A data-driven decision support framework for DEA target setting: an explainable AI approach
ENGINEERING APPLICATIONS OF ARTIFICIAL INTELLIGENCE(2024)
摘要
The intention of target setting for Decision-Making Units (DMUs) in Data Envelopment Analysis (DEA) is to perform better than their peers or reach a reference efficiency level. However, most of the time, the logic behind the target setting is based on mathematical models, which are not achievable in practice. Besides, these models are based on decreasing/increasing inputs/outputs that might not be feasible based on DMU's potential in the real world. We propose a data-driven decision support framework to set actionable and feasible targets based on vital inputs-outputs for target setting. To do so, DMUs are classified in their corresponding Efficiency Frontier (EF) levels based on multiple EFs approach and a machine learning classifier. Then, the vital inputs-outputs are determined using an Explainable Artificial Intelligence (XAI) method. Finally, a Multi-Objective Counterfactual Explanation is developed based on DEA (MOCE-DEA) to lead DMU in reaching the reference EF by adjusting actionable and feasible inputs-outputs. We studied Iranian hospitals to evaluate the proposed framework and presented two cases to demonstrate its mechanism. The results show that the performance of the DMUs is improved to reach the reference EF for studied cases. Then, a validation was conducted with the primal DEA model to show the robust improvement of DMUs after adjusting their original value based on the generated solutions by the proposed framework. It demonstrates that the adjusted values can also improve DMUs' performance in the primal DEA model.
更多查看译文
关键词
Data envelopment analysis,Benchmarking,Target setting,Explainable artificial intelligence,LIME,Multi -objective counterfactual explanation
AI 理解论文
溯源树
样例
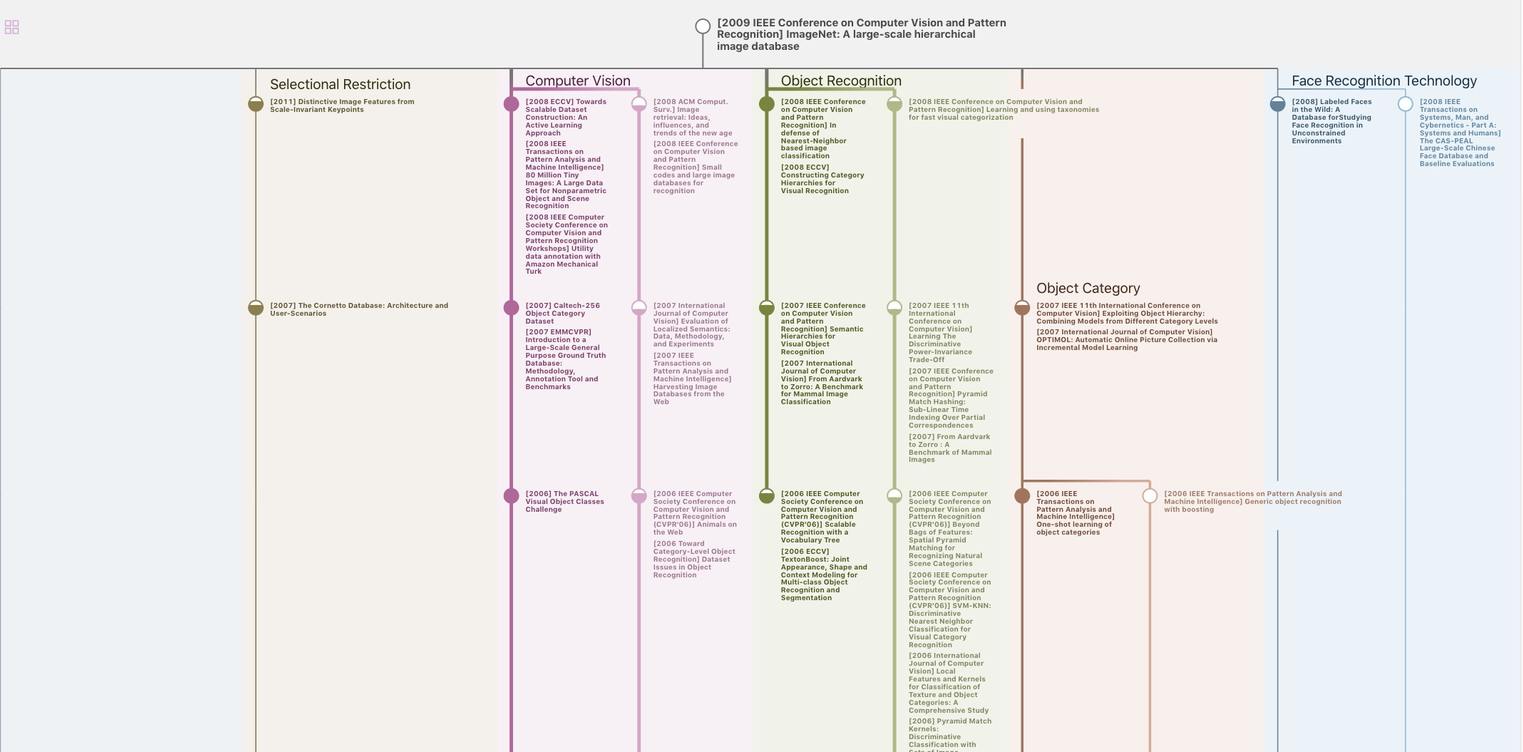
生成溯源树,研究论文发展脉络
Chat Paper
正在生成论文摘要