Something for (almost) nothing: Improving deep ensemble calibration using unlabeled data
CoRR(2023)
摘要
We present a method to improve the calibration of deep ensembles in the small training data regime in the presence of unlabeled data. Our approach is extremely simple to implement: given an unlabeled set, for each unlabeled data point, we simply fit a different randomly selected label with each ensemble member. We provide a theoretical analysis based on a PAC-Bayes bound which guarantees that if we fit such a labeling on unlabeled data, and the true labels on the training data, we obtain low negative log-likelihood and high ensemble diversity on testing samples. Empirically, through detailed experiments, we find that for low to moderately-sized training sets, our ensembles are more diverse and provide better calibration than standard ensembles, sometimes significantly.
更多查看译文
关键词
deep ensemble calibration
AI 理解论文
溯源树
样例
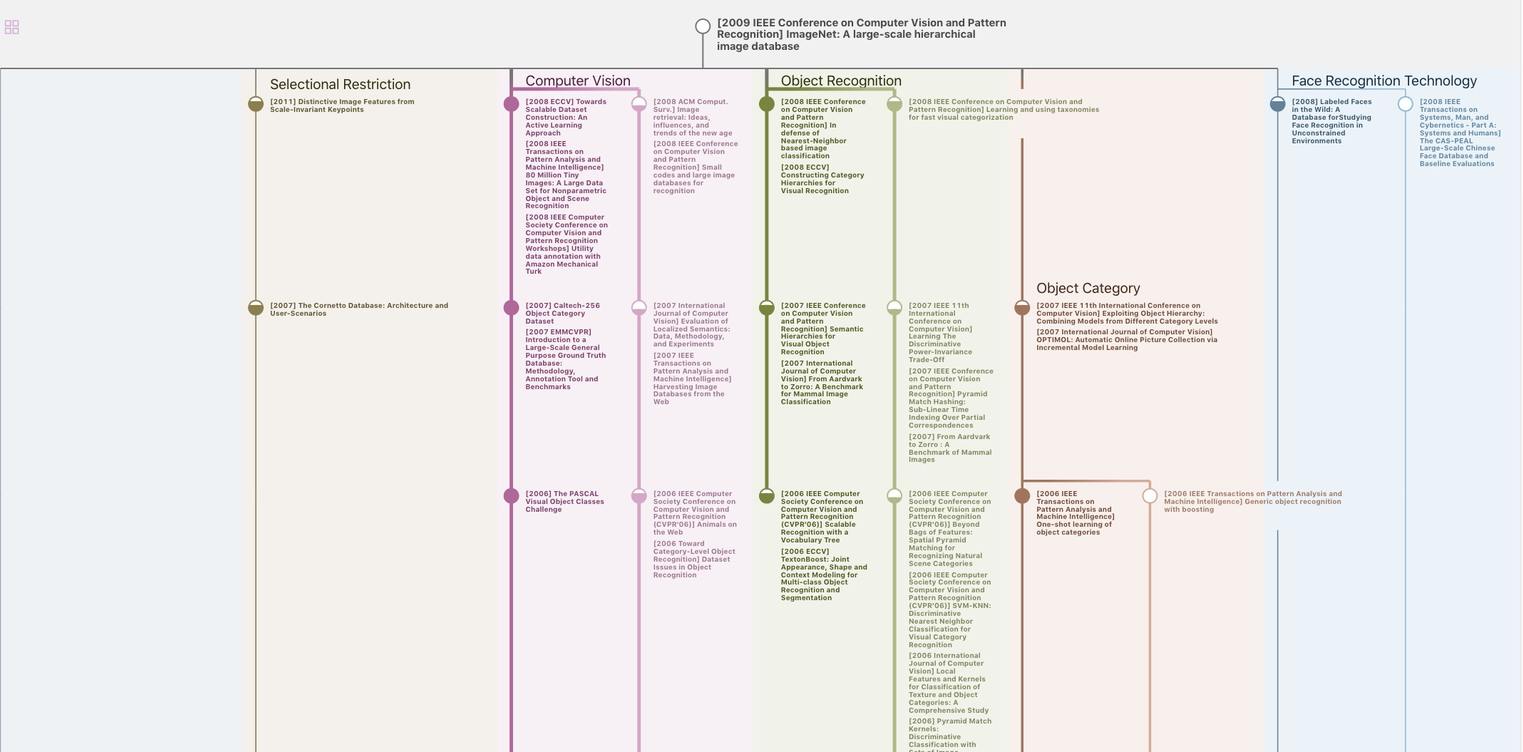
生成溯源树,研究论文发展脉络
Chat Paper
正在生成论文摘要