Efficient low-light image enhancement with model parameters scaled down to 0.02M
International Journal of Machine Learning and Cybernetics(2023)
摘要
In the field of low-light image enhancement, existing deep learning methods face three significant challenges: inaccurate reflection component estimation, poor image enhancement capabilities, and high computational costs. This study introduces a novel, efficient solution to these problems in the form of an Ultra-Lightweight Enhancement Network (ULENet). Our primary contributions are twofold. First, we propose the combination of channel-wise context mining and spatial-wise reinforcement for improved low-light image enhancement. Second, we introduce a novel lightweight neural architecture, ULENet, designed specifically for this purpose. ULENet features two innovative subnetworks: the channel-wise context mining subnetwork for extracting rich context from low-light images, and the spatial-wise reinforcement subnetwork for extensive spatial feature extraction and detail reconstruction. We use the deep-learning framework PyTorch for training and evaluating our model. Extensive experiments demonstrate that ULENet significantly outperforms nine state-of-the-art low-light enhancement methods in terms of speed, accuracy, and adaptability in complex low-light scenarios. These results validate our initial hypothesis and underscore the effectiveness of the proposed approach.
更多查看译文
关键词
Low-light image enhancement,ULENet,Neural architecture,Channel-wise context mining,Spatial-wise feature reinforcement,Deep learning,Lightweight model,Image processing
AI 理解论文
溯源树
样例
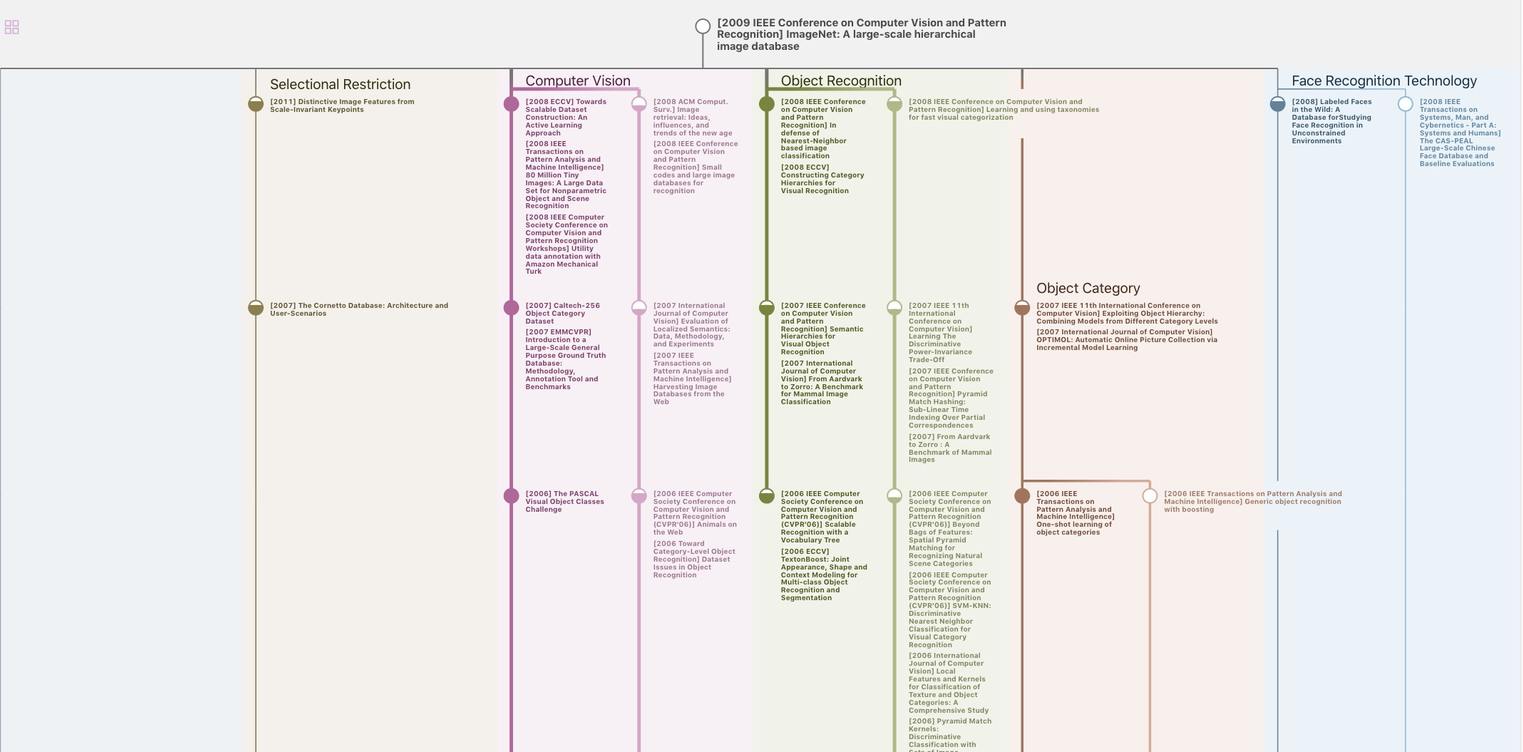
生成溯源树,研究论文发展脉络
Chat Paper
正在生成论文摘要