Early Symptom Detection of Basal Stem Rot Disease in Oil Palm Trees Using a Deep Learning Approach on UAV Images.
Computers and electronics in agriculture(2023)
摘要
The oil palm tree (Elaeis guineensis Jacq.) is a vital and economically important plant, as the oil from its fruit is a primary export for many Southeast Asian countries. Hence the health of these trees is directly linked to their yield and the income of the growers. Unfortunately, basal stem rot (BSR) disease has been identified as the main threat to oil palm trees, causing infected trees to reduce their production and ultimately leading to death. While prior research has shown high accuracy in detecting oil palm trees, there has been limited focus on automating the observation of individual tree health status, particularly in identifying early symptoms of BSR disease within densely populated regions. This study introduces an enhanced approach for early symptom detection of BSR disease in densely populated oil palm tree areas. We present a modified U-Net architecture called the Multi -Convolution Residual (M-CR) U-Net, coupled with an image postprocessing method known as the overlapped contour separation (OVCS) algorithm. The M-CR U-Net differs from the original U-Net as it leverages on multiple convolution kernels of various sizes while incorporating skip connections. This design improvement results in superior segmentation performance compared to approaches utilizing fixed kernel sizes. Our proposed M-CR U -Net outperforms alternative architectures across a range of metrics, demonstrating improved training efficiency and delivering enhanced quantitative and qualitative segmentation outcomes. These achievements underscore its proficiency in accurately delineating the intricate features within input images. The M-CR U-Net excels in capturing the fine details while minimizing overlapping regions, as evidenced by its dice coefficient of 0.978, intersection over union of 0.871, precision of 0.989, and recall of 0.982. Additionally, the OVCS algorithm enables effective segmentation of individual oil palm trees within highly crowded regions. By adeptly separating the boundaries of overlapped areas, this algorithm enhances segmentation results. This innovation ensures precise identification of the health and boundaries of oil palm trees, leading to an average performance improvement of 2.716% across various evaluation metrics. The proposed methodology demonstrates significant potential in detecting early symptoms of BSR disease in oil palm trees, even within crowded regions, leading to more effective management of oil palm plantations.
更多查看译文
关键词
Deep learning,Segmentation,U-Net,Image processing,Basal stem rot,UAV image
AI 理解论文
溯源树
样例
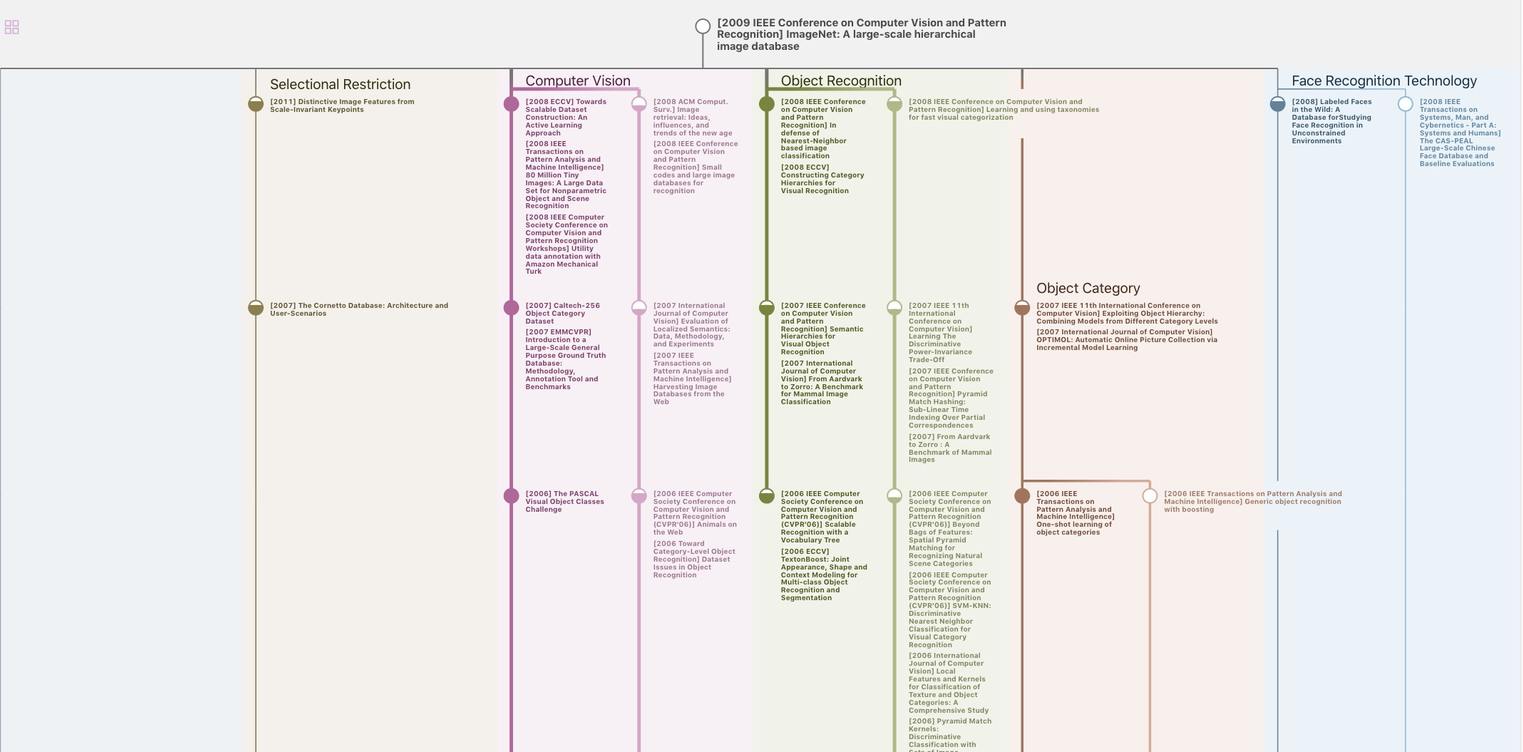
生成溯源树,研究论文发展脉络
Chat Paper
正在生成论文摘要