Analysis of long-term sea level fluctuation patterns and influences of climatic indices around the Korean Peninsula through dimensionality reduction of altimeter data
Elsevier eBooks(2023)
Abstract
Sea-level height is an important indicator related to climate change; although the global sea level has risen, it differs across regions. Satellite data are structured in the three-dimensional array, and it has recently been proposed to analyze massive volumes of data to reduce the data's dimensions with the development of data mining. The empirical orthogonal function (EOF) and self-organizing maps (SOMs) are examples of these approaches. By reducing the dimension of the sea-level anomaly (SLA) and sea surface temperature (SST) data, in the present study, we investigated the sea-level change around the Korean Peninsula and found climatic indices related to climate change. EOF modes identified the annual and seasonal cycles in SLA, including long-term cycles related to the wind, temperature, SST, or currents. SLA data clustered the SOM analysis by 2 × 3 grid of nodes, and the results showed the high sea-level patterns in summer in recent and low sea-level patterns in winter in the early 1990s. Also, positive and negative climatic indices were clustered by the node. Therefore, clustering nonlinear characteristics by using SOM is more effective than EOF analysis. It is considered that the clustered outputs with SOM could be used as preprocessing data via labeling to estimate the sea-level height or SST. It is also likely to be recognized as pattern-based interpolating the missing data.
MoreTranslated text
Key words
climatic indices,korean peninsula,dimensionality reduction,long-term
AI Read Science
Must-Reading Tree
Example
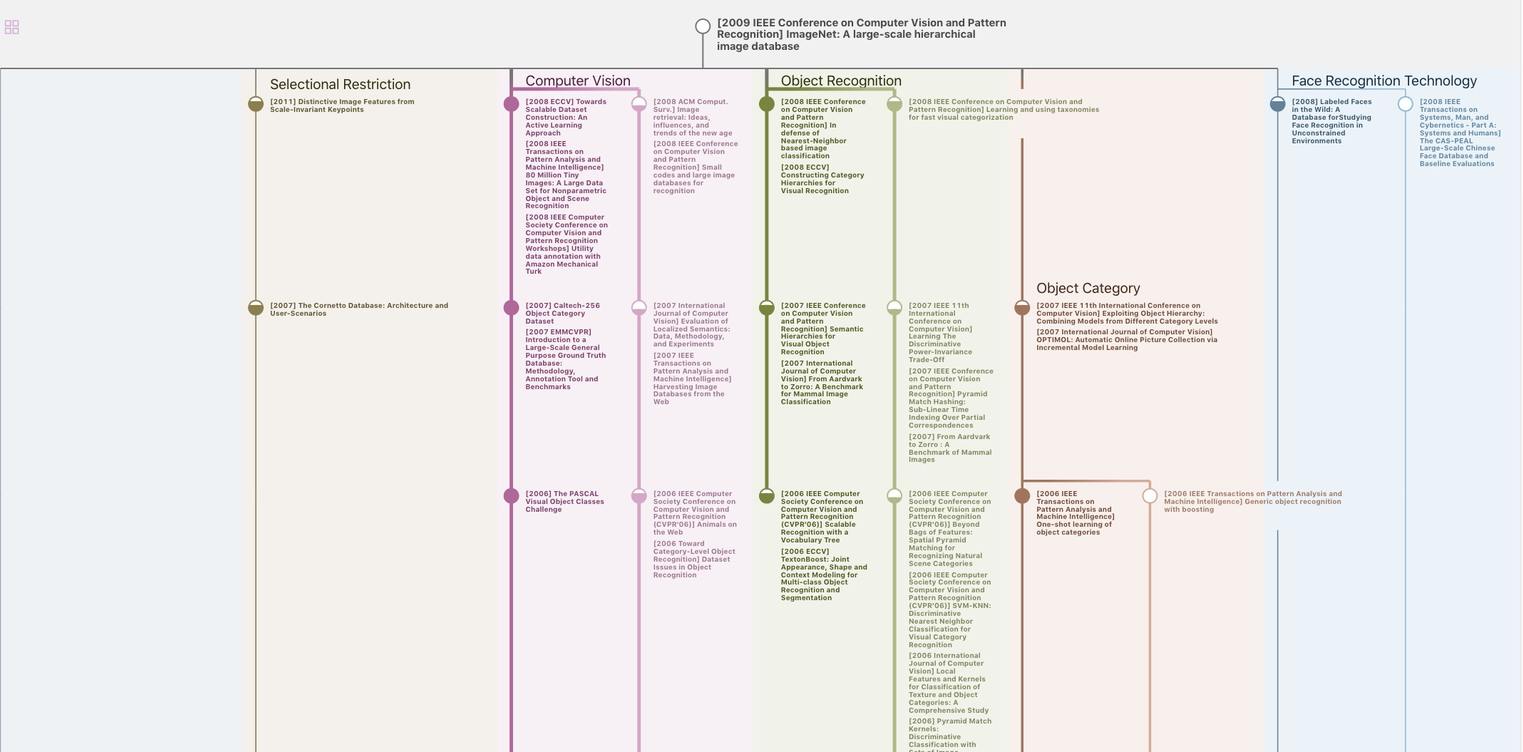
Generate MRT to find the research sequence of this paper
Chat Paper
Summary is being generated by the instructions you defined