Deep Learning for Low-Frequency Extrapolation and Seismic Acoustic Impedance Inversion.
IEEE Trans. Geosci. Remote. Sens.(2023)
摘要
Seismic inversion can be used to invert the subsurface acoustic impedance leveraging migrated seismic section, which can help lithology interpretation. It is not easy to predict impedance directly from poststack seismic data. In the field data, the interference of random noise aggravates the difficulty of impedance inversion. Previous work mainly focused on trace-by-trace strategy leading to poor lateral continuity. We propose a 2-D temporal convolutional network (TCN)-based poststack seismic low-frequency extrapolation and a TCN-based impedance prediction method. We use a two-step workflow for acoustic impedance prediction from poststack seismic data. First, we use a neural network (low-frequency extrapolation network, LE-Net) for the low-frequency extrapolation of seismic data, and then we use another neural network (acoustic impedance network, AI-Net) to predict acoustic impedance. The input to LE-Net is high-frequency band-limited seismic and low-frequency impedance data. Seismic data after low-frequency extrapolation and low-frequency impedance are used to predict impedance. Two-dimensional TCN and multitrace input data can introduce spatial information from surrounding traces. The output of the network is single-trace data. The proposed network can ensure the single-trace prediction accuracy and improve lateral continuity. Numerical experimental results show that our proposed two-step workflow, named AI-LE, performs well on Marmousi II and has a certain generalization on the SEAM model. The results on field data show that AI-Net can predict relatively accurate impedance. The low-frequency extrapolation of seismic data can help improve the performance of impedance prediction.
更多查看译文
关键词
Deep learning,impedance inversion,low-frequency extrapolation,seismic inversion
AI 理解论文
溯源树
样例
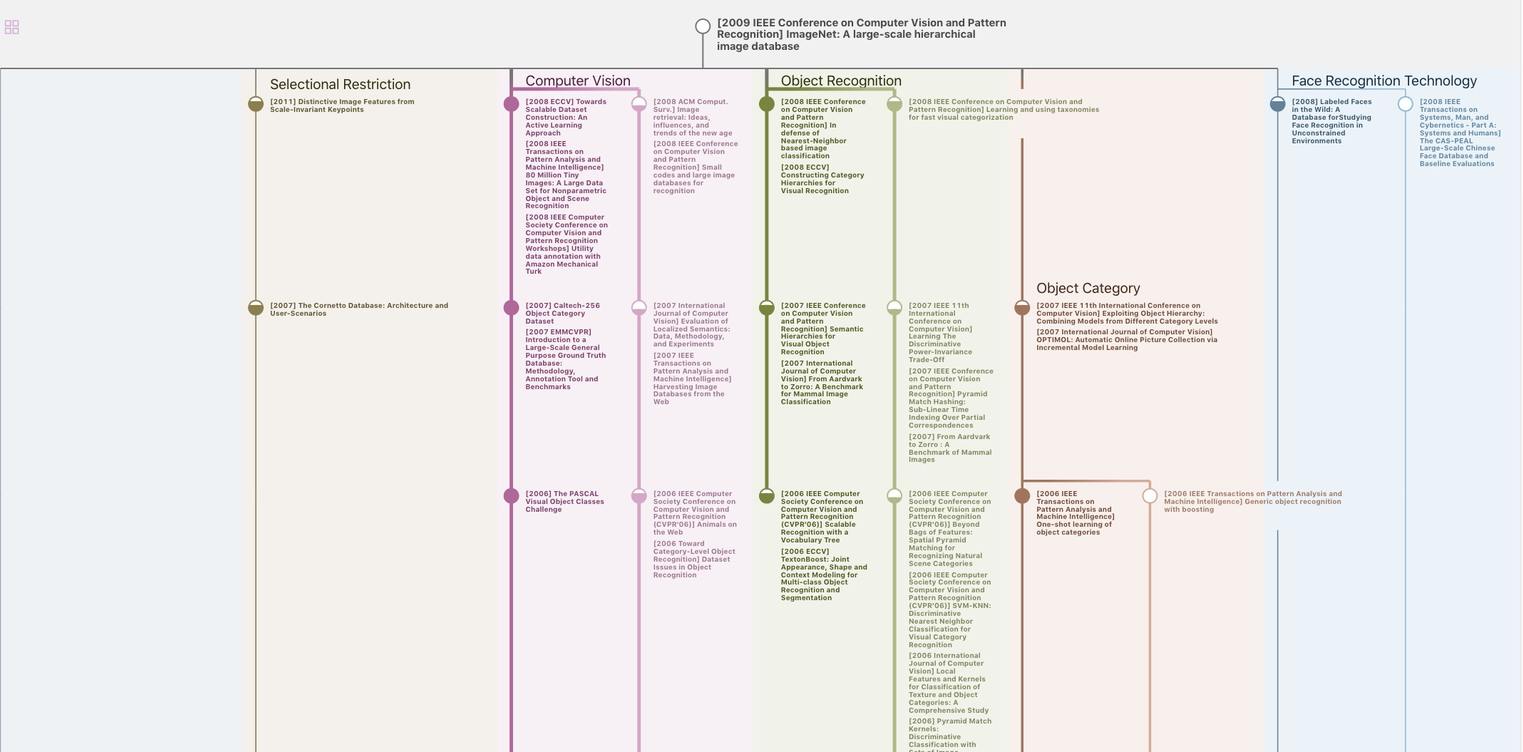
生成溯源树,研究论文发展脉络
Chat Paper
正在生成论文摘要