Anisotropic 6-D Rigid Point Set Registration.
IEEE Trans. Instrum. Meas.(2023)
摘要
Registration of two-point sets (PSs) is an essential problem in research areas of robotics, computer vision, measurements, and computer-assisted interventions (CAIs). Registration, however, is a challenging problem essentially because PSs in real-world scenarios contain noise and outliers. Features such as normal vectors, which can be extracted from raw PSs, have the potential to improve both registration accuracy and robustness. However, like positional vectors, the extracted normal vectors have localization errors as well. This article explicitly considers the anisotropic error distributions with both positional and normal vectors in formulating and solving the PS registration problem. To achieve this aim, the multivariate Gaussian distribution and the Kent distribution are utilized to model the positional and normal error vectors, respectively. With the probabilistic models, the registration task is cast as a maximum likelihood estimation (MLE) problem where the latent variables are point correspondences between two PSs. The expectation maximization (EM) technique is leveraged to solve the above-formulated optimization problem. Experimental results on the human femur and pelvis PSs demonstrate that the proposed method has the best registration accuracy and robustness among all compared state-of-the-art (SOTA) methods. Results show that the proposed method can achieve subdegree rotational error and submilimeter translational error values, under noise and a wide range of outliers.
更多查看译文
关键词
Expectation maximization (EM),generalized point set (PS) registration,Kent distribution,maximum likelihood estimation (MLE),surgical navigation
AI 理解论文
溯源树
样例
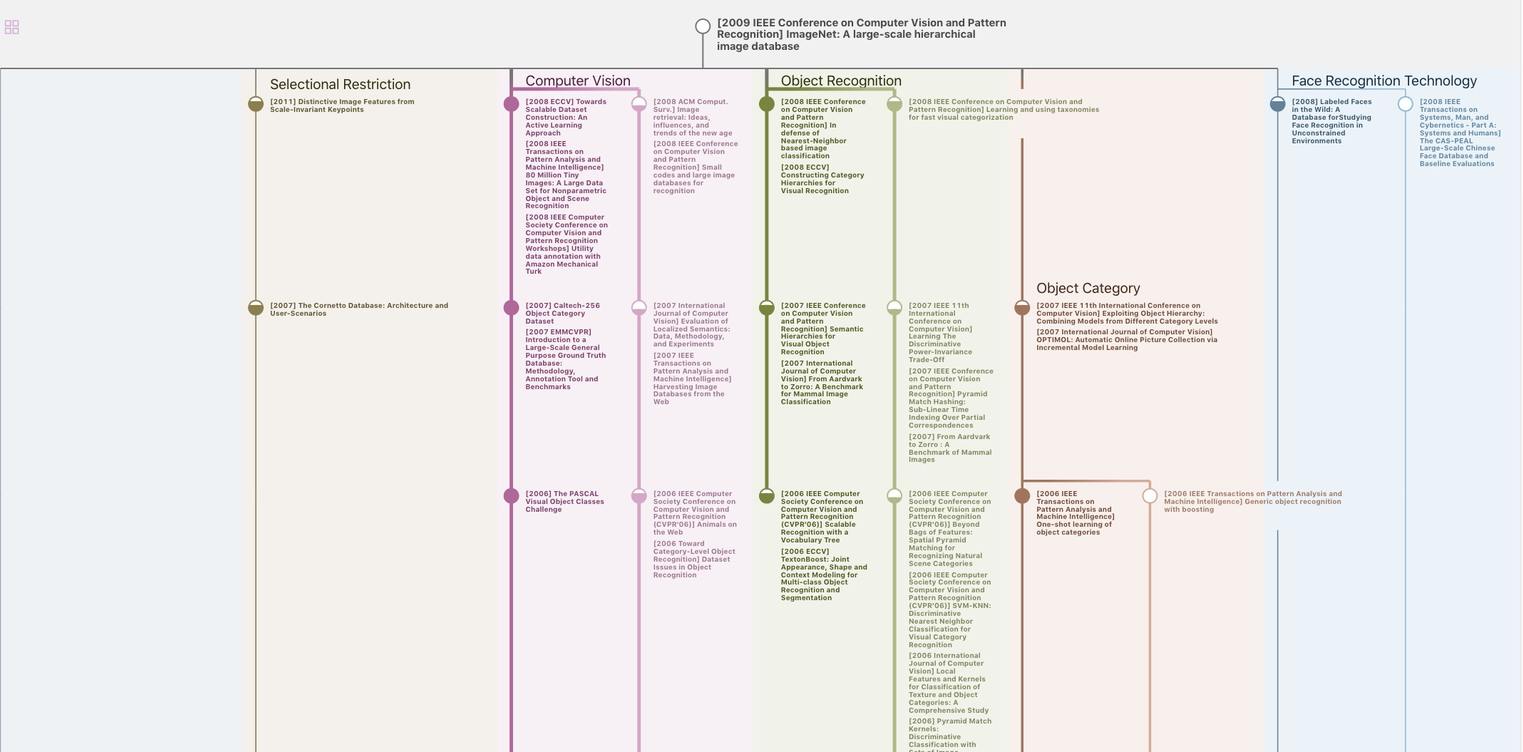
生成溯源树,研究论文发展脉络
Chat Paper
正在生成论文摘要