Research on predicting compressive strength of magnesium silicate hydrate cement based on machine learning
CONSTRUCTION AND BUILDING MATERIALS(2023)
摘要
In this study, a machine learning approach was employed to establish a predictive model for the compressive strength of magnesium silicate hydrate cement (MSHC). Sensitivity analysis of the input parameters was conducted using Shapley Additive Explanations (SHAP). The results indicated that the Gradient Boosting Regression (GBR) and Extreme Gradient Boosting (XGB) models exhibited enhanced precision in forecasting the compressive strength of MSHC, with the XGB model showcasing superior prowess in generalization. Among the various feature parameters, the curing age (Age) was identified as the most significant factors influencing the compressive strength of MSHC. The compressive strength of MSHC was positively correlated with the content of Age, MgO (C-Mg), and SiO2 (C-Si), and negatively correlated with the water-cement ratio (W/C). It also had a nonlinear relationship with parameters such as the specific surface area of MgO (S-Mg) and the Mg/Si ratio. Finally, the optimal parameters for preparing MSHC were proposed, including an optimal S-Mg value of approximately 22 m2/g and an optimal Mg/Si ratio of 1:1. When potassium dihydrogen phosphate (K) was used as a dispersant, the optimal dosage was around 4.5%. On the other hand, when sodium hexametaphosphate (S) was used as a dispersant, a dosage greater than 2.5% resulted in a certain improvement in strength.
更多查看译文
关键词
Magnesium silicate hydrate cement,Machine learning,Feature parameters,Strength prediction
AI 理解论文
溯源树
样例
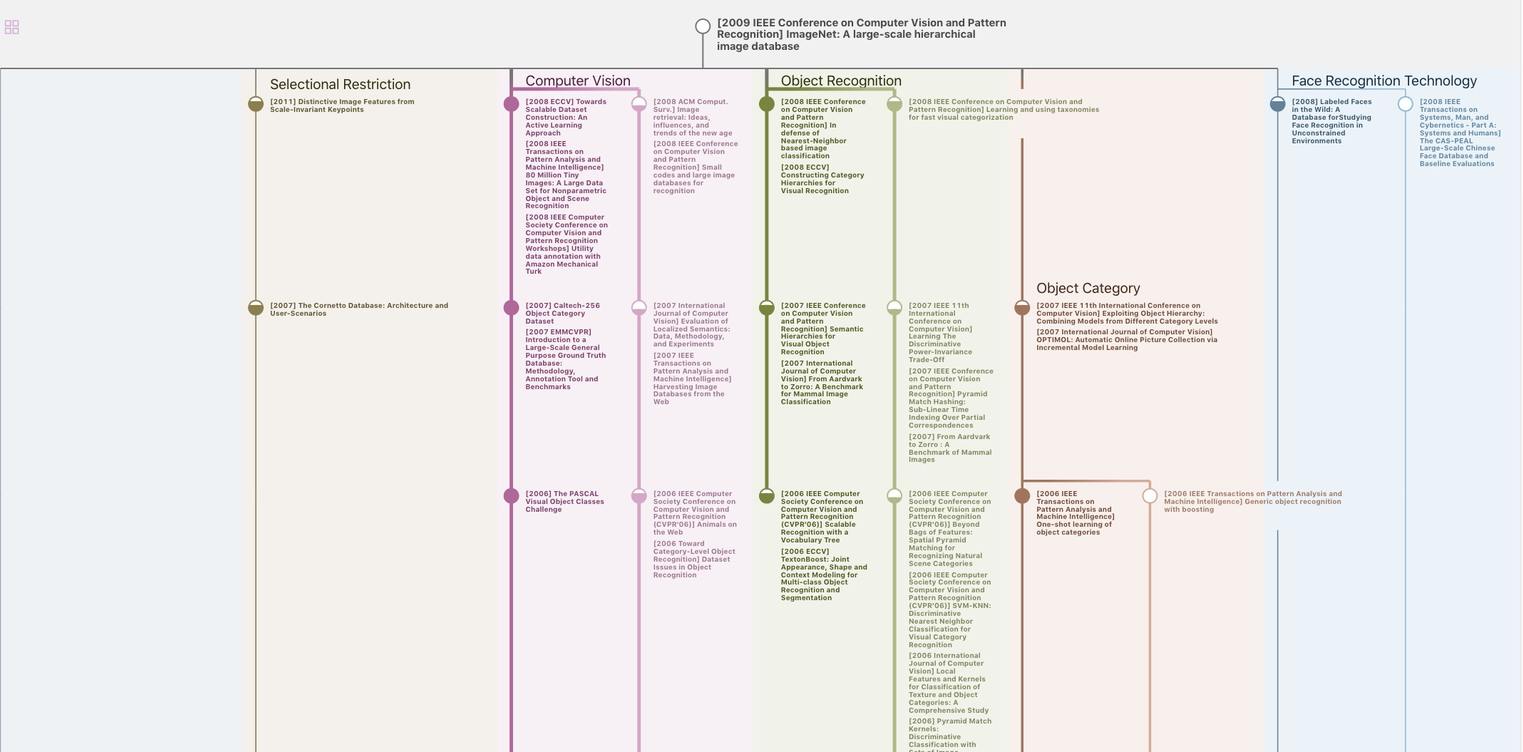
生成溯源树,研究论文发展脉络
Chat Paper
正在生成论文摘要