Estimating network flow and travel behavior using day-to-day system-level data: A computational graph approach
TRANSPORTATION RESEARCH PART C-EMERGING TECHNOLOGIES(2024)
Abstract
To estimate network flow and travel behavior under recurrent traffic conditions, we leverage computational graphs and multi-source system-level data and solve a single-level optimization problem consistent with stochastic user equilibrium under logit assignment (SUELOGIT). Our model learns time-specific O-D matrices and utility functions and network flow parameters such as link flows, path flows, and travel times. To increase the model's representational capacity for reproducing observed link flows and travel times, the parameters of the link performance functions are assumed link-specific. More importantly, the utility function in the route choice model is enriched with (i) link-specific parameters to capture the effect of unobserved attributes on route choices and (ii) period-specific parameters weighting the observed features in the utility function to capture the heterogeneity of travelers preferences among periods of the day. Experiments on synthetic data show that the parameters of the models can be consistently recovered and that the solution of the model satisfies the SUELOGIT conditions with high accuracy. The estimation procedure is also robust to random noise in both the observed traffic flow and travel time, and it requires very few hyperparameter tuning. Subsequently, the algorithm is deployed at a large scale using real-world multi-source data in Fresno, CA, with hourly data collected during the morning and afternoon peak periods of October 2019. The utility function includes link-specific effects and attributes such as travel time, the standard deviation of travel time, the number of traffic incidents, and socio-demographic information obtained from the US Census. We obtain estimates for the total trips and travelers' utility function by hour of the day and for the average values of the link performance parameters that are reasonable and informative on the demand and supply characteristics of the transportation network.
MoreTranslated text
Key words
Computational graphs,Network modeling,Logit-based stochastic user equilibrium,Origin-destination demand estimation
AI Read Science
Must-Reading Tree
Example
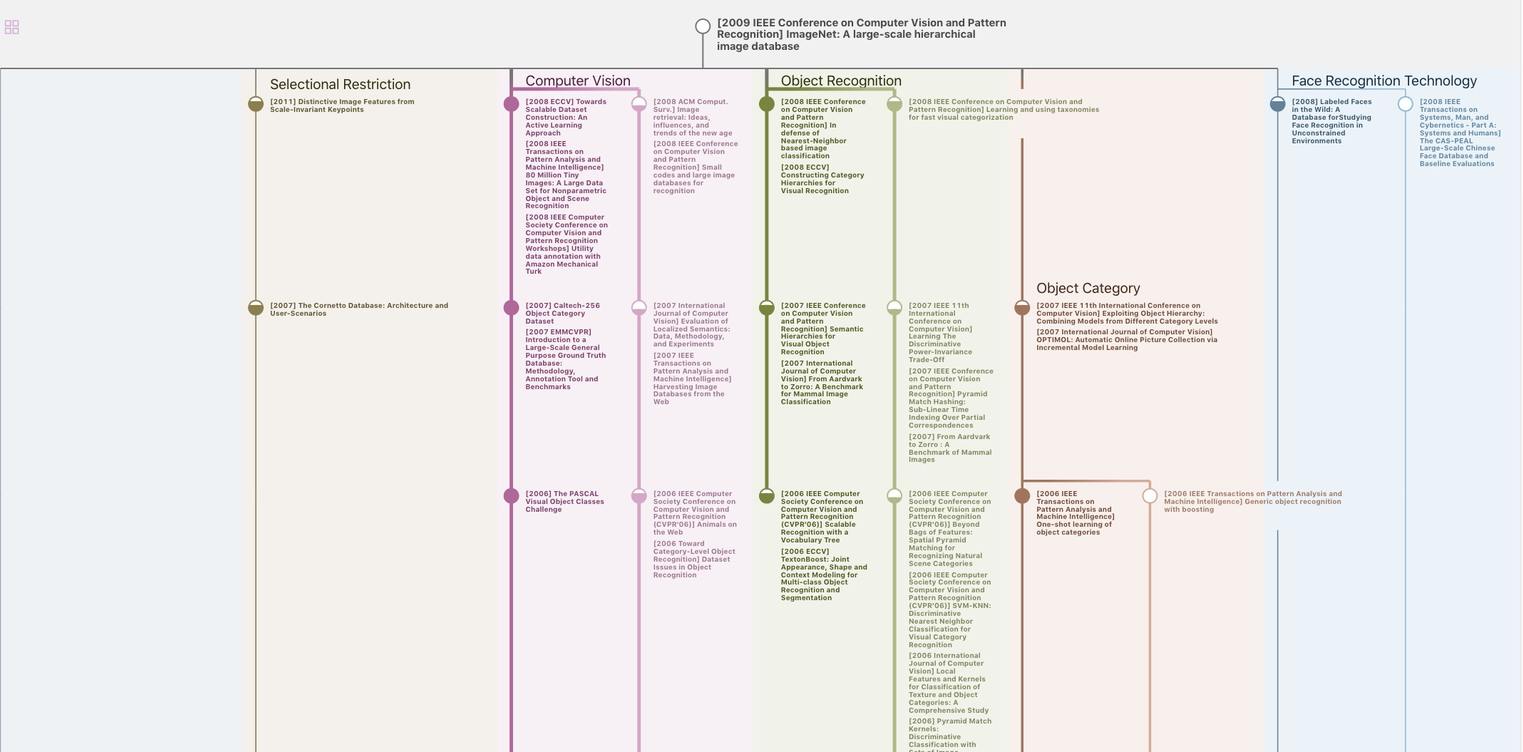
Generate MRT to find the research sequence of this paper
Chat Paper
Summary is being generated by the instructions you defined