Deep Reinforcement Learning for Improving Resource Utilization Efficiency of URLLC With Imperfect Channel State Information
IEEE Wireless Communications Letters(2023)
摘要
This letter optimizes resource blocks allocated for channel estimation and data transmission in multiple-input and single-output (MISO) ultra-reliable low-latency communication (URLLC) systems. The goal is to improve the resource utilization efficiency subject to a reliability constraint. Considering that wireless channels are correlated in the temporal domain, and the channel estimation is not error-free, the problem is formulated as a partial observation Markov decision process (POMDP), which is a sequential decision-making problem with partial observations. To solve this problem, we develop a constrained deep reinforcement learning (DRL) algorithm, namely Cascaded-Action Twin Delayed Deep Deterministic policy (CA-TD3). We train the policy by using a primal domain method and compare it with a primal-dual method and an existing benchmark. Two channel models are considered for evaluation: the first-order autoregressive correlated channel model and the clustered delay line (CDL) channel model. The simulation results show that the proposed primal CA-TD3 method converges faster than the primal-dual method and achieves over 30% improvement in resource utilization efficiency compared to the benchmark.
更多查看译文
关键词
Ultra-reliable and low-latency communications,deep reinforcement learning,resource allocation
AI 理解论文
溯源树
样例
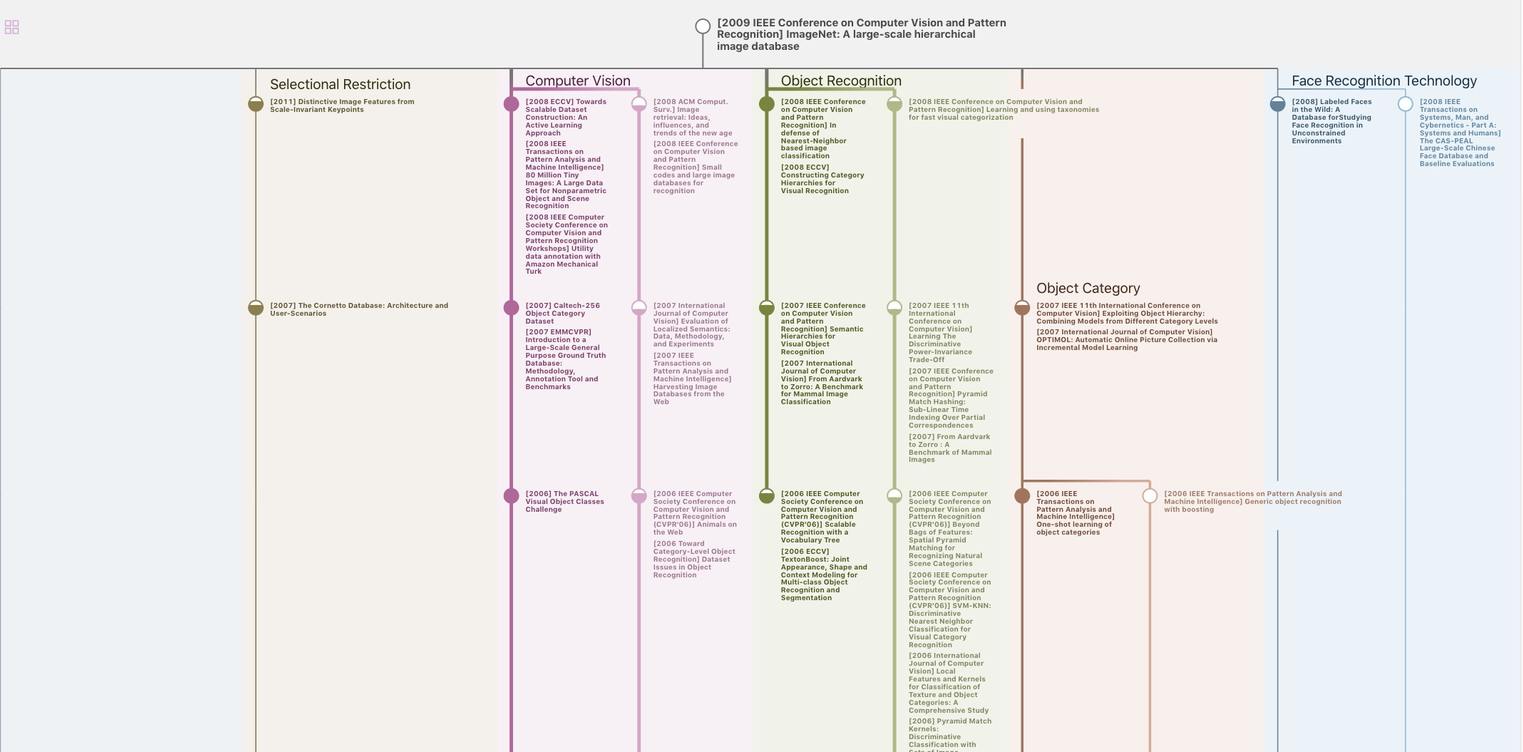
生成溯源树,研究论文发展脉络
Chat Paper
正在生成论文摘要