GastroNet: Gastrointestinal Polyp and Abnormal Feature Detection and Classification with Deep Learning Approach
IEEE access(2023)
摘要
The early detection of digestive problems is essential for lowering the chance of acquiring any form of gastrointestinal cancer, including esophageal cancer. Endoscopy is the method that is used the majority of the time for the purpose of examining and taking photos of this sort of illness. The application of artificial intelligence is now proving to be very efficient in enhancing the identification of gastrointestinal polyps and other abnormal features located inside the gastrointestinal system. As a direct consequence of this development, the use of AI within this sector has seen substantial growth. In the framework of artificial intelligence, this research investigates how well various types of algorithms perform in terms of polyp and abnormal feature recognition accuracy, efficiency, and detection. And introduced a model in this work that is GastroNet. It is developed by doing hyperparameter fine-tuning on YOLOv5 in order to find specific polyps and abnormal characteristics, particularly esophagitis. In this method, a single neural network is used to do an analysis on the whole picture before it is disassembled into its component parts and the bounding boxes and probabilities for each one are calculated independently. The goal of the hyperparameter fine-tuning is to further enhance the overall optimization of the model. Two different methods of annotation were used on a collection of data that consisted of one thousand separate images that needed to be labelled. In addition to implementing the fine-tuned SSD model, this study used three distinct backbone networks: MobileNet v2, MobileNet v2 FPN Lite, and Resnet50 v1 FPN. Additionally, this study has used CSPdarknet53 to create the improved YOLOv4 model. The results of the studies demonstrate that the proposed model, GastroNet was effective in correctly recognizing polyps and aberrant characteristics, reaching a high mAP (mean Average Precision), F1 score, and precision with a value of 0.99 and recall with a value of 1.00. The findings of this research will be a great help to physicians in the proper identification and diagnosis of abnormal features and gastrointestinal polyps.
更多查看译文
关键词
Artificial intelligence,detection and classification,GastroNet,machine learning,health care,gastrointestinal malignancies
AI 理解论文
溯源树
样例
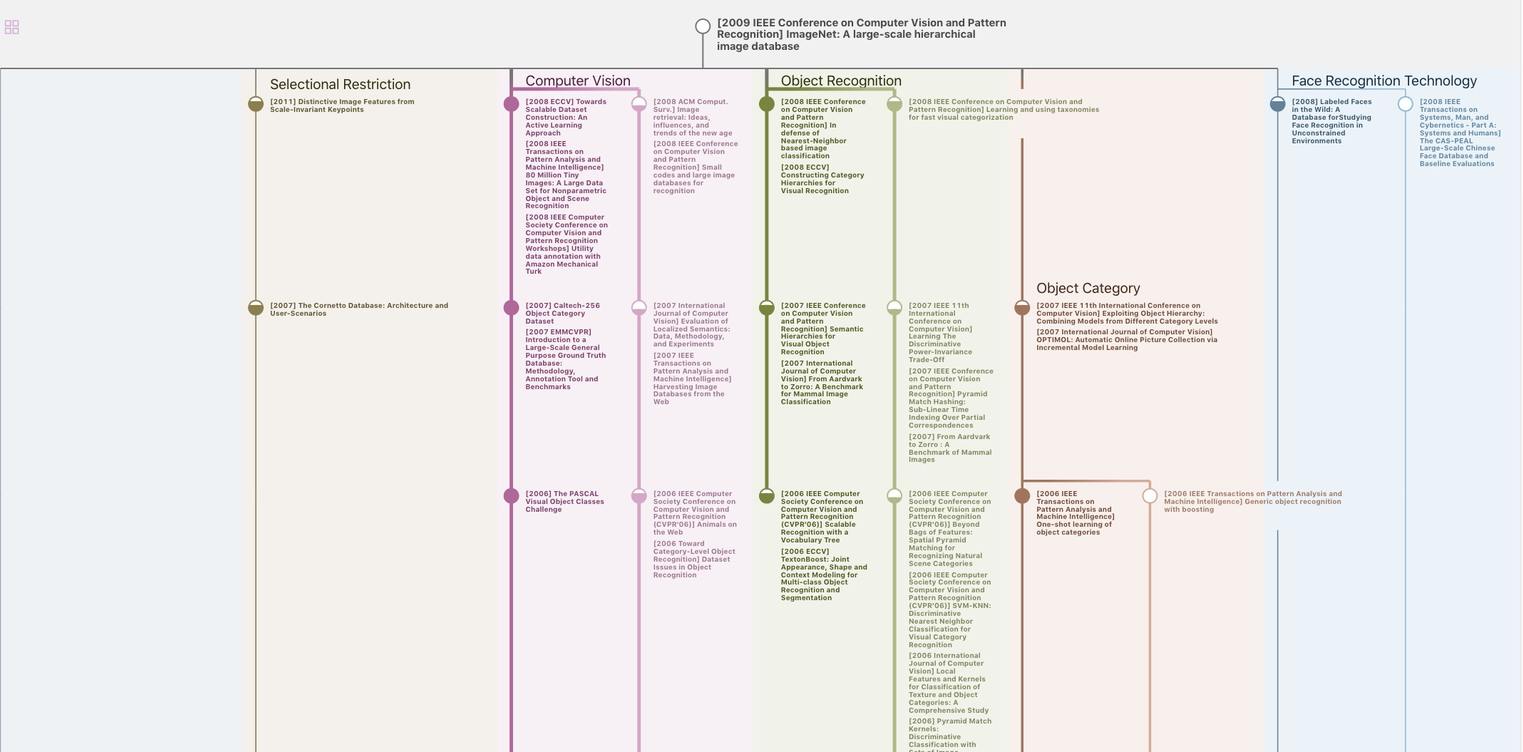
生成溯源树,研究论文发展脉络
Chat Paper
正在生成论文摘要