A novel method for fast forward simulation of ground penetrating radar and buried target detection based on machine learning
CHINESE JOURNAL OF GEOPHYSICS-CHINESE EDITION(2023)
摘要
Ground penetrating radar forward simulation plays an important role in real radar data interpretation and full waveform inversion. A near real-time GPR forward simulation method based on machine learning framework is proposed for the problems of huge computation, time-consuming and unfavorable to timely detection in traditional ground-penetrating radar forward simulation. Using the detection of rebar in concrete as a GPR application scenario, the water content of concrete, the radius and the burial depth of the rebar as model parameters, and numerically simulating the scattered echo signal using FDTD. Principal component analysis is applied to reduce the dimensionality of the echo data to obtain the corresponding principal component weight coefficients, which are used as the output of the machine learning network. A multilayer recurrent network architecture and learning strategy based on random forest is designed, which not only fully explores the intrinsic causal relationship between learning model parameters and principal component weight coefficients, but also shares the interconnection between principal components, and has the function of refining and correcting for each predicted principal component, so as to realize the fast forward simulation of ground-penetrating radar based on machine learning, which effectively improves the accuracy of forward simulation compared with traditional machine learning. On this basis two deep neural networks are combined with random forest to establish a machine learning-based scene parameter prediction model with the principal component weight coefficients of echo data as input, which achieves near real-time buried target detection with a maximum error of 2% for the predicted concrete water content and 6.7% for the maximum error of the buried depth of the rebar.
更多查看译文
关键词
Ground-penetrating radar,Fast forward simulation,Machine learning (ML),Random forest (RF),Principal component analysis (PCA),Buried target detection
AI 理解论文
溯源树
样例
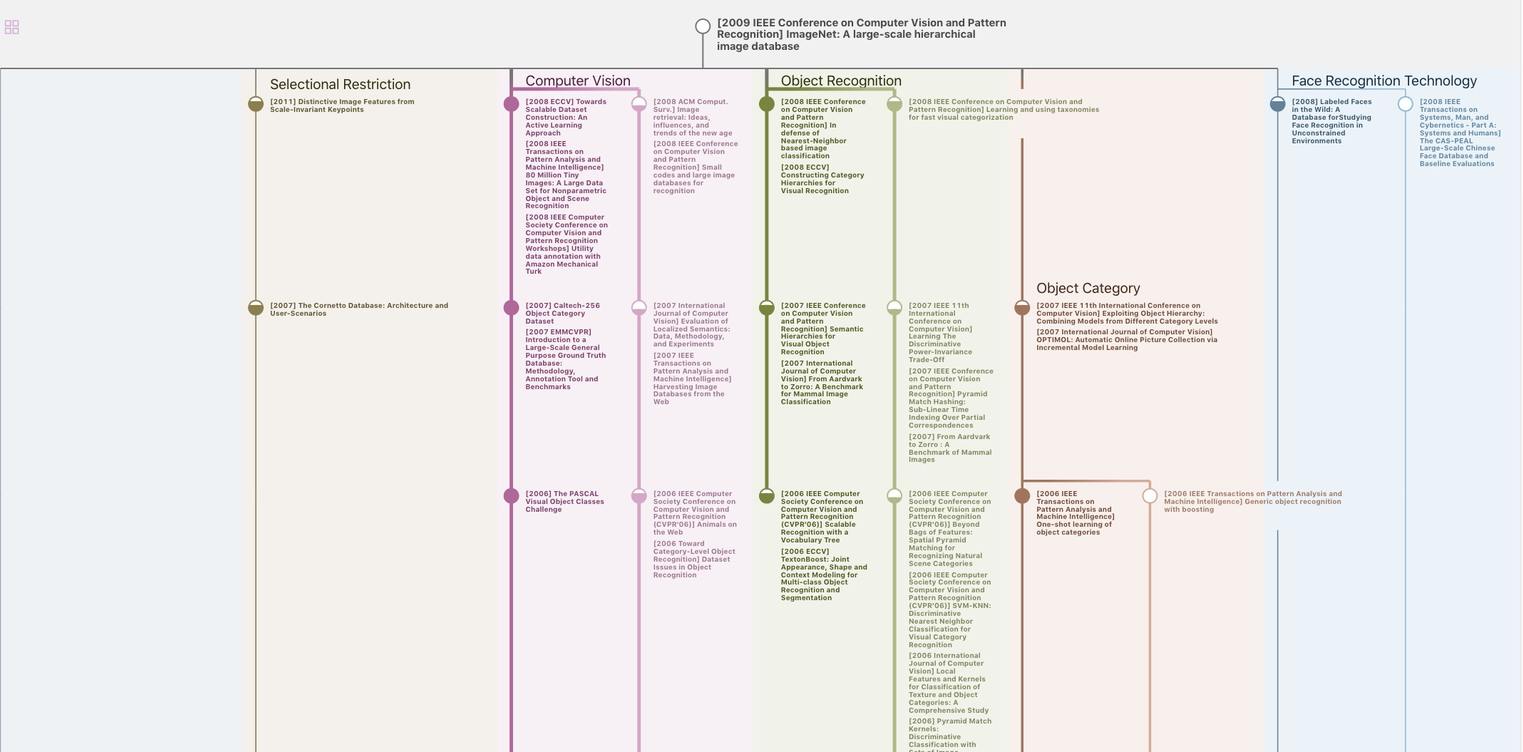
生成溯源树,研究论文发展脉络
Chat Paper
正在生成论文摘要