Dynamic tariffs-based demand response in retail electricity market under uncertainty
arXiv (Cornell University)(2021)
摘要
Demand response (DR) programs have gained much attention after the restructuring of the electricity markets and have been used to optimize the decisions of market participants. They can potentially enhance system reliability and manage price volatility by modifying the amount or time of electricity consumption. This paper proposes a novel game-theoretical model accounting for the relationship between retailers (leaders) and consumers (followers) in a dynamic price environment under uncertainty. The quality and economic gains brought by the proposed procedure essentially stem from the utilization of demand elasticity in a hierarchical decision process that renders the options of different market configurations under different sources of uncertainty. The model is solved under two frameworks: by considering the retailer's market power and by accounting for an equilibrium setting based on a perfect competitive game. These are formulated in terms of a mathematical program with equilibrium constraints (MPEC) and with a mixed-integer linear program (MILP), respectively. In particular, the retailers' market power model is first formulated as a bi-level optimization problem, and the MPEC is subsequently derived by replacing the consumers' problem (lower level) with its Karush-Kuhn-Tucker (KKT) optimality conditions. In contrast, the equilibrium model is solved as a MILP by concatenating the retailer's and consumers' KKT optimality conditions. We illustrate the proposed procedure and numerically assess the performance of the model using realistic data. Numerical results show the applicability and effectiveness of the proposed model to explore the interactions of market power and DR programs. The results confirm that consumers are better off in an equilibrium framework while the retailer increases its expected profit when exercising its market power.
更多查看译文
关键词
retail electricity market,demand response,uncertainty,tariffs-based
AI 理解论文
溯源树
样例
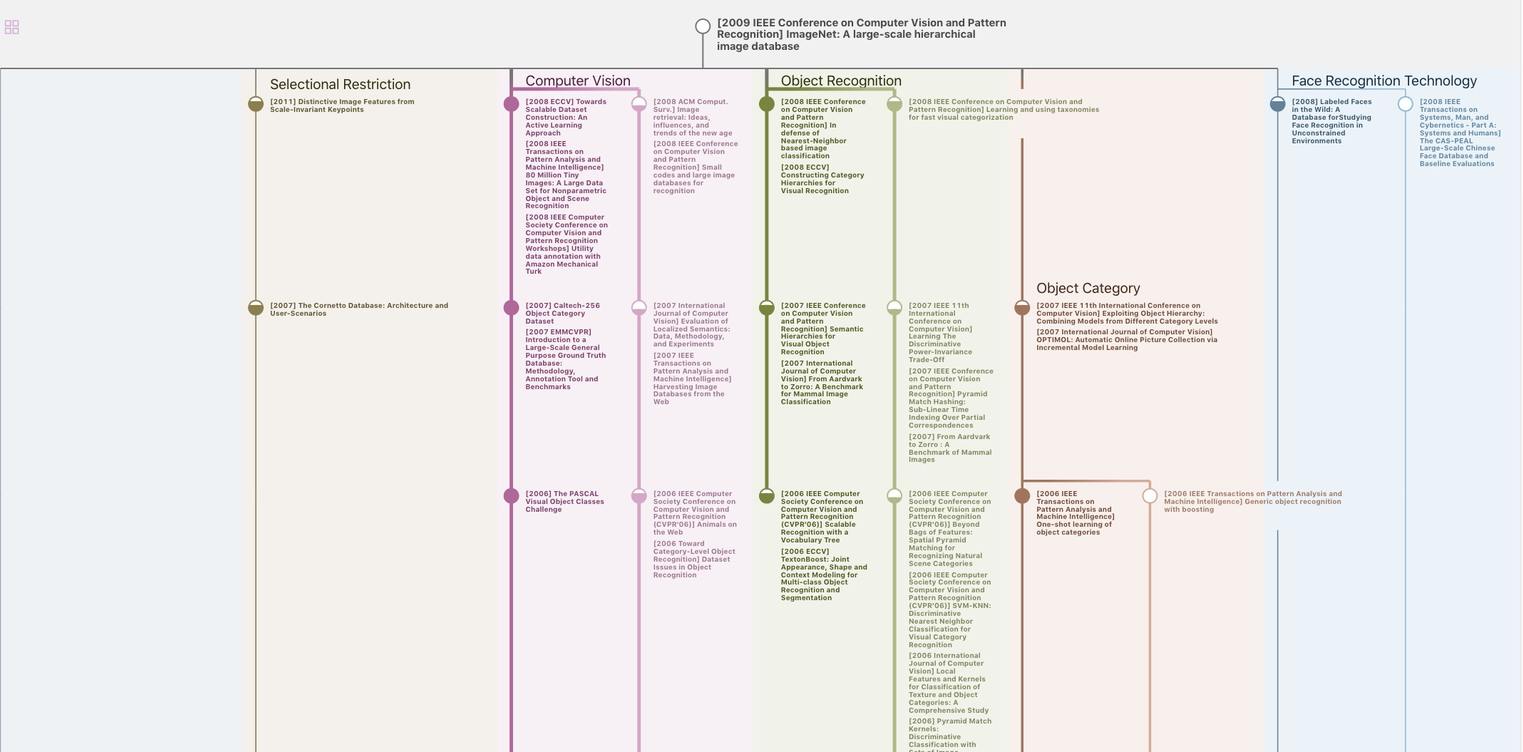
生成溯源树,研究论文发展脉络
Chat Paper
正在生成论文摘要