Explainability-based Metrics to Help Cyber Operators Find and Correct Misclassified Cyberattacks.
SAFE '23: Proceedings of the 2023 on Explainable and Safety Bounded, Fidelitous, Machine Learning for Networking(2023)
摘要
Machine Learning (ML)-based Intrusion Detection Systems (IDS) have shown promising performance. However, in a human-centered context where they are used alongside human operators, there is often a need to understand the reasons of a particular decision. EXplainable AI (XAI) has partially solved this issue, but evaluation of such methods is still difficult and often lacking. This paper revisits two quantitative metrics, Completeness and Correctness, to measure the quality of explanations, i.e., if they properly reflect the actual behaviour of the IDS. Because human operators generally have to handle a huge amount of information in limited time, it is important to ensure that explanations do not miss important causes, and that the important features are indeed causes of an event. However, to be more usable, it is better if explanations are compact. For XAI methods based on feature importance, Completeness shows on some public datasets that explanations tend to point out all important causes only with a high number of features, whereas Correctness seem to be highly correlated with prediction results of the IDS. Finally, besides evaluating the quality of XAI methods, Completeness and Correctness seem to enable identification of IDS failures and can be used to point the operator towards suspicious activity missed or misclassified by the IDS, suggesting manual investigation for correction.
更多查看译文
AI 理解论文
溯源树
样例
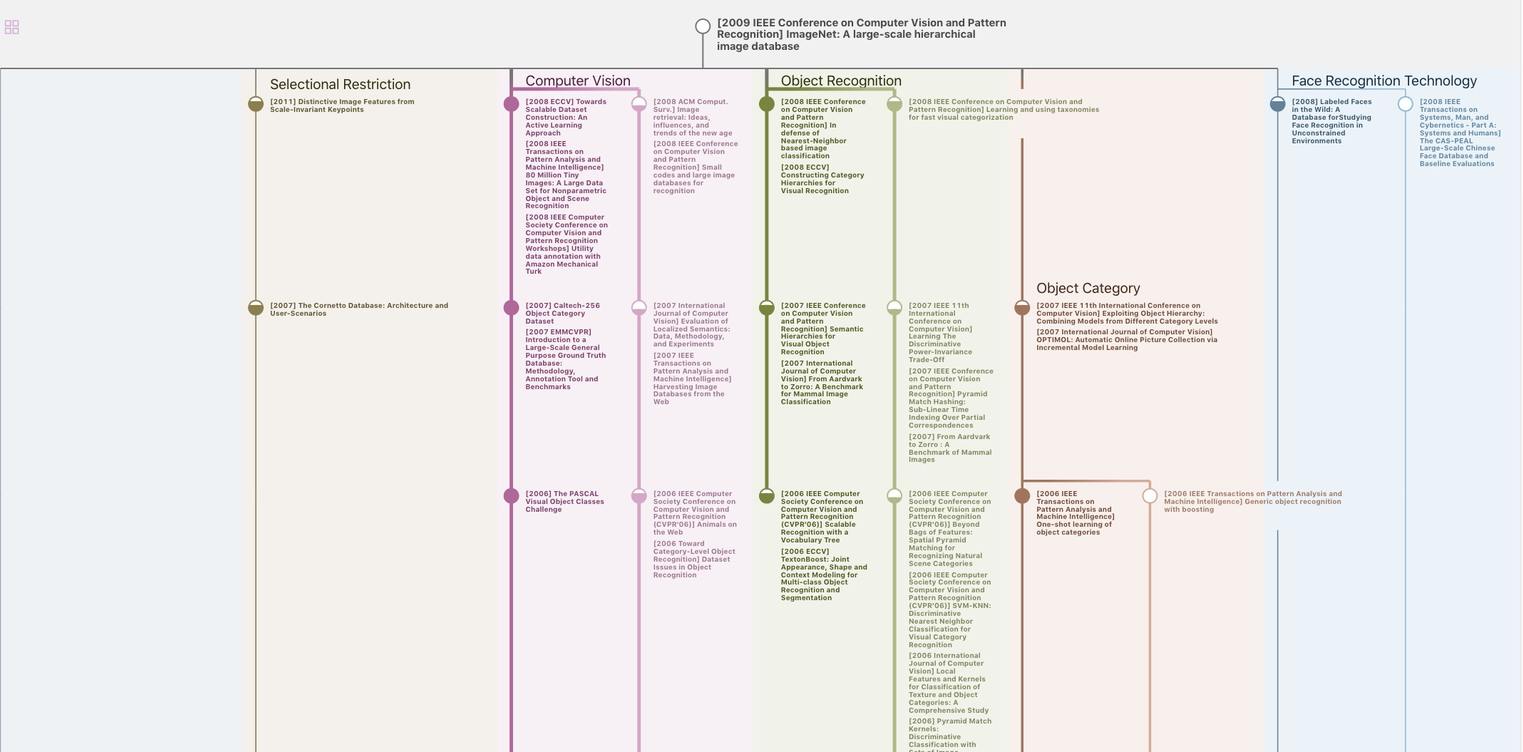
生成溯源树,研究论文发展脉络
Chat Paper
正在生成论文摘要