Advanced transformer model for simultaneous leakage aperture recognition and localization in gas pipelines
RELIABILITY ENGINEERING & SYSTEM SAFETY(2024)
摘要
This study proposes a joint learning end-to-end dual-stream transformer structure based on enhanced external attention and improved feedforward mechanisms (EEA-JL) to address the problem of simultaneous leakage aperture recognition and localization in gas pipelines under noisy backgrounds. The EEA-JL structure can effectively improve the accuracy of leakage aperture recognition and localization in a single model. Traditional convolutional neural networks (CNNs) and recursive frameworks (RNNs) have limitations in extracting leakage features and analyzing positional information, making it difficult to capture the correlation coupling between different leakage apertures and positions. The EEA-JL structure incorporates a self-attention mechanism with two external linear neural memory units to analyze the correlation between samples and deepen the understanding of different leakage scenarios. Additionally, the multi-scale soft-threshold denoising module (MSSD) adaptively estimates the noise threshold of signals under different leakage conditions to achieve denoising. Through simulation experiments on a 169-meter oil and gas pipeline leakage detection system platform and comparison with other advanced methods, the EEA-JL model achieves a precision rate and R2score of 99.7% and 0.993, respectively, in aperture recognition and localization, with an average positioning error controlled within 1.26 m, demonstrating its guiding significance.
更多查看译文
关键词
Localization,Leak aperture recognition,Transformer,Dual-stream network,Joint learning
AI 理解论文
溯源树
样例
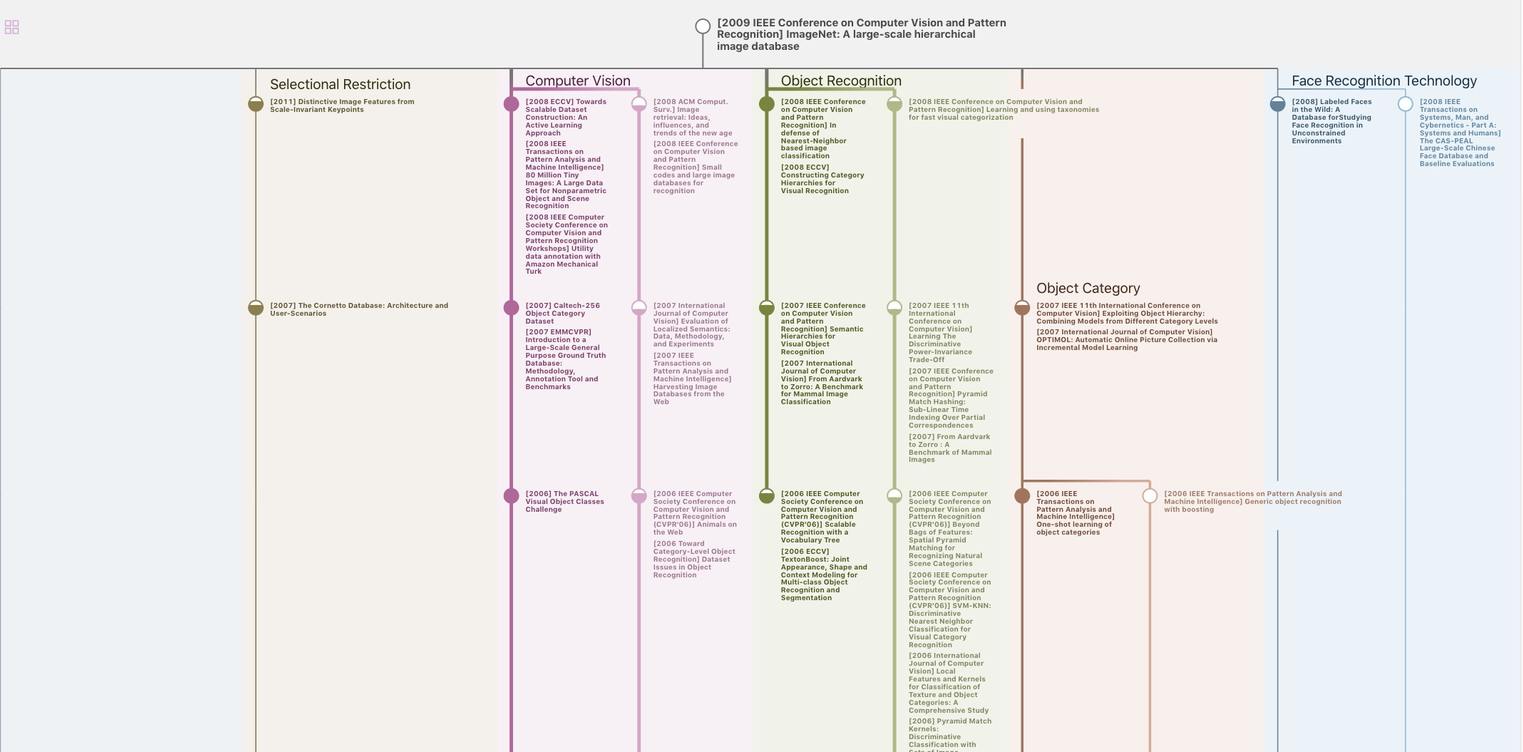
生成溯源树,研究论文发展脉络
Chat Paper
正在生成论文摘要