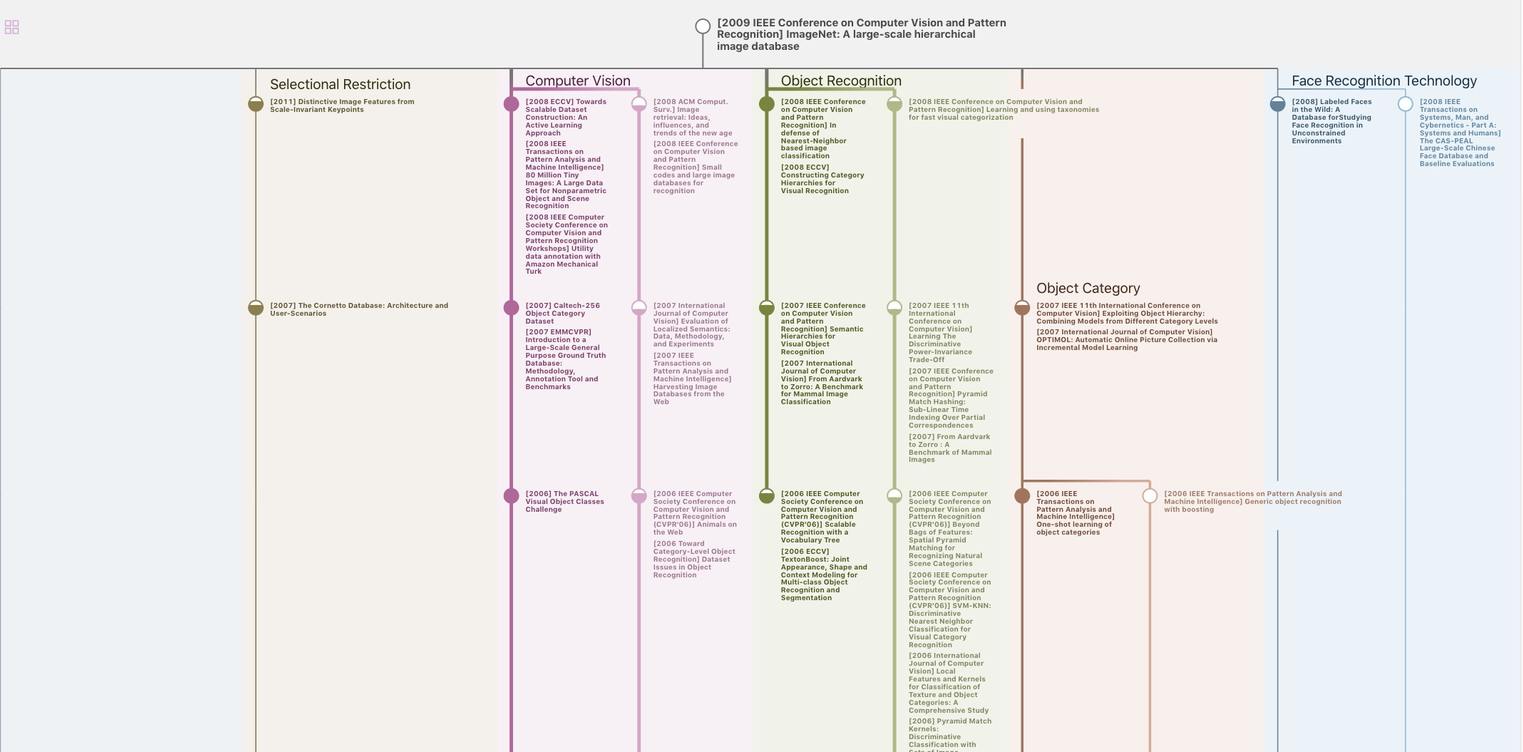
生成溯源树,研究论文发展脉络
Chat Paper
正在生成论文摘要