Multi-scale Conformer Fusion Network for Multi-participant Behavior Analysis
MM '23: Proceedings of the 31st ACM International Conference on Multimedia(2023)
Abstract
Understanding and elucidating human behavior across diverse scenarios represents a pivotal research challenge in pursuing seamless human-computer interaction. However, previous research on multi-participant dialogues has mostly relied on proprietary datasets, which are not standardized and openly accessible. To propel advancements in this domain, the MultiMediate'23 Challenge presents two sub-challenges: Eye contact detection and Next speaker prediction, aiming to foster a comprehensive understanding of multi-participant behavior. To tackle these challenges, we propose a multi-scale conformer fusion network (MSCFN) for enhancing the perception of multi-participant group behaviors. The conformer block combines the strengths of transformers and convolution networks to facilitate the establishment of global and local contextual relationships between sequences. Then the output features from all Conformer blocks are concatenated to fusion multi-scale representations. Our proposed method was evaluated using the officially provided dataset, and it achieves the best and second best performance in next speaker prediction and gaze detection tasks of MultiMediate'23, respectively.
MoreTranslated text
AI Read Science
Must-Reading Tree
Example
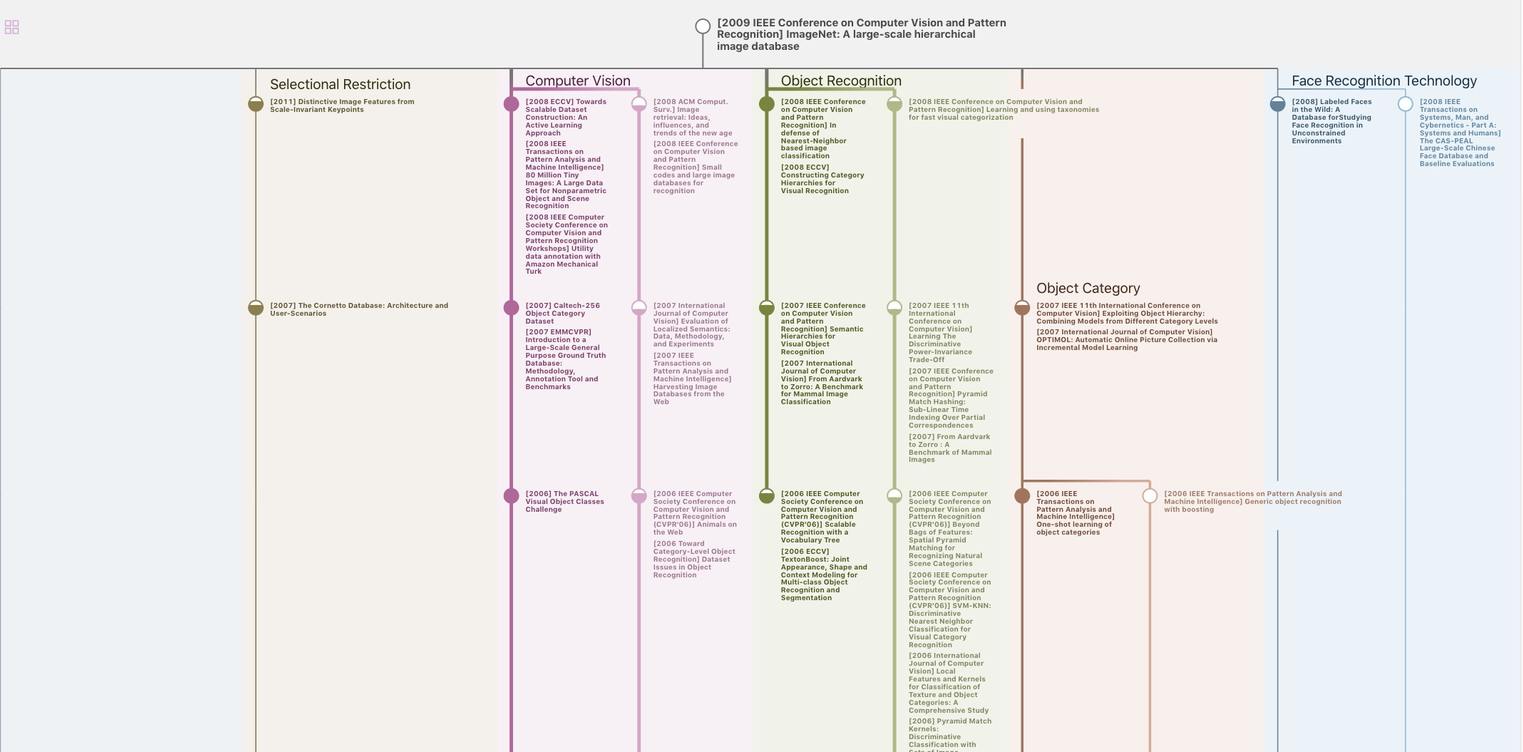
Generate MRT to find the research sequence of this paper
Chat Paper
Summary is being generated by the instructions you defined