Generative De-Quantization for Neural Speech Codec via Latent Diffusion
arXiv (Cornell University)(2023)
Abstract
In low-bitrate speech coding, end-to-end speech coding networks aim to learn\ncompact yet expressive features and a powerful decoder in a single network. A\nchallenging problem as such results in unwelcome complexity increase and\ninferior speech quality. In this paper, we propose to separate the\nrepresentation learning and information reconstruction tasks. We leverage an\nend-to-end codec for learning low-dimensional discrete tokens and employ a\nlatent diffusion model to de-quantize coded features into a high-dimensional\ncontinuous space, relieving the decoder's burden of de-quantizing and\nupsampling. To mitigate the issue of over-smooth generation, we introduce\nmidway-infilling with less noise reduction and stronger conditioning. In\nablation studies, we investigate the hyperparameters for midway-infilling and\nlatent diffusion space with different dimensions. Subjective listening tests\nshow that our model outperforms the state-of-the-art at two low bitrates, 1.5\nand 3 kbps. Codes and samples of this work are available on our webpage.
MoreTranslated text
Key words
Speech Codec,Latent Diffusion Model,Speech Synthesis
AI Read Science
Must-Reading Tree
Example
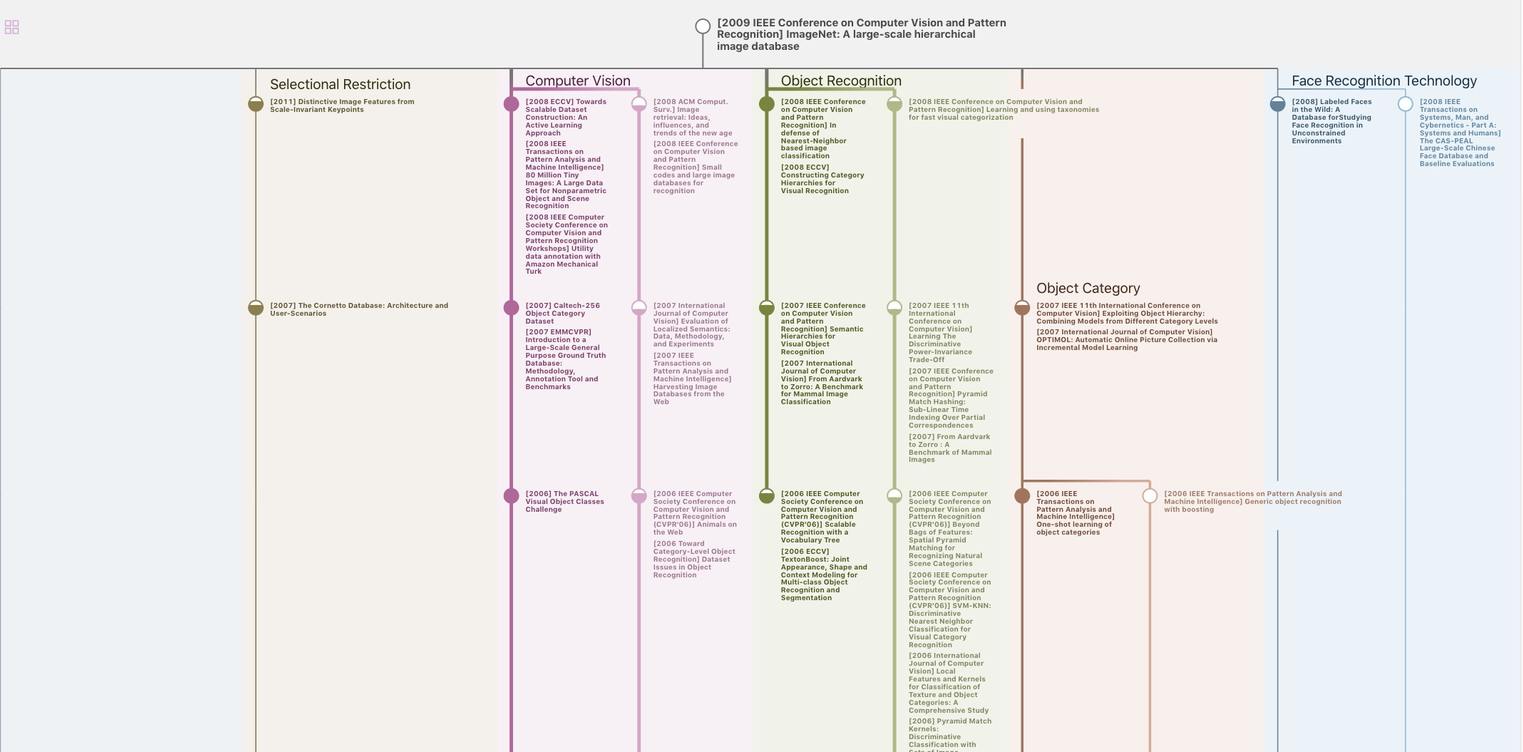
Generate MRT to find the research sequence of this paper
Chat Paper
Summary is being generated by the instructions you defined