Four-channel generative adversarial networks can predict the distribution of reef-associated fish in the South and East China Seas
Ecological Informatics(2023)
摘要
Reef-associated fish (RAF) are socioeconomically and ecologically important. Herein, we used a four-channel generative adversarial network (GAN) architecture with a retrospective cycle feature to model the distribution of RAF in the East China Sea (ECS) and South China Sea (SCS). Our approach entailed the use of RAF suitability maps and key environmental layers, including sea body temperature, chlorophyll-α concentration, and salinity, as input data. These environmental factors have been demonstrated to significantly influence the distribution of RAF. Our results show that four-channel GANs performed better than one-channel GANs in predicting the distribution of RAF. Furthermore, the proposed four-channel retrospective-cycle GAN (4CRCGAN) model outperformed the commonly used four-channel convolutional GAN (4CCGAN), emphasizing the importance of considering temporal dynamics and feedback loops in predicting the distribution of species. However, it is worth noting that the predictive performance for the distribution of RAF in summer and winter was relatively poor, implying that other environmental and biological factors also exert nonnegligible influences on fish movement and abundance; this underscores the necessity of comprehensively understanding the intricate and dynamic nature of species-environment interactions within marine ecosystems. Our study also revealed that the proposed 4CRCGAN model performed well over multiple steps, which is reflective of its potential to provide valuable insights for decision-makers and stakeholders in the fisheries management sector. Overall, our findings offer critical insights for the development of effective and sustainable management strategies that balance the conservation and sustainable use of these valuable marine resources.
更多查看译文
关键词
Generative adversarial network, Reef -associated fish, East China Sea, South China Sea, Suitable habitats, Maxent
AI 理解论文
溯源树
样例
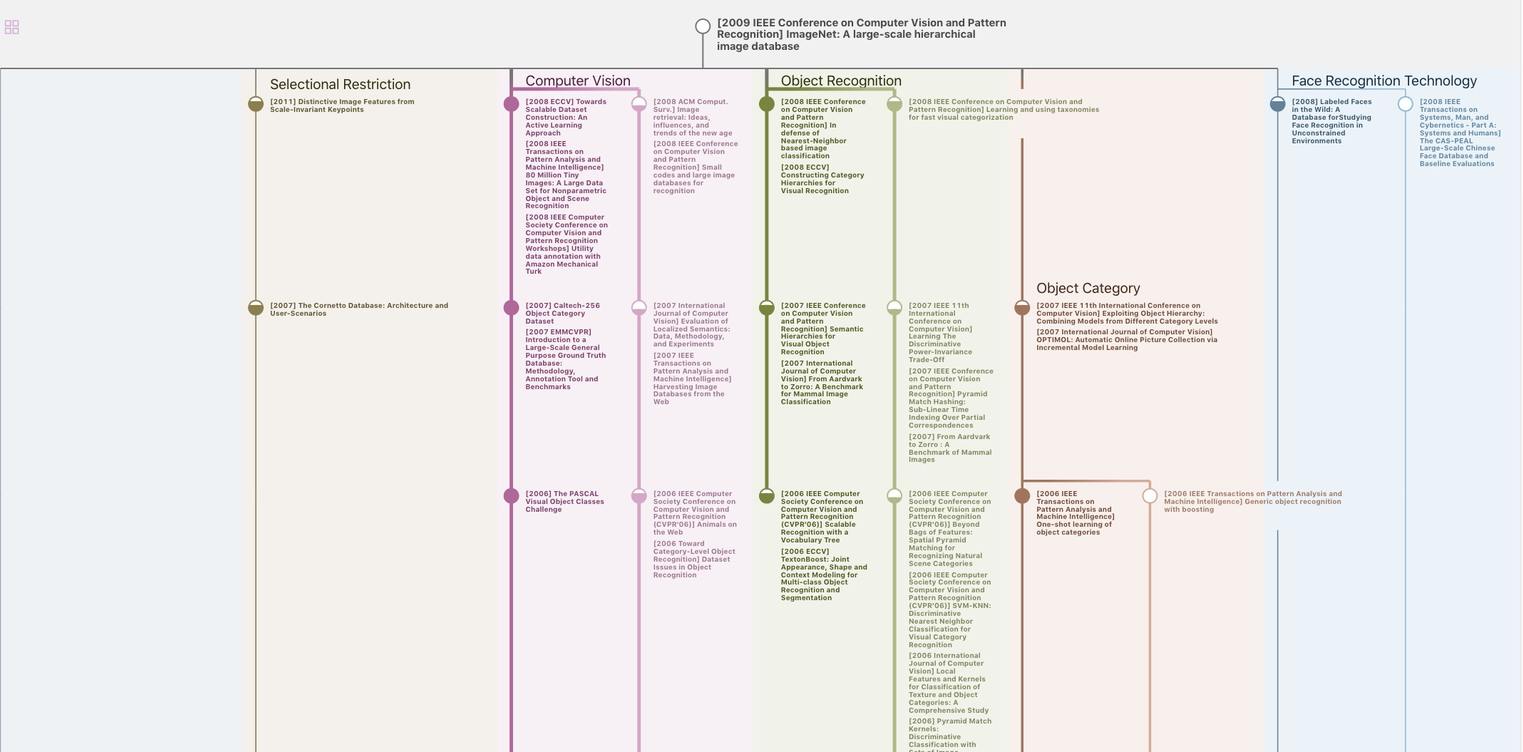
生成溯源树,研究论文发展脉络
Chat Paper
正在生成论文摘要