Mapping Multi-Depth Soil Salinity Using Remote Sensing-Enabled Machine Learning in the Yellow River Delta, China
REMOTE SENSING(2023)
摘要
Soil salinization is a crucial type in the degradation of coastal land, but its spatial distribution and drivers have not been sufficiently explored especially at the depth scale owing to its multidimensional nature. In this study, we proposed a multi-depth soil salinity prediction model (0-10 cm, 10-20 cm, 20-40 cm, and 40-60 cm) fully using the advantages of satellite image data and field sampling to rapidly estimate the multi-depth soil salinity in the Yellow River Delta, China. Firstly, a multi-depth soil salinity predictive factor system was developed through correlation analysis of soil sample electrical conductivity with a series of remote-sensing parameters containing heat, moisture, salinity, vegetation indices, spectral value, and spatial location. Then, three machine learning methods including back propagation neural network (BPNN), support vector machine (SVM), and random forest (RF) were adopted to construct a coastal soil salinity inversion model. By using the best inversion model, we obtain the spatial distribution of soil salinity in the Yellow River Delta. The results show the following: (1) Environmental variables in this study are all effective variables for soil salinity prediction. The most sensitive indicators to multi-depth soil salinity are GDVI, ENDVI, SI-T, NDWI, and LST. (2) The RF model was chosen as the optimal approach for predicting and mapping soil salinity based on performance at four soil depths. (3) The soil salinity profiles exhibited intricate coexistence of two distinct types: surface aggregated and homogeneous. The former was dominant in the east, where salinity was higher. The central and southwestern parts were mostly homogeneous, with lower soil salinity. (4) The soil salinity throughout the four depths examined was found to be most elevated in saltern and bare land and lowest in wetland vegetation and farmland, according to land-cover type. This study proposed a remote sensing prediction method for salinization in multiple soil layers in the coastal plain, which could provide decision support for spatial monitoring of land salinization and achieving land degradation neutrality targets.
更多查看译文
关键词
spatial distribution,soil layer,soil salinization,vertical soil salinity,machine learning
AI 理解论文
溯源树
样例
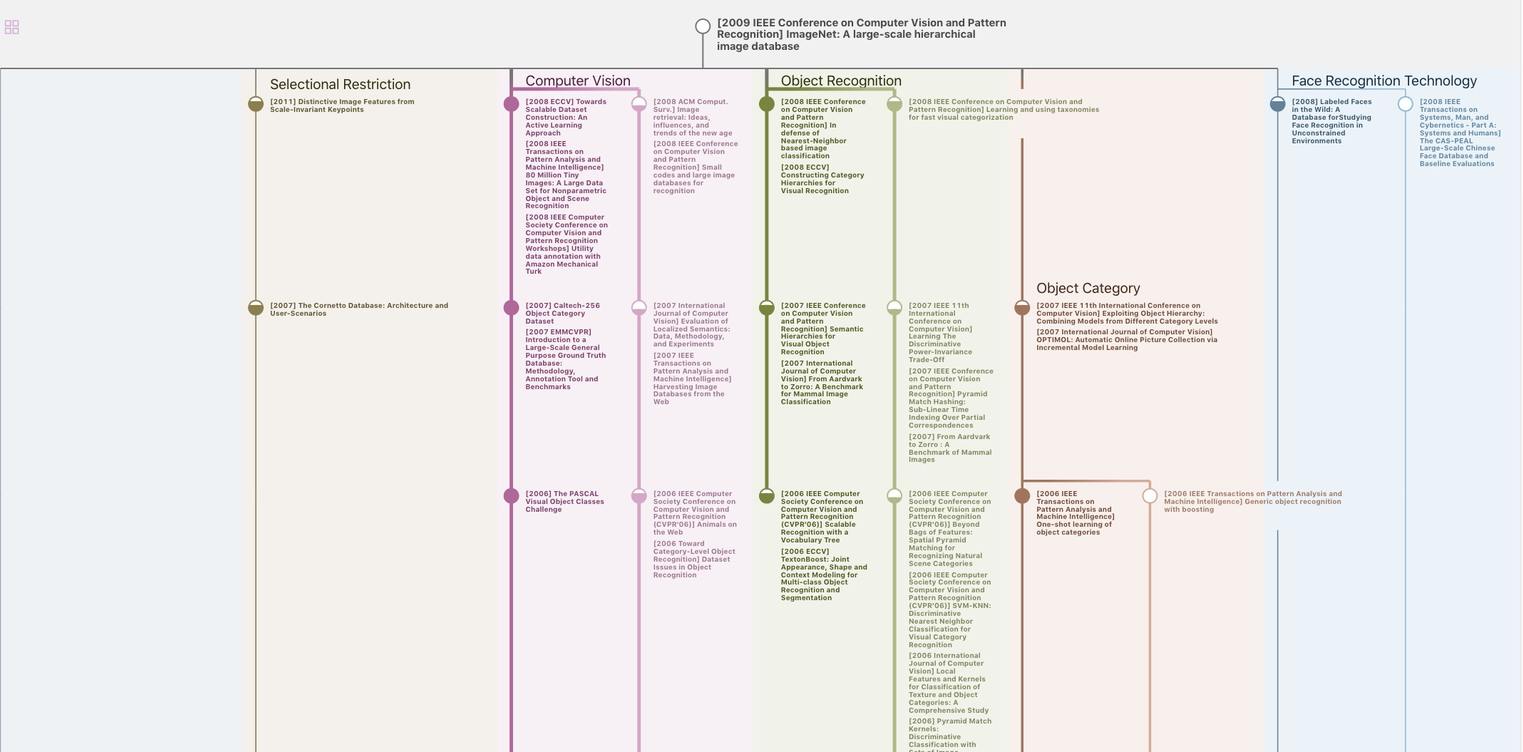
生成溯源树,研究论文发展脉络
Chat Paper
正在生成论文摘要