MSDformer: Multiscale Deformable Transformer for Hyperspectral Image Super-Resolution
IEEE transactions on geoscience and remote sensing(2023)
摘要
Deep learning-based hyperspectral image super-resolution (SR) methods have achieved remarkable success, which can improve the spatial resolution of hyperspectral images with abundant spectral information. However, most of them utilize 2-D or 3-D convolutions to extract local features while ignoring the rich global spatial-spectral information. In this article, we propose a novel method called the Multiscale Deformable Transformer (MSDformer) for single hyperspectral image SR (SHSR). The proposed method incorporates the strengths of the convolutional neural network (CNN) for local spatial-spectral information and the Transformer structure for global spatial-spectral information. Specifically, a multiscale spectral attention module (MSAM) based on dilated convolution is designed to extract local multiscale spatial-spectral information, which leverages shared module parameters to exploit the intrinsic spatial redundancy and spectral attention mechanism to accentuate the subtle differences between different spectral groups. Then a deformable convolution-based Transformer module (DCTM) is proposed to further extract the global spatial-spectral information from the local multiscale features of the previous stage, which can explore the diverse long-range dependencies among all spectral bands. Extensive experiments on three hyperspectral datasets demonstrate that the proposed method achieves excellent SR performance and outperforms the state-of-the-art methods in terms of quantitative quality and visual results. The code is available at https://github.com/Tomchenshi/MSDformer.git.
更多查看译文
关键词
Convolutional neural network (CNN),hyperspectral image,super-resolution (SR),Transformer
AI 理解论文
溯源树
样例
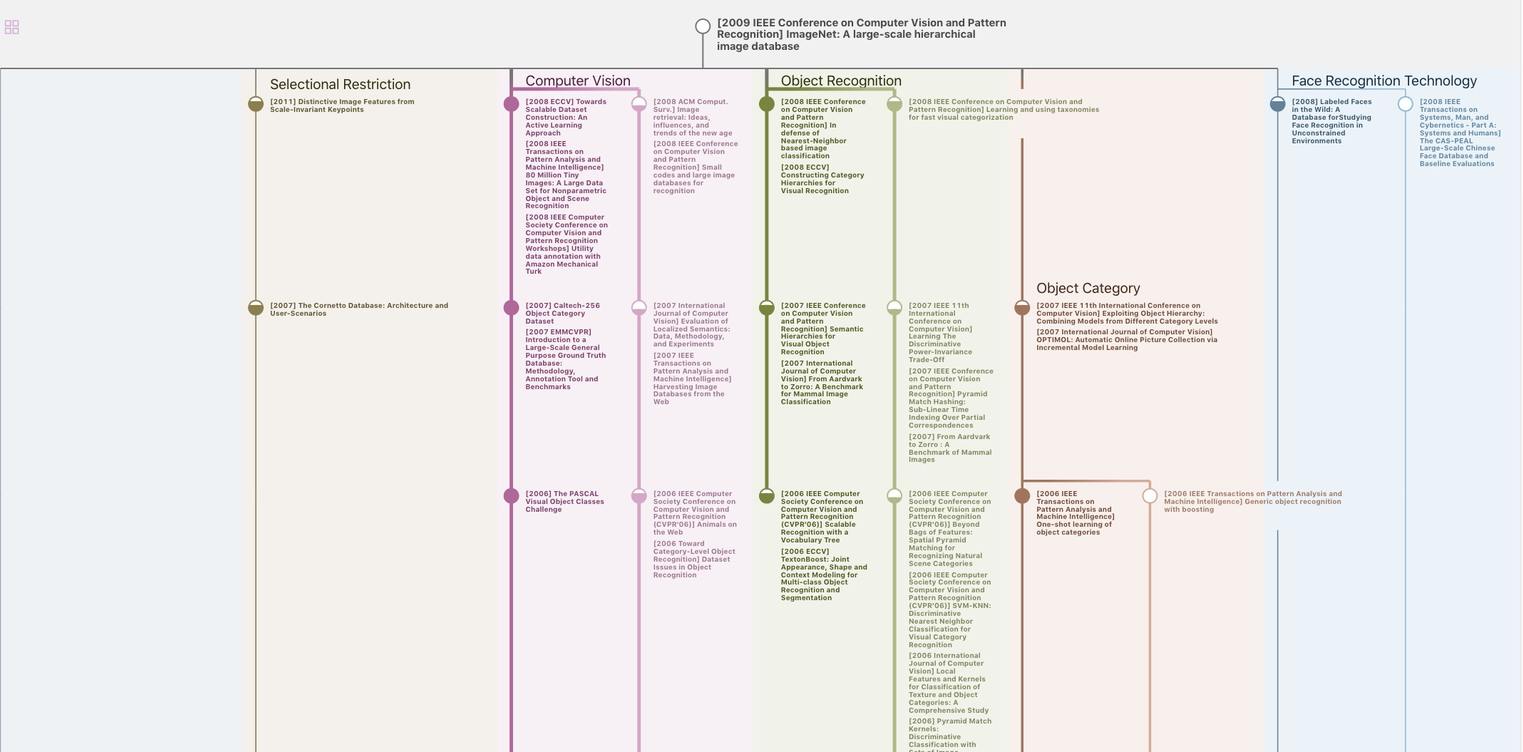
生成溯源树,研究论文发展脉络
Chat Paper
正在生成论文摘要