Prediction of Molecular Conformation Using Deep Generative Neural Networks
CHINESE JOURNAL OF CHEMISTRY(2023)
摘要
The accurate prediction of molecular conformations with high efficiency is crucial in various fields such as materials science, computational chemistry and computer-aided drug design, as the three-dimensional structures accessible at a given condition usually determine the specific physical, chemical, and biological properties of a molecule. Traditional approaches for the conformational sampling of molecules such as molecular dynamics simulations and Markov chain Monte Carlo methods either require an exponentially increasing amount of time as the degree of freedom of the molecule increases or suffer from systematic errors that fail to obtain important conformations, thus presenting significant challenges for conformation sampling with both high efficiency and high accuracy. Recently, deep learning-based generative models have emerged as a promising solution to this problem. These models can generate a large number of molecular conformations in a short time, and their accuracy is comparable and, in some cases, even better than that of current popular open-source and commercial software. This Emerging Topic introduces the recent progresses of using deep learning for predicting molecular conformations and briefly discusses the potential and challenges of this emerging field. Molecular conformations play a crucial role in fields such as materials science and drug design. Traditional methods like molecular dynamics and Monte Carlo simulations are limited in speed and accuracy. Deep learning models offer a promising solution by rapidly generating accurate molecular conformations. This Emerging Topic highlights recent progresses in using deep learning for predicting molecular conformations and explores the potential and challenges of this emerging field.image
更多查看译文
关键词
Conformation generation,Machine learning,Deep Generative Neural Networks,Drug design,Reaction mechanisms
AI 理解论文
溯源树
样例
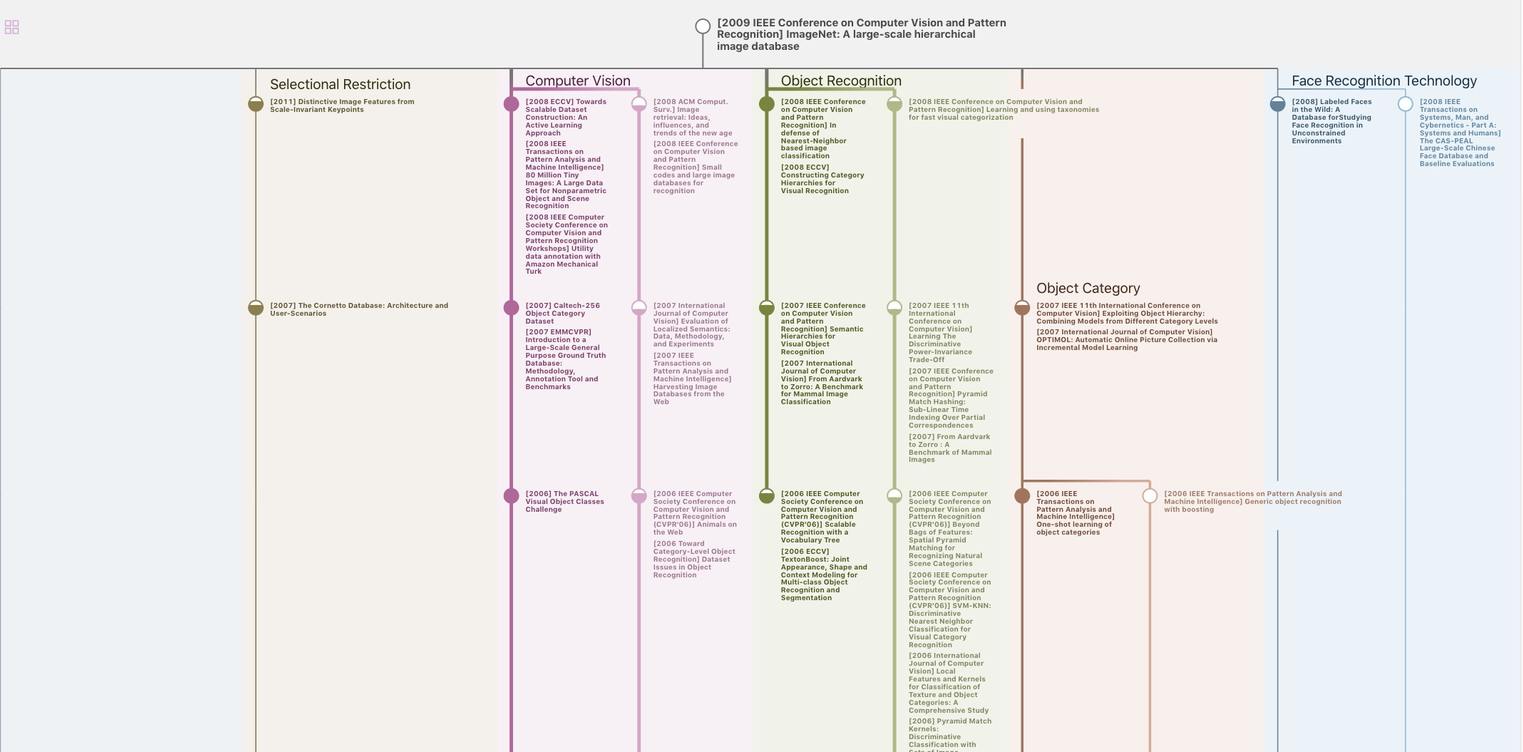
生成溯源树,研究论文发展脉络
Chat Paper
正在生成论文摘要