Abstract 6625: Mathematical modeling of single-cell data reveals the key role of stochasticity - not fitness - in determining the clonal origins of relapse in childhood leukemia
Cancer Research(2023)
摘要
Abstract Acute lymphoblastic leukemia (cALL) is the most common pediatric cancer, and since up to 20% of children relapse after initially responding to chemotherapy treatment, the largest cause of cancer-related childhood deaths in the western world. With the idea that dissecting the evolutionary population dynamics leading to relapse would help explain treatment failure from a mechanistic standpoint, several studies have performed comparisons of genetic heterogeneity at diagnosis and relapse. These showed that as many as 70% of relapses are dominated by a single diagnostic subclone or one of its evolutionary progeny; leading to the prevailing view that treatment selection in cALL primarily operates at the genotype level. However, a crucial caveat of these studies is their reliance, due primarily to technical and economic constraints, on very limited data timepoints. While the dynamics linking said timepoints are often interpreted based on simple intuition. To overcome this sparse data challenge and reconstruct the entire spatial and temporal evolutionary trajectories of individual cALL cells from diagnosis to relapse, we have integrated experimental data with an in silico model. Single-cell WGS and RNAseq data were obtained from primary and xenografted leukemic cells before and after chemotherapy. These were then used to parameterize an agent-based mathematical model allowing tracking of the genotype and evolving phenotype of individual cells over time. When short treatment courses akin to those we delivered in vivo were used, the in silico model closely recapitulated the experimental results. Since cALL treatment lasts several years, it cannot be entirely modelled in vivo. Due to the rarity of post-treatment residual cells and the lack of universal cell surface markers to isolate them, direct analysis of clinical samples is also challenging. However, based on the initial treatment data, our calibrated model provided a unique opportunity to simulate longer remission-relapse dynamics of several leukemias. Mechanistic analysis of the collected data showed that, strikingly, in most cases, clonal dominance at relapse results from stochastic sweeps rather than deterministic differences in clonal fitness. Indeed, independent treatment of the same leukemia with an identical drug regimen more frequently ended with a considerably different clonal landscape (either polyclonal disease or a different dominant clone) than with the same outcome initially observed. Rather than genotype differences, the size of residual disease and a founder effect linked to the stochastic exit, post-treatment, of individual cells from quiescence were the key determinants of the relapse genetic landscape. Altogether, our data highlight the misinterpretation risk associated with snapshot analysis and might inform promising alternative treatment schedules. Citation Format: Virginia Turati, Javier Herrero, Mark Robertson-Tessi, Tariq Enver, Andriy Marusyk, Alexander RA Anderson. Mathematical modeling of single-cell data reveals the key role of stochasticity - not fitness - in determining the clonal origins of relapse in childhood leukemia [abstract]. In: Proceedings of the American Association for Cancer Research Annual Meeting 2023; Part 1 (Regular and Invited Abstracts); 2023 Apr 14-19; Orlando, FL. Philadelphia (PA): AACR; Cancer Res 2023;83(7_Suppl):Abstract nr 6625.
更多查看译文
关键词
stochasticity,clonal origins,mathematical modeling,single-cell
AI 理解论文
溯源树
样例
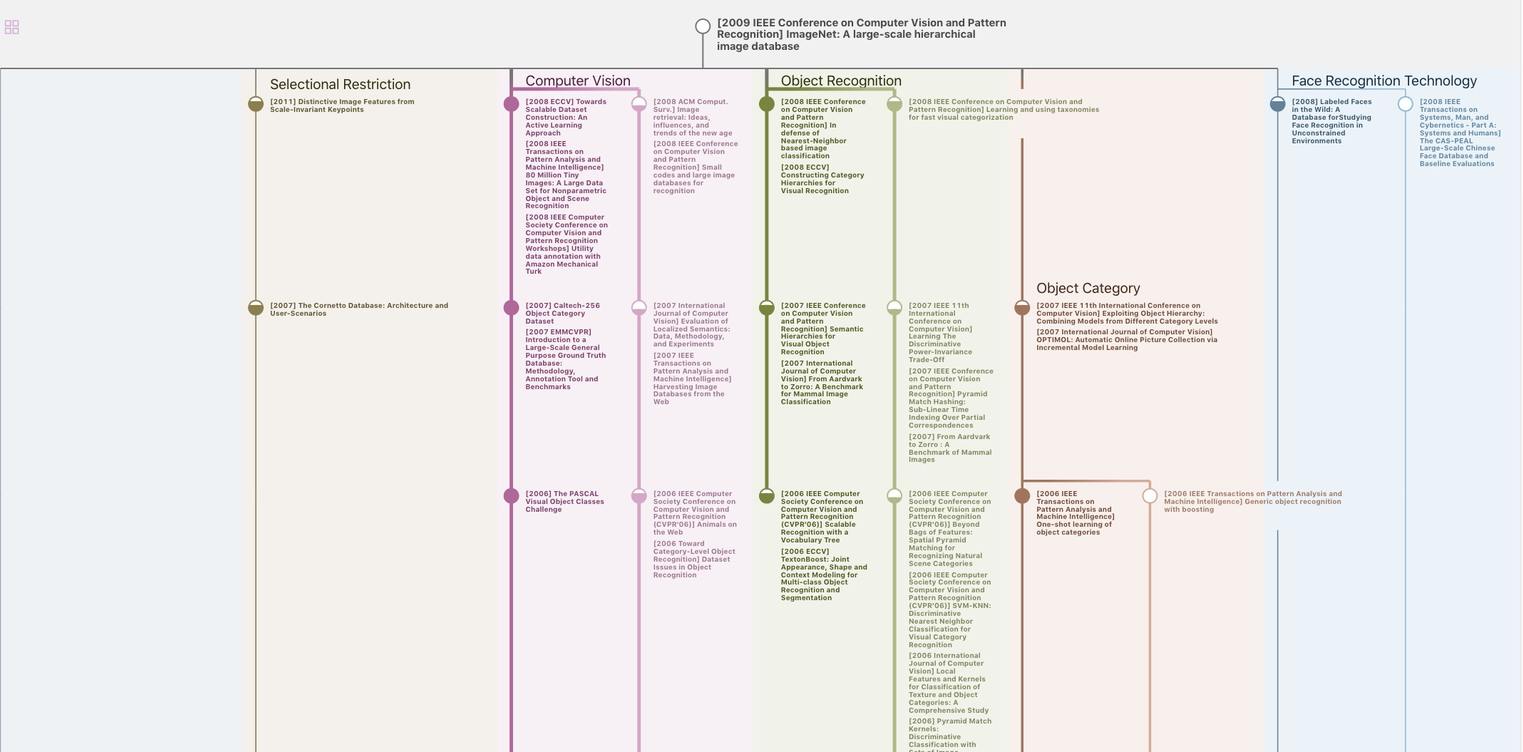
生成溯源树,研究论文发展脉络
Chat Paper
正在生成论文摘要