Image processing and machine learning approach for yolk color evaluation
THAI JOURNAL OF VETERINARY MEDICINE(2023)
摘要
The objective of this study was to develop a predictive model for yolk color measurement using machine learning. A total of eight hundred eggs were randomly collected from thirty laying hens (Lohmann brown, 28-35 weeks). The experimental diets were formulated based on broken rice or corn and further supplemented with canthaxanthin from 10 to 150 mg/kg. The Digital YolkFanTM was used to classify yolk color scales from 0 to 15, whereas the Hunter Lab was used to measure color values. Furthermore, yolk images were obtained from a digital camera and then extracted into red color, green color and blue (RGB) and hue, saturation and value (HSV). Machine learning, including multiple linear regression, decision tree (DT), support vector machine, artificial neural networks and deep learning were used to develop the predictive models. The accuracy of R2 was greater for the HSV (0.971) than for the Hunter Lab (0.969) and RGB (0.947) approaches. The root mean square error (RMSE) was also lower for HSV than for Hunter Lab and RGB (0.770, 0.805 and 1.055, respectively). Further improvement based on HSV with DT achieved the highest accuracy (R2 = 0.996) and the lowest statistical error measurements (RMSE = 0.288). In conclusion, the HSV obtained from the digital yolk image provided a suitable color system, with the use of the DT model expected to improve the accuracy of prediction. Therefore, combined digital imagery and machine learning provided a rapid and highly cost-effective technique requiring little human subjectivity for yolk color evaluation.
更多查看译文
关键词
Decision tree,digital image,laying hen,machine learning,yolk color
AI 理解论文
溯源树
样例
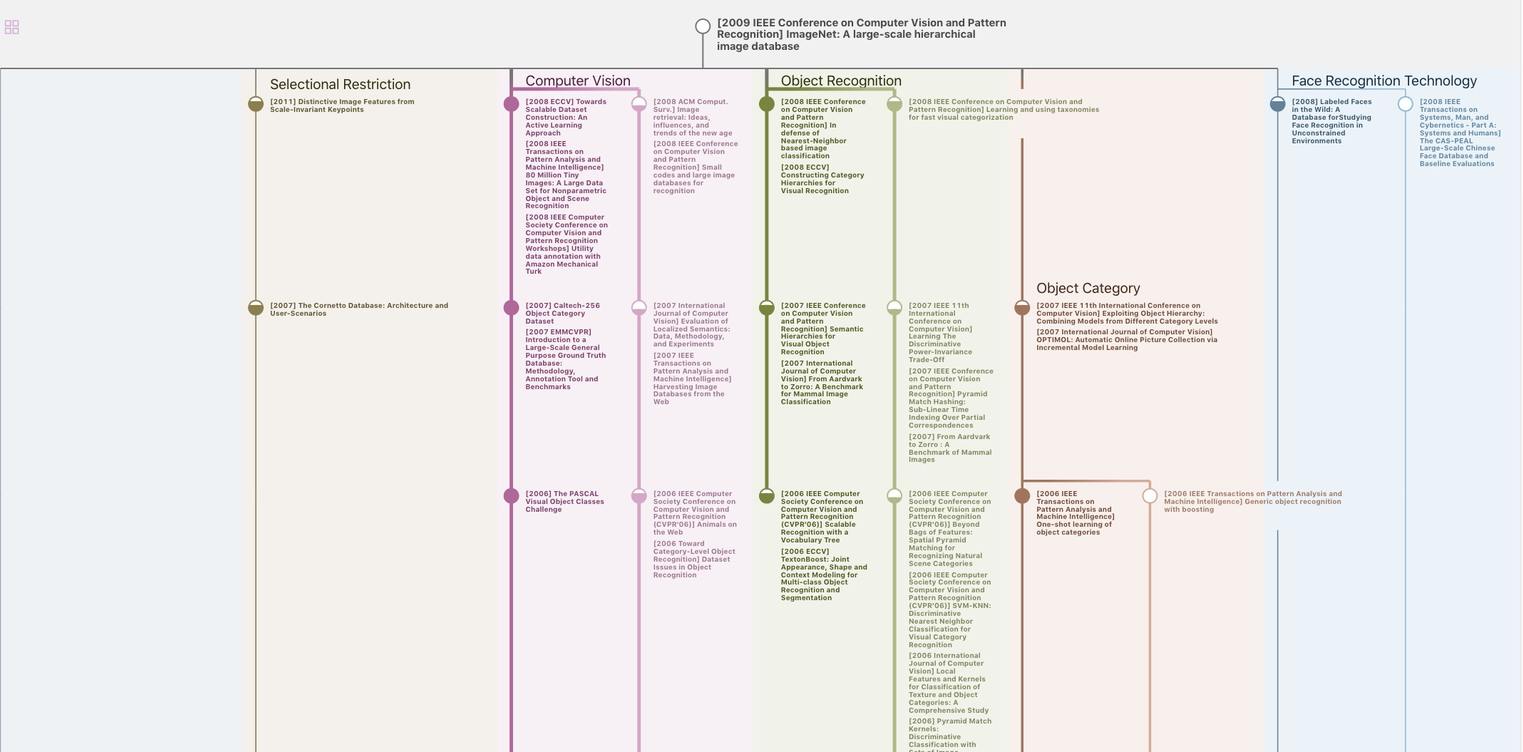
生成溯源树,研究论文发展脉络
Chat Paper
正在生成论文摘要