Data-Driven Early Warning for Dynamic Insecurity Risk of Hybrid AC/DC Power Grids
2023 International Conference on Power System Technology (PowerCon)(2023)
摘要
The dynamic interactions between AC and DC systems and the uncertainty of renewable generation increase the risk of cascading failures and bring challenges for the secure operation of hybrid AC/DC grids. Insecurity early warning identifies the security risk and guides the control decision-making, which is important for the secure power system operation. To achieve fast insecurity early warning, a data-driven early warning method for dynamic insecurity risk is proposed in this paper. First, Latin hypercube sampling and improved K-medoids algorithm are utilized to generate typical operating conditions (OCs) in the future. Then, stacking denoising autoencoders and ensemble extreme learning machines are deployed to construct security risk assessment models to fast assess the dynamic security of typical OCs. And model update is performed to ensure the assessment accuracy when cascading failures occur. Finally, the insecure OCs are ranked into different insecure levels based on the control cost needed to ensure the system security. Simulation results of the Northwest hybrid AC/DC grid in China demonstrate that the proposed method can achieve fast insecurity early warning and effectively track the security risk tendency during the process of cascading failures.
更多查看译文
关键词
security risk,early warning,hybrid AC/DC grids,deep learning,data-driven
AI 理解论文
溯源树
样例
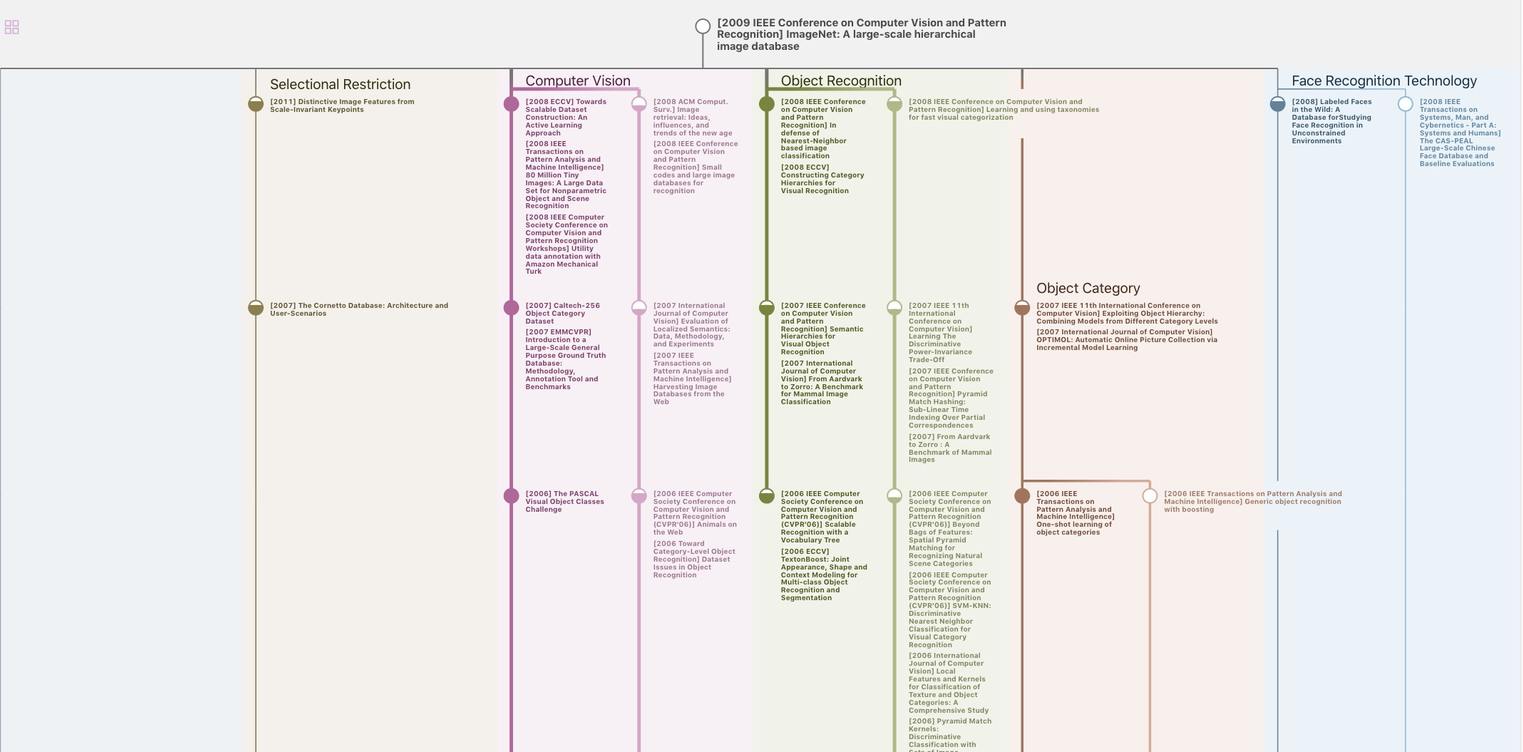
生成溯源树,研究论文发展脉络
Chat Paper
正在生成论文摘要