The Application of Physics-Informed Machine Learning in Multiphysics Modeling in Chemical Engineering
INDUSTRIAL & ENGINEERING CHEMISTRY RESEARCH(2023)
摘要
Physics-Informed Machine Learning (PIML) is an emerging computing paradigm that offers a new approach to tackle multiphysics modeling problems prevalent in the field of chemical engineering. These problems often involve complex transport processes, nonlinear reaction kinetics, and multiphysics coupling. This Review provides a detailed account of the main contributions of PIML with a specific emphasis on modeling momentum transfer, heat transfer, mass transfer, and chemical reactions. The progress in method development (e.g., algorithm and architecture), software libraries, and specific applications (e.g., multiphysics coupling and surrogate modeling) are detailed. On this basis, future challenges highlight the importance of developing more practical solutions and strategies for PIML, including turbulence models, domain decomposition, training acceleration, surrogate modeling, hybrid modeling, and geometry module creation.
更多查看译文
关键词
multiphysics modeling,chemical engineering,machine learning,physics-informed
AI 理解论文
溯源树
样例
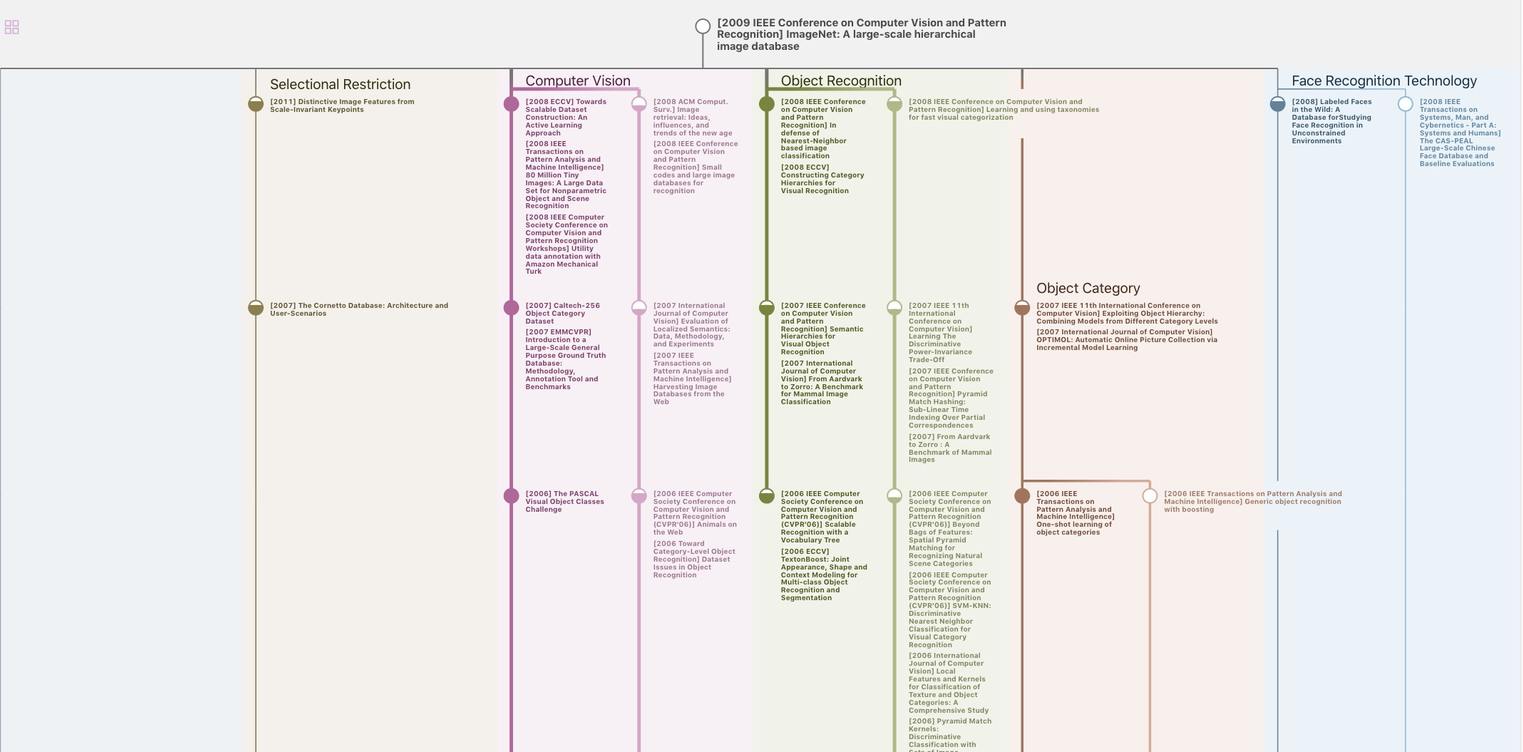
生成溯源树,研究论文发展脉络
Chat Paper
正在生成论文摘要