Robust Interplanetary Trajectory Design under Multiple Uncertainties Via Meta-Reinforcement Learning
Acta astronautica(2024)
摘要
This paper focuses on the application of meta-reinforcement learning to the robust design of low-thrust interplanetary trajectories in the presence of multiple uncertainties. A closed-loop control policy is used to optimally steer the spacecraft to a final target state despite the considered perturbations. The control policy is approximated by a deep recurrent neural network, trained by policy-gradient reinforcement learning on a collection of environments featuring mixed sources of uncertainty, namely dynamic uncertainty and control execution errors. The recurrent network is able to build an internal representation of the distribution of environments, thus better adapting the control to the different stochastic scenarios. The results in terms of optimality, constraint handling, and robustness on a fuel-optimal low-thrust transfer between Earth and Mars are compared with those obtained via a traditional reinforcement learning approach based on a feed-forward neural network.
更多查看译文
关键词
Meta-reinforcement learning,Robust trajectory design,Closed-loop guidance,Recurrent neural network,Proximal policy optimization,Stochastic optimal control
AI 理解论文
溯源树
样例
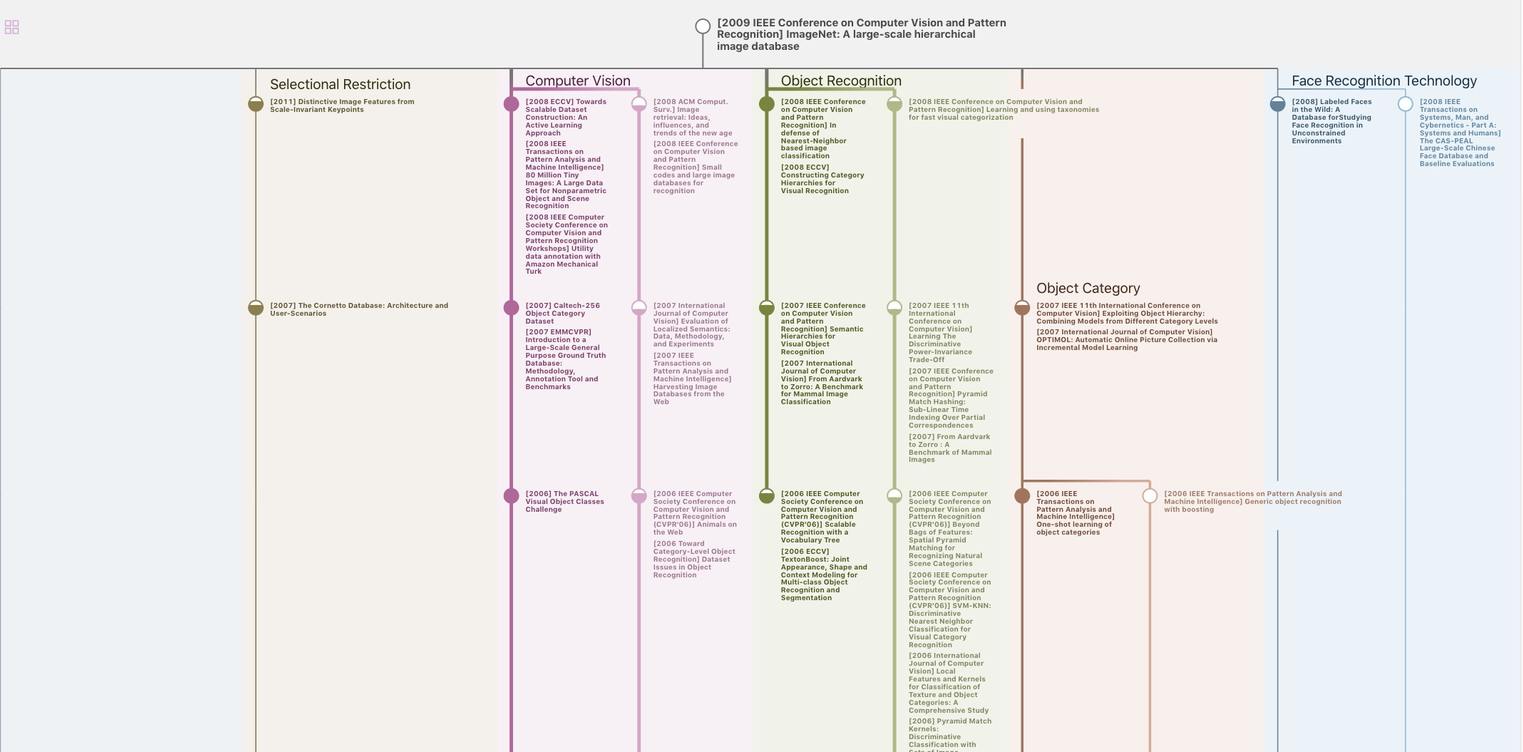
生成溯源树,研究论文发展脉络
Chat Paper
正在生成论文摘要