Data Factors for Better Compositional Generalization
Proceedings of the 2023 Conference on Empirical Methods in Natural Language Processing(2023)
关键词
Topic Modeling
AI 理解论文
溯源树
样例
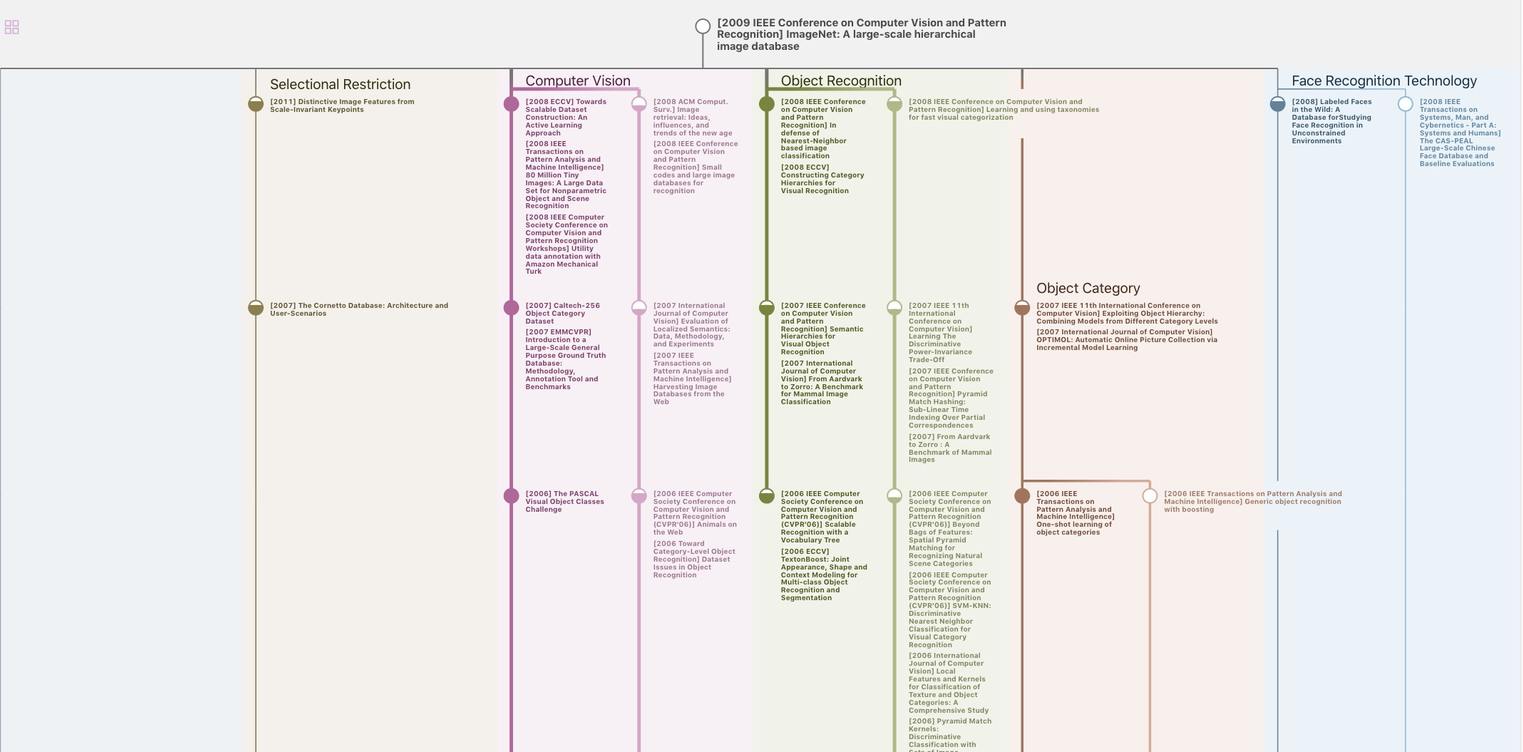
生成溯源树,研究论文发展脉络
Chat Paper
正在生成论文摘要
Proceedings of the 2023 Conference on Empirical Methods in Natural Language Processing(2023)