Comparative Study of Gaussian Processes, Multi Layer Perceptrons, and Deep Kernel Learning for Indoor Visible Light Positioning Systems.
2023 13th International Conference on Indoor Positioning and Indoor Navigation (IPIN)(2023)
Abstract
In indoor localization, Received Signal Strength (RSS)-based Visible Light Positioning combined with Multi Layer Perceptrons (MLPs) or Gaussian processes (GPs) has attracted much attention due to its high accuracy. However, there is a lack of detailed investigation on the advantages, disadvantages, and applicability of MLPs and GPs in large datasets collected from representative industrial environments. In this paper, we present a comprehensive comparison and analysis of MLPs and GPs from theoretical and experimental perspectives, focusing on model parameters, complexity, and interpretability. Our study demonstrates that while GPs outperform MLPs on small datasets, they exhibit drawbacks such as high computational cost on larger datasets. Furthermore, our investigation reveals that including Batch Normalization (BN) layers in MLPs enhances their generalization and suppresses outliers in prediction. To address the issues of scalability and interpretability, we introduce the Deep Kernel Learning (DKL) model as a solution, supported by both theoretical and experimental findings.
MoreTranslated text
Key words
multi layer perceptrons,visible light positioning,gaussian process regression,deep kernel learning
AI Read Science
Must-Reading Tree
Example
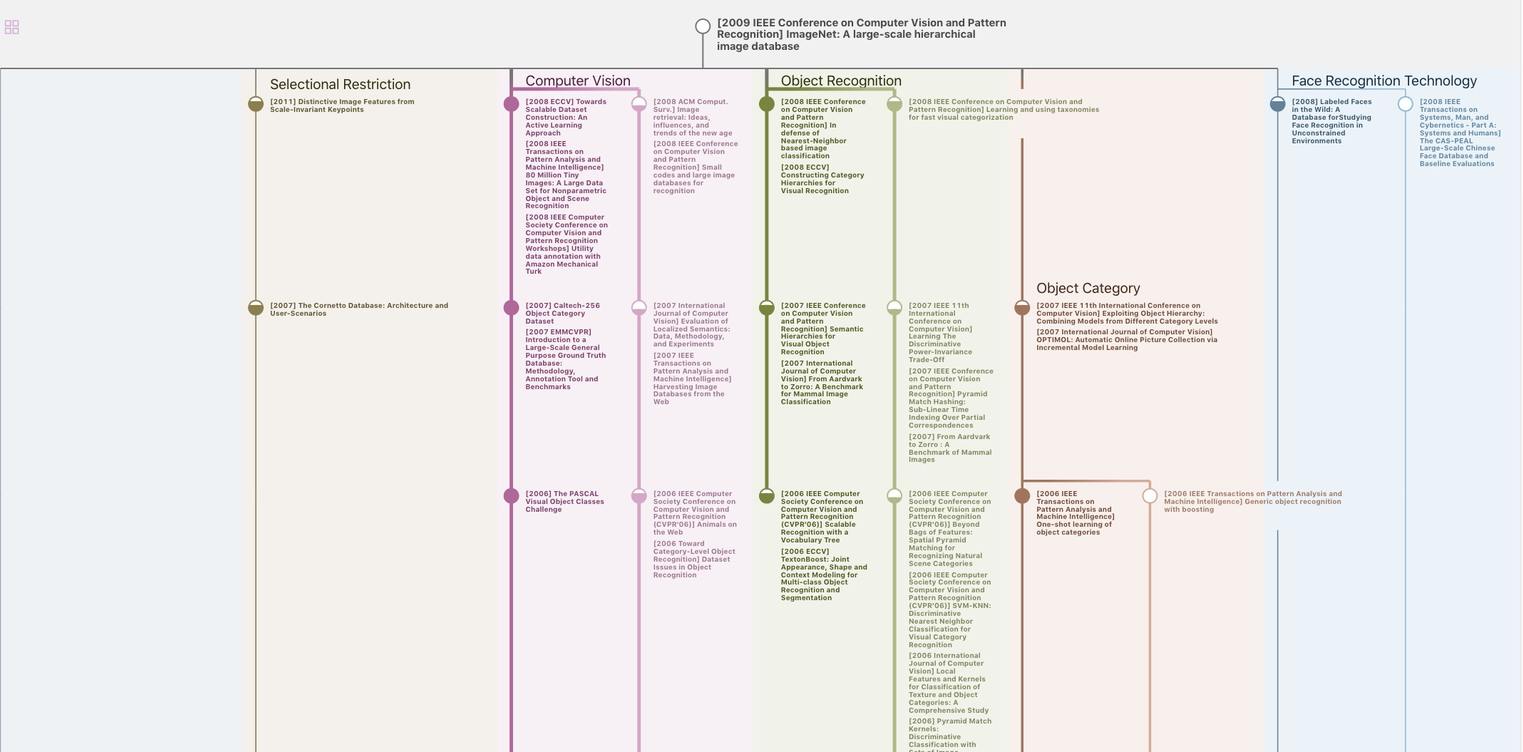
Generate MRT to find the research sequence of this paper
Chat Paper
Summary is being generated by the instructions you defined