GATBoost: Mining graph attention networks-based important substructures of polymers for a better property prediction
MATERIALS TODAY COMMUNICATIONS(2024)
摘要
The structure of polymers determines their properties. Within the polymer structure, substructures are often highly correlated with the corresponding properties. Due to the specificity of molecular graphs, graph neural networks (GNN) can be better applied for structure-property prediction of polymers. Many recent experiments have demonstrated that GNN and their variants show powerful potential in the problem of property prediction of polymers. This paper proposed a comprehensive framework GATBoost to model the property prediction of polymers. In this regard, graph attention networks (GAT) were employed to mine the polymer substructures highly correlated with the target properties (glass transition temperature, Tg). Then XGBoost-based supervised learning was utilized for property prediction. It was demonstrated that GATBoost could achieve high-accuracy prediction and greatly improve the prediction efficiency and shorten the experimental time. Furthermore, at the same time, the important substructures of polymers mined from our model can be visualized directly.
更多查看译文
关键词
Graph attention networks,Polymers,Deep learning,Graph neural networks,XGBoost,Structure-property prediction
AI 理解论文
溯源树
样例
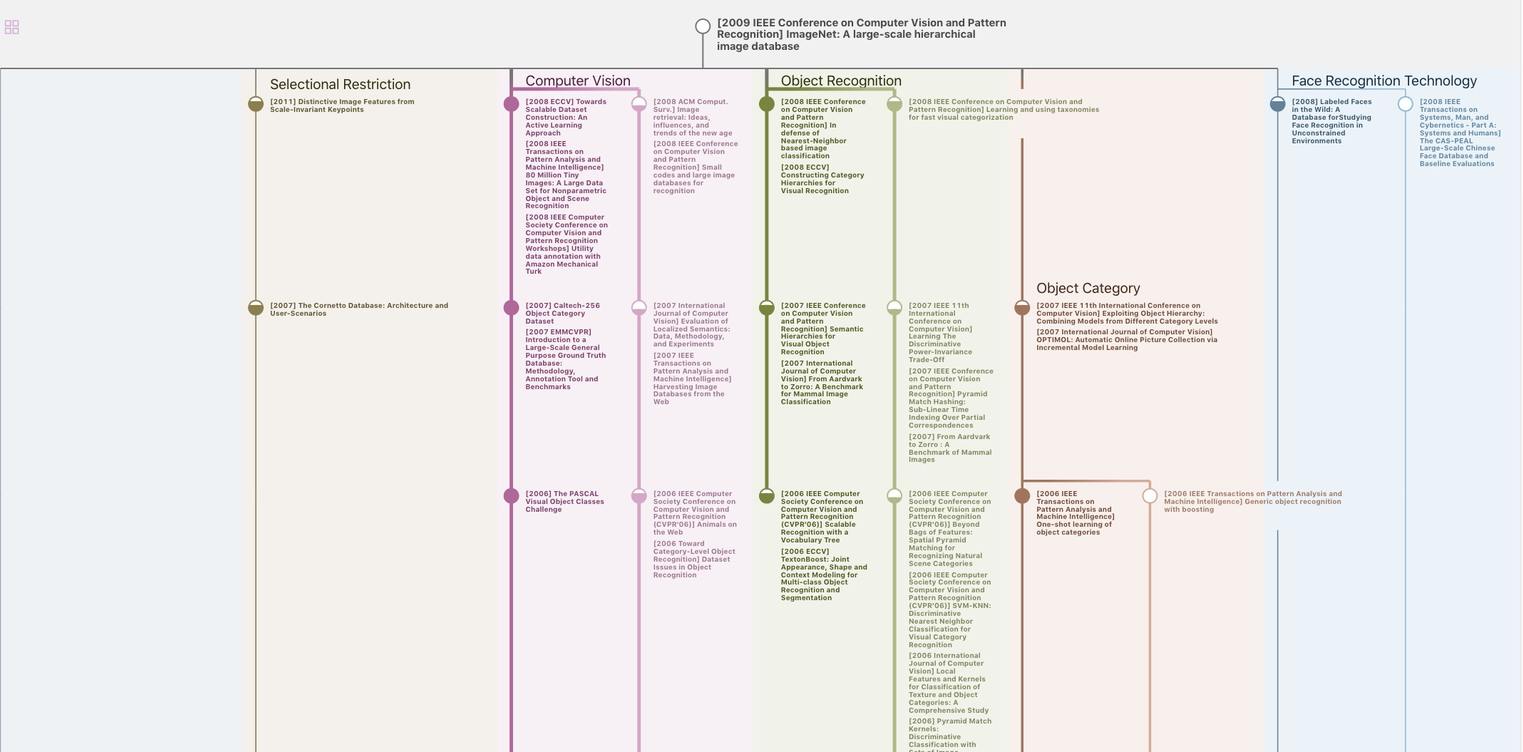
生成溯源树,研究论文发展脉络
Chat Paper
正在生成论文摘要