Hybrid ANFIS-PSO algorithm for estimation of the characteristics of porous vacuum preloaded air bearings and comparison performance of the intelligent algorithm with the ANN
ENGINEERING APPLICATIONS OF ARTIFICIAL INTELLIGENCE(2024)
摘要
Air bearings are commonly used in systems requiring high precision, including semiconductor manufacturing, optical and electronic devices, and aerospace systems, and the usage of vacuum preloading and porous material provides a homogeneous pressure distribution on the bearing surface. The prediction of the characteristics of these air bearings, such as air film thickness and location, is crucial for designing and optimizing the performance of the systems. They exhibit highly nonlinear behavior and are challenging to accurately predict their characteristics using conventional analytical or numerical techniques. In this study, the characteristics of vacuum preloaded porous air bearings are estimated using a hybrid ANFIS-PSO predictor soft computing method with a certain pressure (410 kPa), vacuum (50.8 kPa), and with three different diameters (50, 75 and 90 mm). The usage of different algorithms in the characteristics and selection and design criteria of air bearing has been applied. Six different cases are simulated with different input and output parameters to predict the air film thickness and location of the air bearing. The location of the air bearing has been determined with two angle parameters, alpha, and beta. The dataset required for training and testing the algorithm was obtained through experimental study. In the training and testing process, 40 and 20 datasets were used, respectively. Before the starting analysis, a sensitivity analysis was executed to find optimum PSO parameters such as the number of particles, acceleration coefficients, number of membership functions, and inertia weight. The proposed hybrid ANFIS-PSO method was compared with classical ANFIS and ANN, and it showed better performance and a more accurate solution to estimate the characteristics of vacuum preloaded porous air bearings in both training and testing phases with the R2 of 0.9958 and MSE value of 0.102. The maximum training performance of the ANFIS-PSO is better than ANFIS and ANN with the ratio of 6.8% and 8.5%, respectively. On the other hand, the maximum testing performance of the ANFIS-PSO algorithm is higher than ANFIS and ANN, with the ratio of 22.2% and 24.1%, respectively.
更多查看译文
关键词
Air bearing,Vacuum preloaded,Porous,Adaptive neural -fuzzy inference systems,Artificial neural network,Particle swarm optimization
AI 理解论文
溯源树
样例
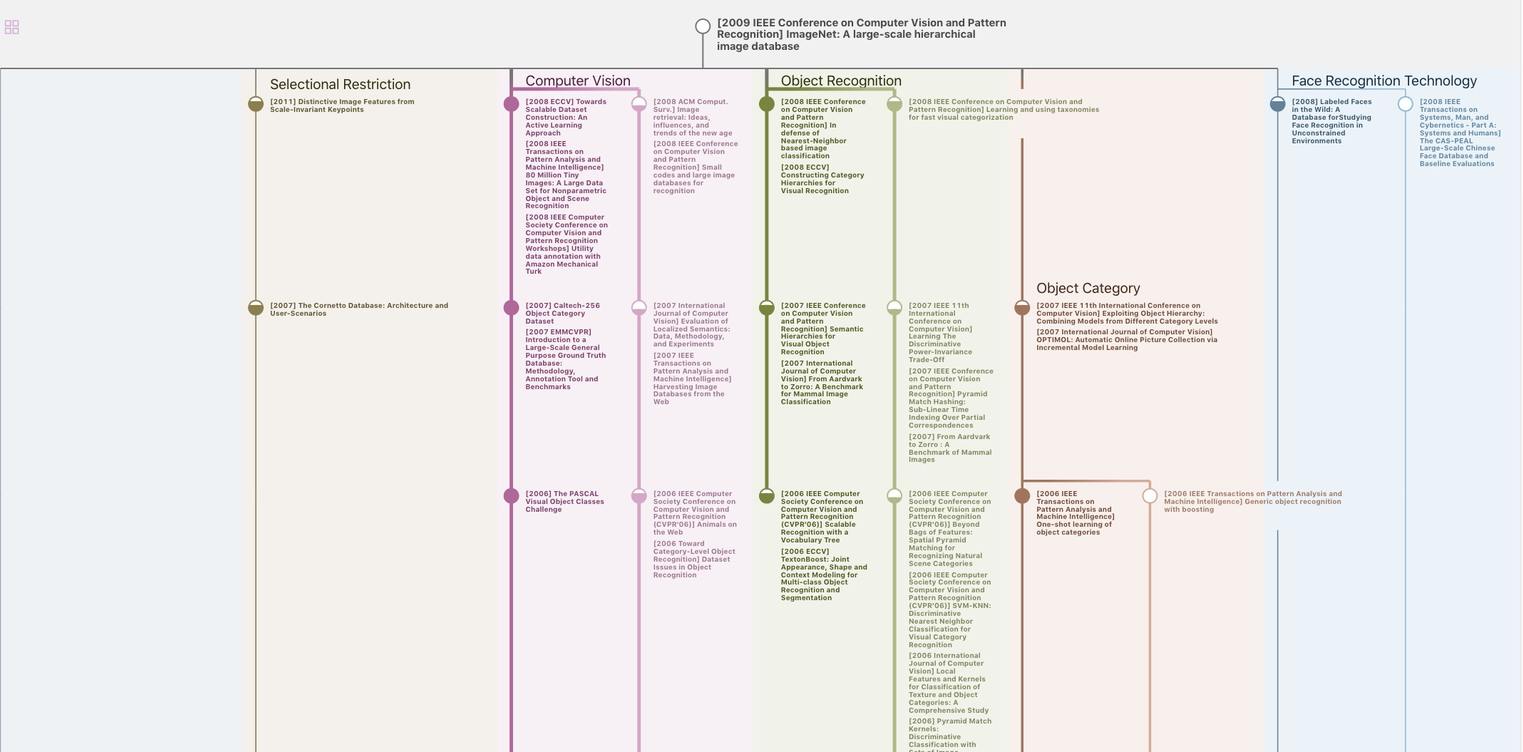
生成溯源树,研究论文发展脉络
Chat Paper
正在生成论文摘要