The Semisupervised Weighted Centroid Prototype Network for Fault Diagnosis of Wind Turbine Gearbox
IEEE-ASME TRANSACTIONS ON MECHATRONICS(2024)
摘要
The success of fault diagnosis based on deep learning benefits from a large amount of labeled fault samples. However, the scarcity of labeled fault samples in fault diagnosis of wind turbine gearbox (WTG) makes it difficult to train a satisfactory diagnostic model. To address this issue, this article proposed a semisupervised weighted centroid prototype network (SSWCPN) for WTG fault diagnosis. Specifically, SSWCPN is a few-shot semisupervised learning framework, which alleviates the matter of overfitting caused by the lack of supervision information. First, to capture abundant semisupervised information to guide network training, a sample selection model based on the evolution trend of posterior probability is proposed, which could efficiently cherry-pick out the unlabeled samples of high confidence to refine prototypes. Second, a new prototype updating strategy based on a weighted centroid prototype is designed, which controls the prototype drifting issue caused by incorrect pseudolabels and the introduction of new data distribution. Finally, experiments performed on test-bench data and successful application on WTG data show that the proposed SSWCPN-based WTG fault diagnosis achieves the best fault diagnosis performance among the comparison methods.
更多查看译文
关键词
Fault diagnosis,few-shot learning (FSL),prototype network,semisupervised learning,wind turbine gearbox (WTG)
AI 理解论文
溯源树
样例
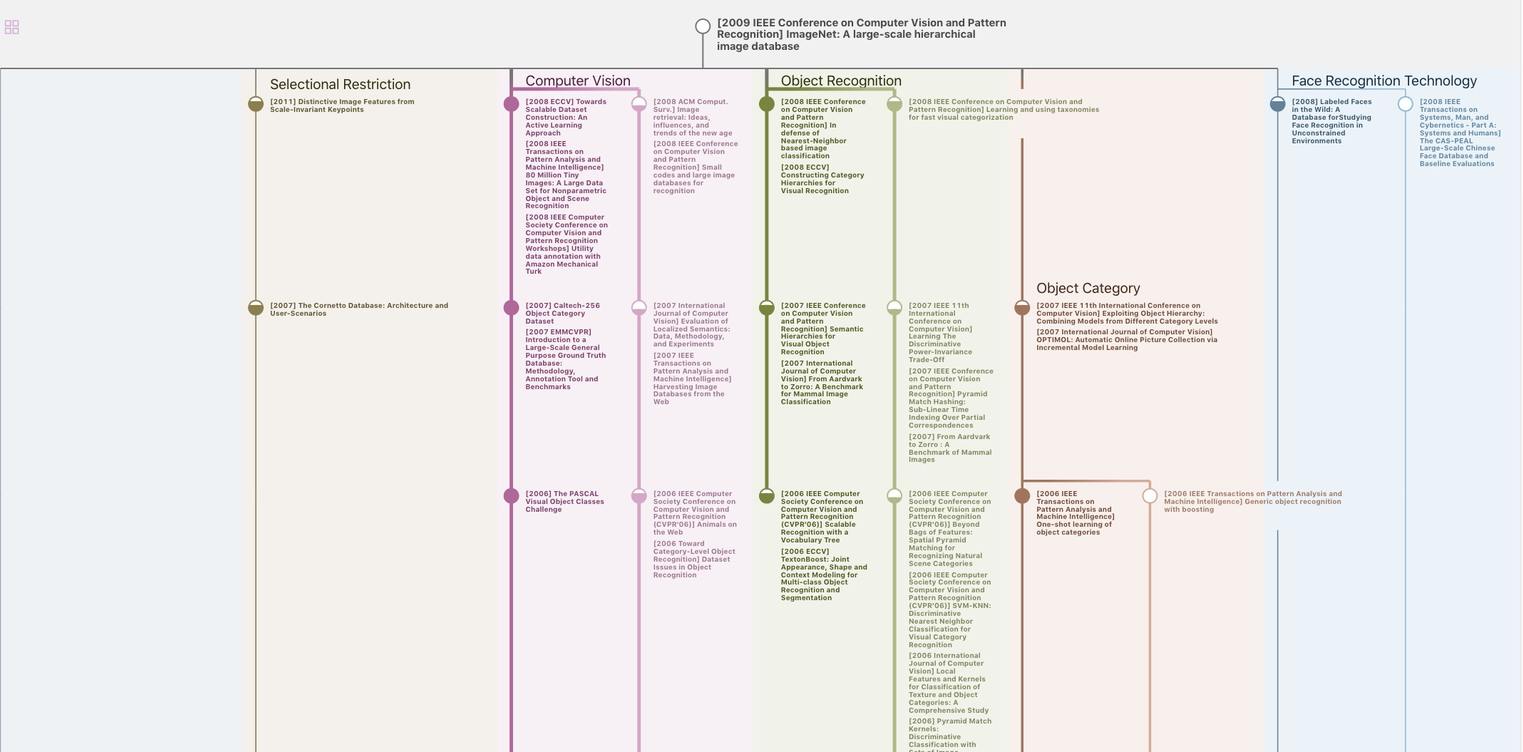
生成溯源树,研究论文发展脉络
Chat Paper
正在生成论文摘要