Periodically Exchange Teacher-Student for Source-Free Object Detection
Proceedings of the IEEE/CVF International Conference on Computer Vision (ICCV)(2023)
摘要
Source-free object detection (SFOD) aims to adapt the source detector to unlabeled target domain data in the absence of source domain data. Most SFOD methods follow the same self-training paradigm using mean-teacher (MT) framework where the student model is guided by only one single teacher model. However, such paradigm can easily fall into a training instability problem that when the teacher model collapses uncontrollably due to the domain shift, the student model also suffers drastic performance degradation. To address this issue, we propose the Periodically Exchange Teacher-Student (PETS) method, a simple yet novel approach that introduces a multiple-teacher framework consisting of a static teacher, a dynamic teacher, and a student model. During the training phase, we periodically exchange the weights between the static teacher and the student model. Then, we update the dynamic teacher using the moving average of the student model that has already been exchanged by the static teacher. In this way, the dynamic teacher can integrate knowledge from past periods, effectively reducing error accumulation and enabling a more stable training process within the MT-based framework. Further, we develop a consensus mechanism to merge the predictions of two teacher models to provide higher-quality pseudo labels for student model. Extensive experiments on multiple SFOD benchmarks show that the proposed method achieves state-of-the-art performance compared with other related methods, demonstrating the effectiveness and superiority of our method on SFOD task.
更多查看译文
关键词
Transfer Learning,Semi-Supervised Learning,Object Detection,Few-Shot Learning,Cross-Modal Retrieval
AI 理解论文
溯源树
样例
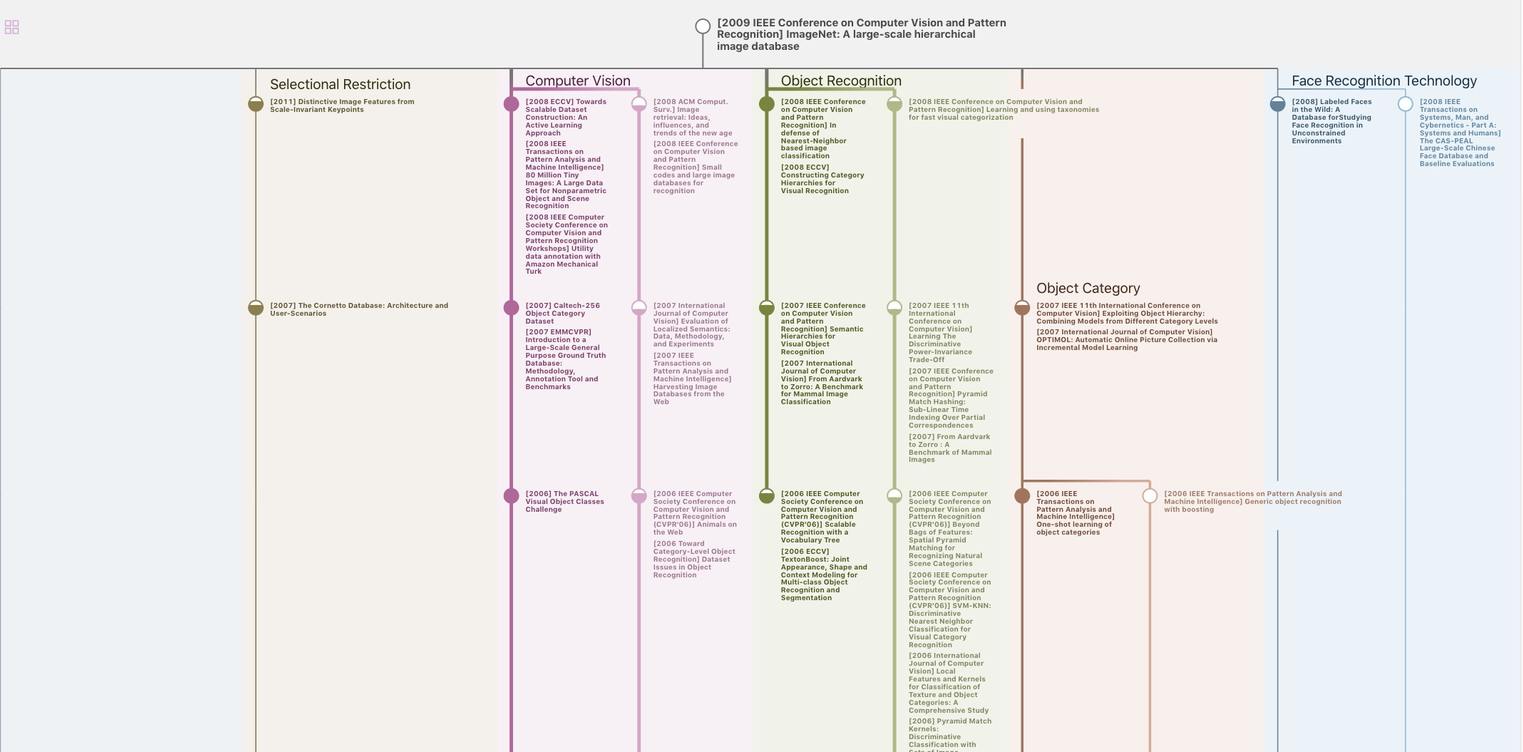
生成溯源树,研究论文发展脉络
Chat Paper
正在生成论文摘要