Machine learning prediction of 3CL
Computational Biology and Chemistry(2022)
摘要
Molecular docking results of two training sets containing 866 and 8,696 compounds were used to train three different machine learning (ML) approaches. Neural network approaches according to Keras and TensorFlow libraries and the gradient boosted decision trees approach of XGBoost were used with DScribe’s Smooth Overlap of Atomic Positions molecular descriptors. In addition, neural networks using the SchNetPack library and descriptors were used. The ML performance was tested on three different sets, including compounds for future organic synthesis. The final evaluation of the ML predicted docking scores was based on the ZINC in vivo set, from which 1,200 compounds were randomly selected with respect to their size. The results obtained showed a consistent ML prediction capability of docking scores, and even though compounds with more than 60 atoms were found slightly overestimated they remain valid for a subsequent evaluation of their drug repurposing suitability. Display Omitted • AutoDock docking scores of 12,000 compounds are obtained for 3CLpro (6WQF). • Machine learning (ML) is based on TensorFlow, XGBoost and SchNetPack libraries. • DScribe and SchNet molecular descriptors use xyz/mol2 file formats. • Predictions of docking scores tend to be overestimated for large compounds. • Further improvement of ML models is possible for better tuned train sets.
更多查看译文
关键词
AutoDock molecular docking,3CLpro Mpro 6WQF,Machine learning,TensorFlow XGBoost SchNetPack,COVID19,SARS-CoV-2
AI 理解论文
溯源树
样例
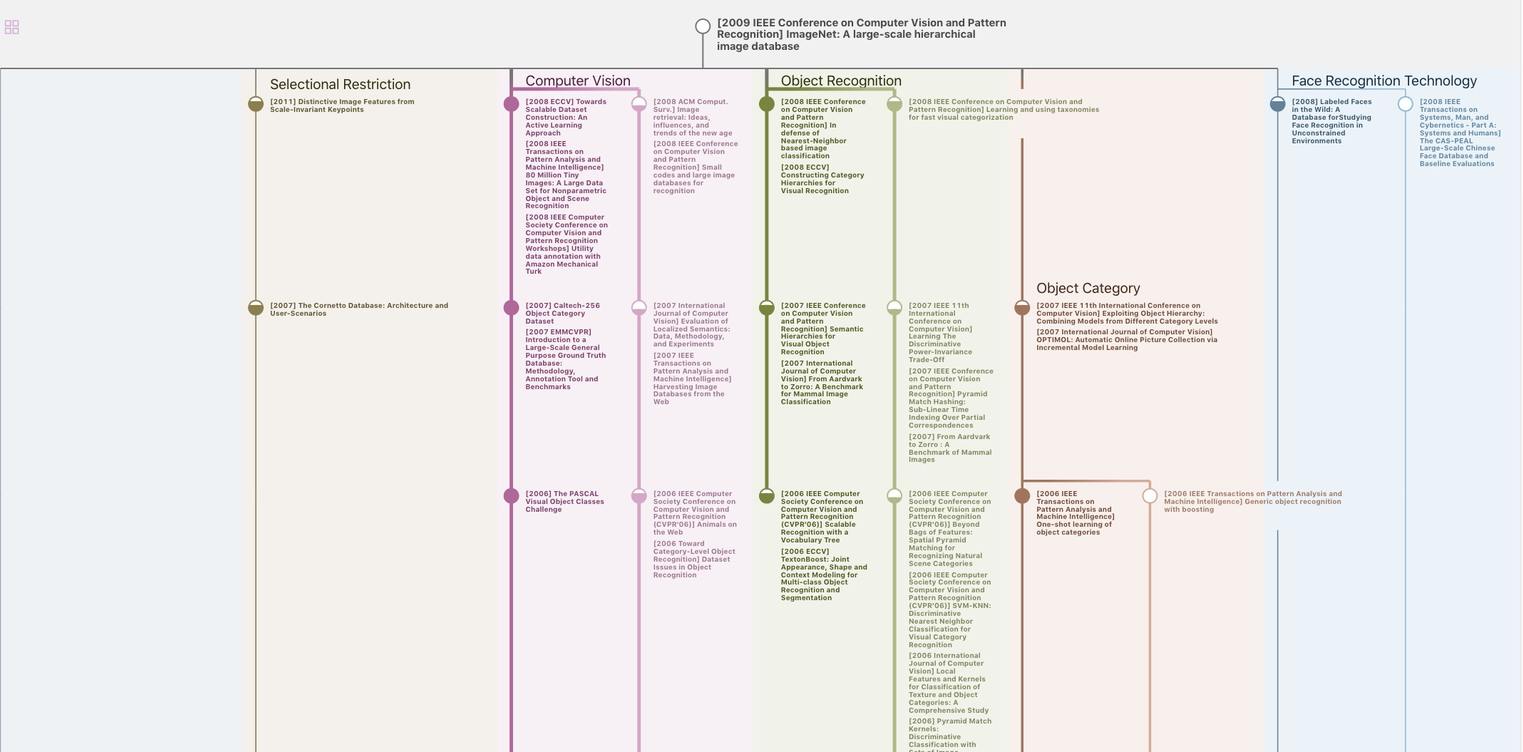
生成溯源树,研究论文发展脉络
Chat Paper
正在生成论文摘要