Robust Multitask Learning With Sample Gradient Similarity
IEEE TRANSACTIONS ON SYSTEMS MAN CYBERNETICS-SYSTEMS(2024)
摘要
Multitask learning has led to great success in many deep learning applications during the last decade. However, recent experiments have demonstrated that the performance of multitask learning depends on how to balance the relationship between different tasks. Therefore, many approaches have been proposed to adjust per-task gradient directions or design a more appropriate task reweighting scheme based on task-level statistics. In this article, we discuss how to boost the performance of multitask learning by using more fine-grained sample gradient information. To this end, we propose the concept of sample gradient similarity, which measures the agreement between the sample gradient for a task and the true gradient. Based on this concept, greater weight is assigned to more consistent tasks and more robust training samples to improve the training process of multitask learning. Extensive experimental results show that our proposed method outperforms the state-of-the-art algorithms on a series of challenging multitask datasets.
更多查看译文
关键词
Deep learning,Automation,multitask learning,sample gradient,sample reweighting,task reweighting
AI 理解论文
溯源树
样例
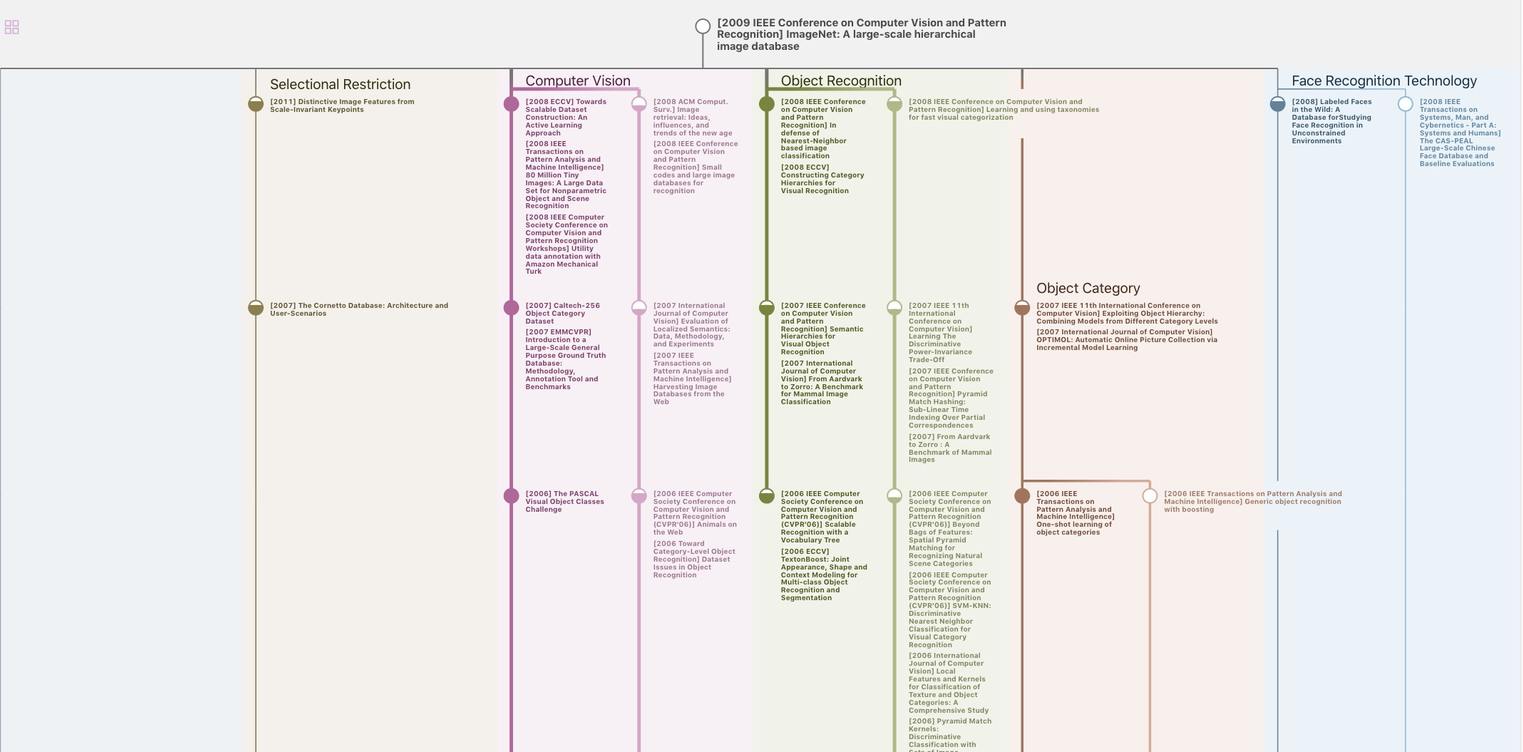
生成溯源树,研究论文发展脉络
Chat Paper
正在生成论文摘要